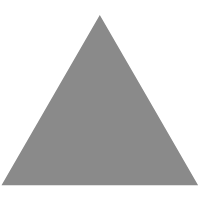
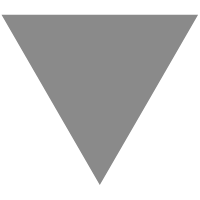
Without metadata, your fancy cloud data store is useless
source link: https://diginomica.com/without-metadata-your-fancy-cloud-data-store-useless
Go to the source link to view the article. You can view the picture content, updated content and better typesetting reading experience. If the link is broken, please click the button below to view the snapshot at that time.
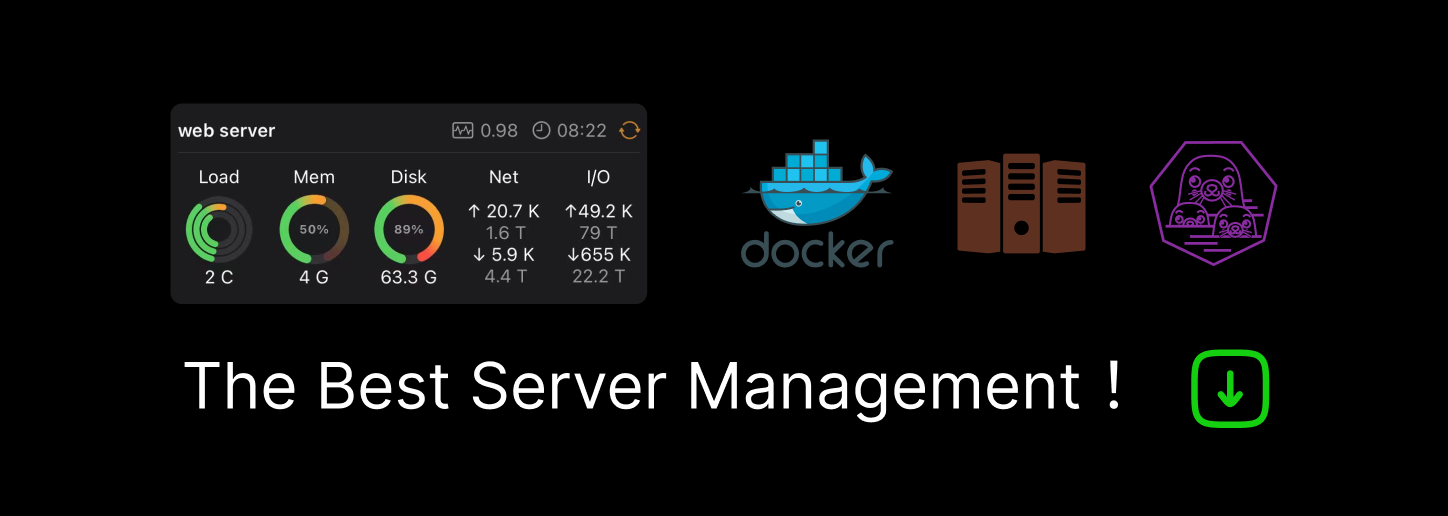
Without metadata, your fancy cloud data store is useless

(© Iconic Bestiary - Shutterstock)
In the process of gathering data for secondhand use, such as data warehouses and their many variants, organizations picked and chose pieces of data that fit their idea of what was needed to support specific applications of business Intelligence, visualization and other specific applications.
Today, organizations collect whole datasets, and many of them, without regard to a direct purpose other than the needs of data scientists and AI engineers. The freedom to vastly expand the scale of these loose repositories, first with Hadoop, then data lakes, and now cloud object stores and variants, allows for these extensive collections.
Understanding what is in the collection becomes more complicated. Data pulled for data warehouses typically came from "operational exhaust," not the digital exhaust of logs and streams and third-party data, including unstructured data not as easily searchable, including formats like audio, video, social media postings, spreadsheets, and email, all without a clear data model.
All of this data is relatively useless without extensive and current metadata. This isn’t something that a person or group of people can do effectively. Reasonable solutions are popping up using ML to crawl through data constantly and add or modify relevant metadata.
I wrote a few years ago: The real magic in applying machine learning models to a software product is producing the right mix of things that are general enough to work with a wide range of situations and powerful enough to produce non-trivial results repeatedly (useless example, "Most auto injury accidents occur when the driver is at least 16 years old.”) Supporting data science with Integrated (no code) tools requires creating and maintaining a comprehensive data catalog, but a few steps precede it.
