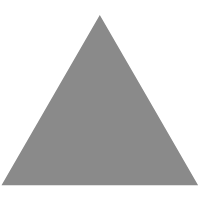
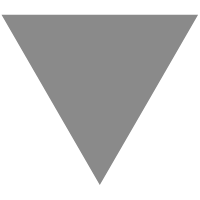
The Top Healthcare AI Trends Shaping the Industry
source link: https://devm.io/machine-learning/ai-healthcare-2022-trends
Go to the source link to view the article. You can view the picture content, updated content and better typesetting reading experience. If the link is broken, please click the button below to view the snapshot at that time.
Looking at the 2022 AI in Healthcare Survey
The Top Healthcare AI Trends Shaping the Industry
19. Jul 2022
The artificial intelligence (AI) in healthcare market is projected to grow to more than $67 billion by 2027—nearly doubling in size from 2021. Driven in part by the global pandemic, AI in healthcare startups and VC funding have exploded, and there are no signs of that slowing. But giving all the credit to COVID is oversimplifying the market.
To really understand the impact of Healthcare AI, we need to look at the trends driving it forward. The “2022 AI in Healthcare Survey” aims to do just that. Now, in its second year, Gradient Flow and John Snow Labs explored what 300+ technology professionals are experiencing in their AI programs. From the challenges to the solutions, and the use cases to the users, several key trends have emerged, and they’re worth taking note of.
1. Data Integration, BI, NLP, and Data Annotation named top AI technologies
When asked what technologies they plan to have in place by the end of 2022, technical leaders cited data integration (46%), BI (44%), NLP (43%), and new this year, data annotation (38% ). Text is now the most likely data type used in AI applications, and the prioritization of data annotation indicates an uptick in more sophisticated NLP technologies. With this comes more sophisticated NLP use cases, such as clinical decision support and matching patients to clinical guidelines. The pandemic has accelerated many areas of medical research, and drug discovery is no exception.
Text is now the most likely data type used in AI applications, and the prioritization of data annotation indicates an uptick in more sophisticated NLP technologies.
2. User bases shift from data scientists to domain experts
When asked about intended users for AI tools and technologies, over half of respondents identified clinicians (61%) as target users, and close to half indicated that healthcare providers (45%) are among their target users. Additionally, a higher rate of technical leaders cited healthcare payers and drug development professionals as potential users of AI applications. The shift from data scientist to domain expertise will continue and it’s a big step for democratizing the use of AI in healthcare and other industries.
3. Public cloud providers fall behind open source solutions
When asked what types of software respondents are using to build their AI applications, the most popular selections were locally installed commercial software (37%), and open source software (35%). With the emphasis on open source software came a 12% decline in use of cloud services (30%) from last year’s survey results (42%). With these changes come new entry points, and as healthcare becomes a bigger target for cyber attacks, practitioners need to be vigilant regardless of whether they use open source or cloud solutions.
4. Dealing with data in-house
A majority of respondents (53%) choose to rely on their own data to validate models, rather than on third-party or software vendor metrics. Respondents from mature organizations (68%) had a clear preference for using in-house evaluation and for tuning their models themselves. These data points aren’t surprising, as the healthcare industry is heavily regulated with strict laws around working with data. Protecting health information, especially regarding patients, is important and many healthcare organizations are rightfully wary of trusting other entities with it. Given the variety in EHR implementations and healthcare data, validating models on localized data makes sense.
The shift from data scientist to domain expertise will continue and it’s a big step for democratizing the use of AI in healthcare and other industries.
5. Production readiness is a top priority
Production readiness was the top criteria used to evaluate machine learning, NLP, and computer vision solutions among technical leaders, while respondents from mature organizations prioritized the ability to train and tune models. When evaluating locally installed software libraries or SaaS solutions, both technical leaders and respondents from mature organizations cited the availability of healthcare-specific models and algorithms. When you’re dealing with patients and medications, it’s easy to understand why model specificity and the ability to tune models to specific datasets and use cases is important.
Healthcare AI has made major strides over the past few years. With the increased investments and interest from a broader range of users, it’s easy to understand what’s fueling this growth. Given strict regulations and laws about protecting healthcare data and patient information, it’s encouraging to see AI thriving. And with ethical and responsible practices built in from the ground up, other industries can take a page out of the healthcare AI playbook.
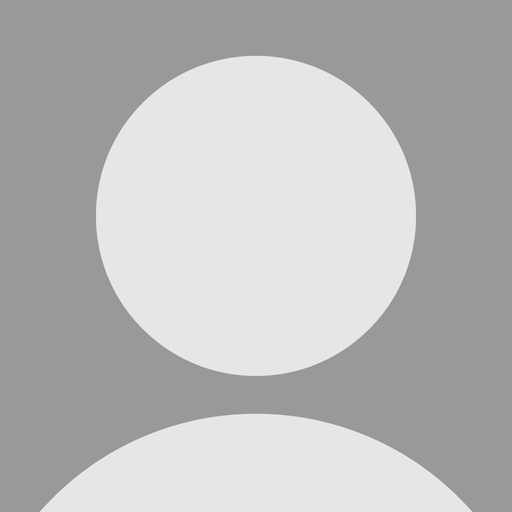
David Talby, PhD, MBA, is the CTO of John Snow Labs. He has spent his career making AI, big data and data science solve real-world problems in healthcare, life science and related fields.
Recommend
About Joyk
Aggregate valuable and interesting links.
Joyk means Joy of geeK