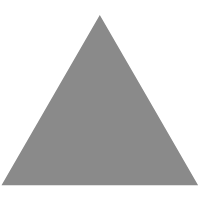
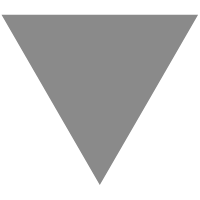
用GPU来运行Python代码
source link: https://www.pkslow.com/archives/run-python-with-gpu
Go to the source link to view the article. You can view the picture content, updated content and better typesetting reading experience. If the link is broken, please click the button below to view the snapshot at that time.
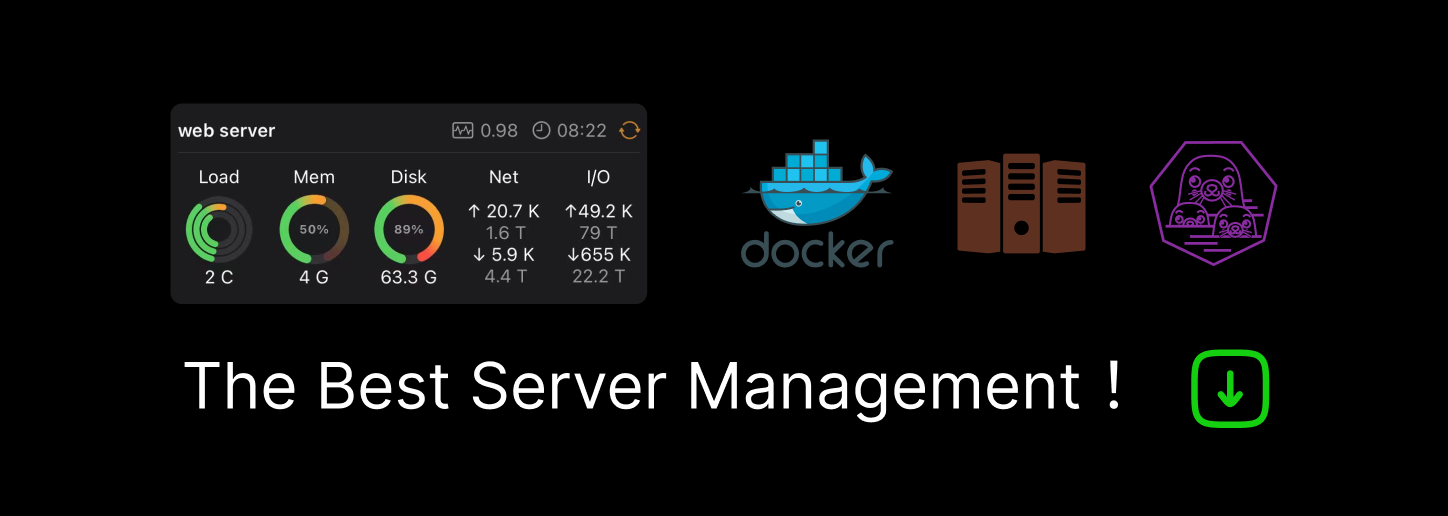
南瓜慢说官方网站 pkslow.com
敢一行,则予知。脚踏实地,知行合一。
前几天捣鼓了一下Ubuntu,正是想用一下我旧电脑上的N卡,可以用GPU来跑代码,体验一下多核的快乐。
还好我这破电脑也是支持Cuda的:
$ sudo lshw -C display
*-display
description: 3D controller
product: GK208M [GeForce GT 740M]
vendor: NVIDIA Corporation
physical id: 0
bus info: pci@0000:01:00.0
version: a1
width: 64 bits
clock: 33MHz
capabilities: pm msi pciexpress bus_master cap_list rom
configuration: driver=nouveau latency=0
resources: irq:35 memory:f0000000-f0ffffff memory:c0000000-cfffffff memory:d0000000-d1ffffff ioport:6000(size=128)
安装相关工具
首先安装一下Cuda的开发工具,命令如下:
$ sudo apt install nvidia-cuda-toolkit
查看一下相关信息:
$ nvcc --version
nvcc: NVIDIA (R) Cuda compiler driver
Copyright (c) 2005-2021 NVIDIA Corporation
Built on Thu_Nov_18_09:45:30_PST_2021
Cuda compilation tools, release 11.5, V11.5.119
Build cuda_11.5.r11.5/compiler.30672275_0
通过Conda安装相关的依赖包:
conda install numba & conda install cudatoolkit
通过pip安装也可以,一样的。
测试与驱动安装
简单测试了一下,发觉报错了:
$ /home/larry/anaconda3/bin/python /home/larry/code/pkslow-samples/python/src/main/python/cuda/test1.py
Traceback (most recent call last):
File "/home/larry/anaconda3/lib/python3.9/site-packages/numba/cuda/cudadrv/driver.py", line 246, in ensure_initialized
self.cuInit(0)
File "/home/larry/anaconda3/lib/python3.9/site-packages/numba/cuda/cudadrv/driver.py", line 319, in safe_cuda_api_call
self._check_ctypes_error(fname, retcode)
File "/home/larry/anaconda3/lib/python3.9/site-packages/numba/cuda/cudadrv/driver.py", line 387, in _check_ctypes_error
raise CudaAPIError(retcode, msg)
numba.cuda.cudadrv.driver.CudaAPIError: [100] Call to cuInit results in CUDA_ERROR_NO_DEVICE
During handling of the above exception, another exception occurred:
Traceback (most recent call last):
File "/home/larry/code/pkslow-samples/python/src/main/python/cuda/test1.py", line 15, in <module>
gpu_print[1, 2]()
File "/home/larry/anaconda3/lib/python3.9/site-packages/numba/cuda/compiler.py", line 862, in __getitem__
return self.configure(*args)
File "/home/larry/anaconda3/lib/python3.9/site-packages/numba/cuda/compiler.py", line 857, in configure
return _KernelConfiguration(self, griddim, blockdim, stream, sharedmem)
File "/home/larry/anaconda3/lib/python3.9/site-packages/numba/cuda/compiler.py", line 718, in __init__
ctx = get_context()
