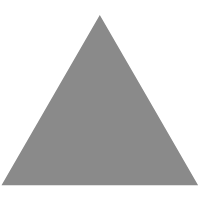
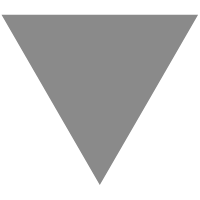
Train, Validation and Test Split for torchvision Datasets · GitHub
source link: https://gist.github.com/kevinzakka/d33bf8d6c7f06a9d8c76d97a7879f5cb
Go to the source link to view the article. You can view the picture content, updated content and better typesetting reading experience. If the link is broken, please click the button below to view the snapshot at that time.
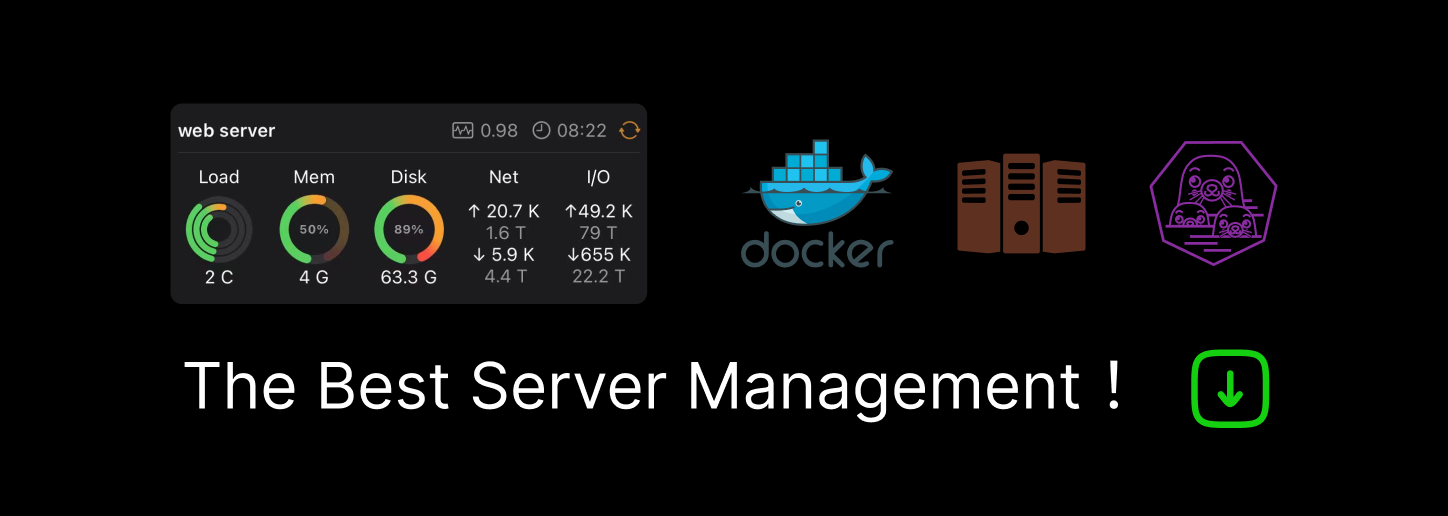
Why load the dataset twice into 'train_dataset' and 'valid_dataset'?
Author
kevinzakka commented on Oct 22, 2017
@ajwitty train and valid might not always have the same transformations
If using CUDA, num_workers should be set to 1
@kevinzakka Why?
I searched for discussions and documentation about the relationship between using GPUs and setting PyTorch's num_workers, but couldn't find any.
Also, thank you for writing this gist.
Hey, @kevinzakka can you please tell me how to use your script ? Should I copy paste it in my script or import it in my script? What are the modules of torch should I import ? I'm getting errors. Please help.
@krishnavishalv
import numpy as np
import torch
from torchvision import datasets, transforms
from torch.utils.data.sampler import SubsetRandomSampler
Hi @kevinzakka, so for the train_loader and test_loader, shuffle has to be False according to the Pytorch documentation on DataLoader. Does that mean in your way we have to sacrifice shuffling during training?
Hi, in my opinion, the normalize
should be optional, considering the mean/std in other datasets is not the same as yours (mean = [0.485, 0.456, 0.406], std = [0.229, 0.224, 0.225]
), though ideally mean/std would not be too different from it, not to mention that we still have batch norm.