Relationship discovery
If you think about it, the most crucial part of managing collections of unalike data is finding relationships. Finding relationships between many forms of information is practically impossible to do by hand. You are dealing with tabular/columnar data, figuring out what names will likely point to similar data (though not consistently accurate). Instead, the magic investigates the actual data to determine what it is.
To put this in perspective, if you have a few billion instances to compare, this can be a computationally expensive (read, slow) process. Here is the first example of machine learning boosting the process. An unsupervised machine learning model can quickly break down the similarities and converge on a solution. As the process flows through the data collection, it builds a relationship (graph) map that drives all of the elements of the system. Some powerful techniques that data discovery vendors are employing to find these relationships are:
- Recurrent Convolutional Neural Networks RCNN.
- Semi-Structured Data Parsing: Hidden Markov Model and Gene Sequencing algorithms.
Recommendations are provided to help the analyst join data sets, enrich the data, choose columns, add filters, and aggregate the data. The algorithms convert the mapping recommendation problem into a machine translation problem using:
- Encoder-Decoder architecture for primitive one-to-one mappings.
- Then using maximal grouping.
- An Attention Neural Network (ANN) is used to resolve the recommendation.
Machine learning-based discovery describes how data flows between databases and data sources and how data moves through the organization, discovering where data emanates and the affinities in the data itself.
What about sensitive data?
There are two types of sensitive data in sources. The first is the obvious personal information such as name, social security number, date of birth, and demographic, sociographic, and psychographic data. The problem is that this data may not be identifiable by looking at the column names or other available metadata. Only by examining the data can an algorithm decide the data is within the "sensitive realm."
But there is a deeper problem. Personally Identifiable Information (PII) is seemingly non-sensitive information that can be combined with other non-sensitive details to create an "emergent" identity. Additionally, data may be considered sensitive or confidential to an organization defined by company policy, which may also be considered "sensitive."
Considering these types of sensitive data, there are many issues where it is essential to manage the process. First are regulatory issues, such as the General Data Protection Regulation (GDPR). But there are also organizational promises to customers and suppliers to be good stewards of data you collect about them. It is relatively easy to govern these policies when a single internal system generates and manages the data. Still, if the data is scattered across sources and locations, gaps in governance and even the "emergent" problem can occur.
And finally, the connection between policy and digital processing is wide. The policy is stated in natural language, but how that policy is implemented in software can be pretty tricky.
Like a trend analysis, this captures changes in the source data at different points in time. For example, if new sensitive data is introduced into the database, impact analysis can determine when that occurred and quantify the delta.
Redundant data may, and usually does, have different modification cycles, leading to confusion. Generally, there aren't redundant data sources of primary enterprise data (though it happens). Still, other data sets can creep into the universe of sources, such as saved analysis outputs, training data sets and spreadsheets. The relationship map can identify these redundant sources and allow the analysts to choose the appropriate one.
Organizations can accumulate vast quantities of redundant data. They may be impacted by storage costs and unknowingly leave such data unmanaged and unprotected. Redundant data also requires management so organizations can decide on the appropriate remediation steps as part of the data management process once identified.
The automated data catalog is driven by relationship discovery. The whole point of a semantically rich data catalog is to provide analysts, data scientists, business and technology users (anyone who uses data, actually) a means to find the data needed, to understand what it means, how it relates to other data, its flow and to support collaboration and enable good data governance, data management and ultimately business analytics. Unlike proprietary metadata of an application, such as enterprise applications like ERP or CRM or the proprietary metadata of business intelligence and visualization tools, the catalog is not tied to a specific schema or model. Its generality is the key to its usefulness.
The most common repositories of metadata relate to customer and product domains. There is no doubt that these repositories are useful, but they lack perhaps 90 percent of the valuable data for analytics and data science.
Metadata management in the past
Metadata management did not present significant problems because organizations mainly dealt with highly structured data, built applications that were schema-on-write approaches (data structures were modeled before writing to DB), architectures were centralized, and stable processes were usually batch processing. All of this rendered metadata easy to handle and highly static simultaneously.