File "/home/larry/anaconda3/lib/python3.9/site-packages/numba/cuda/cudadrv/devices.py", line 220, in get_context
return _runtime.get_or_create_context(devnum)
File "/home/larry/anaconda3/lib/python3.9/site-packages/numba/cuda/cudadrv/devices.py", line 138, in get_or_create_context
return self._get_or_create_context_uncached(devnum)
File "/home/larry/anaconda3/lib/python3.9/site-packages/numba/cuda/cudadrv/devices.py", line 153, in _get_or_create_context_uncached
with driver.get_active_context() as ac:
File "/home/larry/anaconda3/lib/python3.9/site-packages/numba/cuda/cudadrv/driver.py", line 487, in __enter__
driver.cuCtxGetCurrent(byref(hctx))
File "/home/larry/anaconda3/lib/python3.9/site-packages/numba/cuda/cudadrv/driver.py", line 284, in __getattr__
self.ensure_initialized()
File "/home/larry/anaconda3/lib/python3.9/site-packages/numba/cuda/cudadrv/driver.py", line 250, in ensure_initialized
raise CudaSupportError(f"Error at driver init: {description}")
numba.cuda.cudadrv.error.CudaSupportError: Error at driver init: Call to cuInit results in CUDA_ERROR_NO_DEVICE (100)
网上搜了一下,发现是驱动问题。通过Ubuntu自带的工具安装显卡驱动:
还是失败:
$ nvidia-smi
NVIDIA-SMI has failed because it couldn't communicate with the NVIDIA driver. Make sure that the latest NVIDIA driver is installed and running.
最后,通过命令行安装驱动,成功解决这个问题:
$ sudo apt install nvidia-driver-470
检查后发现正常了:
$ nvidia-smi
Wed Dec 7 22:13:49 2022
+-----------------------------------------------------------------------------+
| NVIDIA-SMI 470.161.03 Driver Version: 470.161.03 CUDA Version: 11.4 |
|-------------------------------+----------------------+----------------------+
| GPU Name Persistence-M| Bus-Id Disp.A | Volatile Uncorr. ECC |
| Fan Temp Perf Pwr:Usage/Cap| Memory-Usage | GPU-Util Compute M. |
| | | MIG M. |
|===============================+======================+======================|
| 0 NVIDIA GeForce ... Off | 00000000:01:00.0 N/A | N/A |
| N/A 51C P8 N/A / N/A | 4MiB / 2004MiB | N/A Default |
| | | N/A |
+-------------------------------+----------------------+----------------------+
+-----------------------------------------------------------------------------+
| Processes: |
| GPU GI CI PID Type Process name GPU Memory |
| ID ID Usage |
|=============================================================================|
| No running processes found |
+-----------------------------------------------------------------------------+
测试代码也可以跑了。
测试Python代码
准备以下代码:
from numba import cuda
import os
def cpu_print():
print('cpu print')
@cuda.jit
def gpu_print():
dataIndex = cuda.threadIdx.x + cuda.blockIdx.x * cuda.blockDim.x
print('gpu print ', cuda.threadIdx.x, cuda.blockIdx.x, cuda.blockDim.x, dataIndex)
if __name__ == '__main__':
gpu_print[4, 4]()
cuda.synchronize()