Author
kevinzakka commented on Jan 25, 2018
@wanglouis49 it actually does not because we use SubsetRandomSampler
and according to the documentation: "Samples elements randomly from a given list of indices, without replacement."
Isn't it pointless to set a fixed random seed? It does help to generate the same order of indices for splitting the training set and validation set. But the SubsetRandomSampler does not use the seed, thus each batch sampled for training will be different every time.
Author
kevinzakka commented on May 3, 2018
Isn't it pointless to set a fixed random seed? It does help to generate the same order of indices for splitting the training set and validation set. But the SubsetRandomSampler does not use the seed, thus each batch sampled for training will be different every time.
@songkangsg I'm setting the seed exactly for that purpose: to have the same validation set all the time. I don't care about the order in which I receive the validation images. The goal is to compute a mean validation accuracy and loss.
The mean and std you adopted in this script are for ImageNet not CIFAR10 or CIFAR100
Author
kevinzakka commented on May 17, 2018
@sunkevin1214 nice catch! Fixed it now.
Using this I have len(train_loader.dataset) = len(val_loader.dataset)=60000, which is wrong.
Author
kevinzakka commented on Jun 14, 2018
@tan1889 that's because they both use the same underlying dataset, but a different sampler. You need to do len(train_loader.sampler)
instead.
@kevinzakka
im trying the pytorch firstly.
i used to use the keras and the dataset has 3 parts , train,valid,test.
but when i check the https://github.com/pytorch/examples/blob/master/mnist/main.py, it has train
function
and test
function
.
I cannot find the valid_dataset
,only the train_loader
and test_loader
So i think that the valid_dataset
doesn't to exist.
It confused me now.
Do you give me some explainations? thanks
The normalisation should only be done on the training set.But here the normalization is on the whole set. It should be a problem
Author
kevinzakka commented on Sep 5, 2018
@huangchaoxing validation and test sets should be normalized with train set statistics.
Author
kevinzakka commented on Sep 7, 2018
@sytelus the validation data is taken from the training set. The test set is untouched at all times.
amobiny commented on May 20, 2019 •
@kevinzakka
Hey Kevin and thanks for the gist.
I had a quick question about the valid_loader. How do you make sure that the validation sampler sweeps all the samples in the validation set exactly once? My understanding is that it takes batches of the provided indices randomly! so if we execute
for images, labels in valid_loader: ...
to for example compute the loss and accuracy over the validation (feed batch by batch and average), it will not do it correctly as it doesn't sweep the whole set once. Am I correct?
Author
kevinzakka commented on May 20, 2019
@amobiny I think you have sampler
and dataloader
confused. The dataloader
traverses the entire data set in batches. It selects the samples from the batch using the sampler
. The sampler
can be sequential so say for a batch of 4 and a dataset of size 32 you'd have [0, 1, 2, 3]
, [4, 5, 6, 7]
, etc until [28, 29, 30, 31]
. In our case, the sampler
is random and without replacement, in which case you'd have possibly something like [17, 1, 12, 31]
, [2, 8, 18, 28]
, etc. that would still cover the whole validation set. Does that make sense?
why does train & val not have same statistics usually for normalizing?
also the pytorch tutorials use 0.5 as opposte to:
test:
normalize = transforms.Normalize(
mean=[0.485, 0.456, 0.406],
std=[0.229, 0.224, 0.225],
)
and
train
normalize = transforms.Normalize(
mean=[0.4914, 0.4822, 0.4465],
std=[0.2023, 0.1994, 0.2010],
)
why get_train_valid_loader() return None-Type ?
also the pytorch tutorials use 0.5 as opposte to:
test:
normalize = transforms.Normalize( mean=[0.485, 0.456, 0.406], std=[0.229, 0.224, 0.225], )
and
trainnormalize = transforms.Normalize( mean=[0.4914, 0.4822, 0.4465], std=[0.2023, 0.1994, 0.2010], )
I can't speak the choice of transform used here, but from my own testing I will say that the transform applied to the train set should be the same as that of the test set. Prior to doing this, I was getting inconsistent accuracies on the test set when compared to the validation set. I chose to set both to
normalize = transforms.Normalize(
mean=[0.4914, 0.4822, 0.4465],
std=[0.2023, 0.1994, 0.2010],
)
Sign up for free to join this conversation on GitHub. Already have an account? Sign in to comment
Recommend
About Joyk
Aggregate valuable and interesting links.
Joyk means Joy of geeK