Metadata management struggled to keep pace with the introduction of data lakes, and homogeneity concepts were abandoned altogether. Instead, metadata had to deal with various structures and data types, non-homogenous data, schema-on-read, and many data sources, all of which demanded more modern metadata management solutions. Many approaches emerged, such as catalogs, data flow, and discoverability. Others were quite sophisticated full-fledged solutions with only narrow (vendor) applicability.
All of these different approaches had one thing in common: manual maintenance. The arrival of distributed data lakes, even the poorly defined data mesh, exacerbated the problem.
Today, the limitless data streams from business processes in finance, healthcare, manufacturing, insurance, and other industries and stream analytics compress the time between information demand and supply. It offers a way to boost performance and replace legacy batch, periodical operations with (near-)real-time ones.
Examples of today's data dynamicity:
- distributed and evolving domains
- highly non-homogeneous data platforms
- a variety of data and data sources
- the demand for real-time information,
- a multitude of different technologies
Altogether, they make so-called passive metadata management unusable in modern architectures.
Data has changed a lot in the past years. It is becoming prevalent for organizations to collect whole datasets, and many of them, without regard to a direct purpose other than the needs of data scientists and AI engineers. The freedom to vastly expand the scale of these loose repositories, first with Hadoop, then data lakes, and now cloud object stores and variants, allows for these extensive collections.
Understanding what is in the collection becomes more complicated. Data pulled for data warehouses typically came from "operational exhaust," not the digital exhaust of logs and streams and third-party data, including unstructured data not as easily searchable, including formats like audio, video, social media postings, spreadsheets, and email, all without a clear business purpose.
As a result, it is necessary to evolve how we handle metadata. Data has to be described, cataloged and visualized for use by the entire spectrum of data workers in the organization so that it is understandable, available and consistent.
Common and main issues with the legacy approach
Metadata management solutions built and maintained by manual management rarely provide sufficient value and inevitably lead to “shadow projects” that drain budgets and thwart efforts to standardize data management. These projects often looked reasonable initially but were victims of their success as the demand grew, causing increasingly manual interventions, delays and frustration.
Constrained by available staff and budget, many projects introduced artificial scale-backs to reduce or even halt the complexity of the diverse sources and uses. And further limited progress. Other single-purpose solutions resorted to APIs such as OWL, RDF, or SKOS, allowing more freedom for domain specialists and SMEs to explore federated architectures and decentralized responsibility, but it mainly remained manual.
Manual metadata maintenance is too inefficient, so metadata management solutions driven by automatic processes were the next step, using crawlers or agents. These techniques are basically “dumb,” as they attach themselves to data sources and report to metadata management services and usually collapse under surging complexity and become unmanageable.
My take
The metadata management aspect of data architecture is central to a successful transformation. Obsolete passive metadata management cannot service the organization’s needs for the emerging data architectures. It is a lot of work to place it with autonomous processes of active data management approaches. Comparing data governance techniques where metadata management plays a central role will be crucial in data mesh or fabric approaches.
Recommend
-
61
README.md diff-so-fancy diff-so-fancy strives to make your diffs human readable instead of machine readable. This helps improve...
-
37
I3buntu - ( Ubuntu server + I3wm ) In this project, you can install i3 from the Ubuntu server without installing anything that you don't need. Still Under development: For using these types of projec...
-
18
Cloud Sync Button Button/progress-bar with cloud synchronization animation in vanilla JavaScript, Vue.js and Web component formats
-
6
Useless Ruby Gems for your pleasure Posted: 2008-08-14 - Last updated: 2019-06-05 Tagged
-
5
Scaleout Metadata File Systems already store much of your data. What are they?An overview of Google Colossus, Facebook Tectonic, Microsoft ADLSv2, HopsFSTL;DR: A new class of hierarchical distributed fi...
-
10
Technical Articles
-
4
You Don't Need a Fancy PC for Data Science: Use These Cloud Platforms!December 23rd 2021 new story6
-
7
A Fancy Hover Effect For Your Avatar Temani...
-
16
Support is great. Feedback is even better."Thanks for your support and checking ou the launch. I'd love to know what's your impression about Gasby, the goods and the bads about the tool and where I can improve. Many thanks"...
-
6
Aug 9th, 2023Potluck × Is TypeScript Fancy Duct Tape × Back Pain × Cloud Service Rate Limits👇 Download Show
About Joyk
Aggregate valuable and interesting links.
Joyk means Joy of geeK