cpu_print()
这个代码主要有两个函数,一个是用CPU执行,一个是用GPU执行,执行打印操作。关键在于@cuda.jit
这个注解,让代码在GPU上执行。运行结果如下:
$ /home/larry/anaconda3/bin/python /home/larry/code/pkslow-samples/python/src/main/python/cuda/print_test.py
gpu print 0 3 4 12
gpu print 1 3 4 13
gpu print 2 3 4 14
gpu print 3 3 4 15
gpu print 0 2 4 8
gpu print 1 2 4 9
gpu print 2 2 4 10
gpu print 3 2 4 11
gpu print 0 1 4 4
gpu print 1 1 4 5
gpu print 2 1 4 6
gpu print 3 1 4 7
gpu print 0 0 4 0
gpu print 1 0 4 1
gpu print 2 0 4 2
gpu print 3 0 4 3
cpu print
可以看到GPU总共打印了16次,使用了不同的Thread来执行。这次每次打印的结果都可能不同,因为提交GPU是异步执行的,无法确保哪个单元先执行。同时也需要调用同步函数cuda.synchronize()
,确保GPU执行完再继续往下跑。
我们通过这个函数来看GPU并行的力量:
from numba import jit, cuda
import numpy as np
# to measure exec time
from timeit import default_timer as timer
# normal function to run on cpu
def func(a):
for i in range(10000000):
a[i] += 1
# function optimized to run on gpu
@jit(target_backend='cuda')
def func2(a):
for i in range(10000000):
a[i] += 1
if __name__ == "__main__":
n = 10000000
a = np.ones(n, dtype=np.float64)
start = timer()
func(a)
print("without GPU:", timer() - start)
start = timer()
func2(a)
print("with GPU:", timer() - start)
结果如下:
$ /home/larry/anaconda3/bin/python /home/larry/code/pkslow-samples/python/src/main/python/cuda/time_test.py
without GPU: 3.7136273959999926
with GPU: 0.4040513340000871
可以看到使用CPU需要3.7秒,而GPU则只要0.4秒,还是能快不少的。当然这里不是说GPU一定比CPU快,具体要看任务的类型。
代码请看GitHub: https://github.com/LarryDpk/pkslow-samples/tree/master/python
References:
https://www.geeksforgeeks.org/running-python-script-on-gpu/
https://zhuanlan.zhihu.com/p/76297133
https://zhuanlan.zhihu.com/p/77307505
Code for all: GitHub
欢迎关注微信公众号<南瓜慢说>,将持续为你更新...
Recommendations:
Cloud Native
Terraform
Container: Docker/Kubernetes
Spring Boot / Spring Cloud
Https
如何制定切实可行的计划并好好执行
Recommend
-
78
其实,不只是iPad,手机也可以。 痛点 我组织过几次线下编程工作坊,带着同学们用Python处理数据科学问题。 其中最让人头疼的,就是运行环境的安装。 实事求是地讲,参加工作坊之前,我已经做了认真准备。 例如集成环境,选用了对用户很友好的Anaconda。
-
35
-
24
分享创造 - @Keller0 - 做了一个运行短代码的网站.目前支持的语言 Bash, C, C++, Go, Haskell, Java, Perl, PHP, Python, Ruby, Rust.前端用 Vuej
-
28
前端日常开发中,我们使用喜爱的 IDE 调试 JavaScript 代码,比如我喜欢的代码编辑器有两个, Sublime Text 3 和 VS Code ,前几年还使用过 Atom ,偶尔我们会遇到临时...
-
10
昨天 Ian Lance Taylor 和 Robert Griesemer 发布了Go泛型的新的草案( The Next Step for Generics ), 国内外的Gopher反响非常的热烈,大家纷纷对草案和这个文章进行了解读,...
-
8
python中代码运行的困惑 网上看到的代码: def clear_list(l):ll = [1,2,3]clear_list(ll)print(l...
-
6
若干GPU代码优化摘要因为最近一直在研究优化CUDA代码,使深度学习模型运行速度变快,读了不少NVIDIA博客的文章,其中有四篇印象比较深刻,实用度也比较高,特此总结一下。Optimize for Pointer Aliasing
-
3
南瓜慢说 www.pkslow.com 随笔 - 137, 文章 - 0, 评论 - 42, 阅读 - 21万
-
7
使用 Golang 和 Docker 运行 Python 代码2023年05月21日
-
6
Python 多进程使用单机多GPU加速推理发表于2024-01-17|更新于2024-01-17|technology
About Joyk
Aggregate valuable and interesting links.
Joyk means Joy of geeK