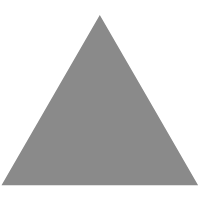
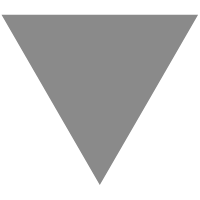
Product quality improvement using machine learning
source link: https://www.neuraldesigner.com/solutions/quality-improvement
Go to the source link to view the article. You can view the picture content, updated content and better typesetting reading experience. If the link is broken, please click the button below to view the snapshot at that time.
Product quality improvement using machine learning
Product quality improvement using machine learning
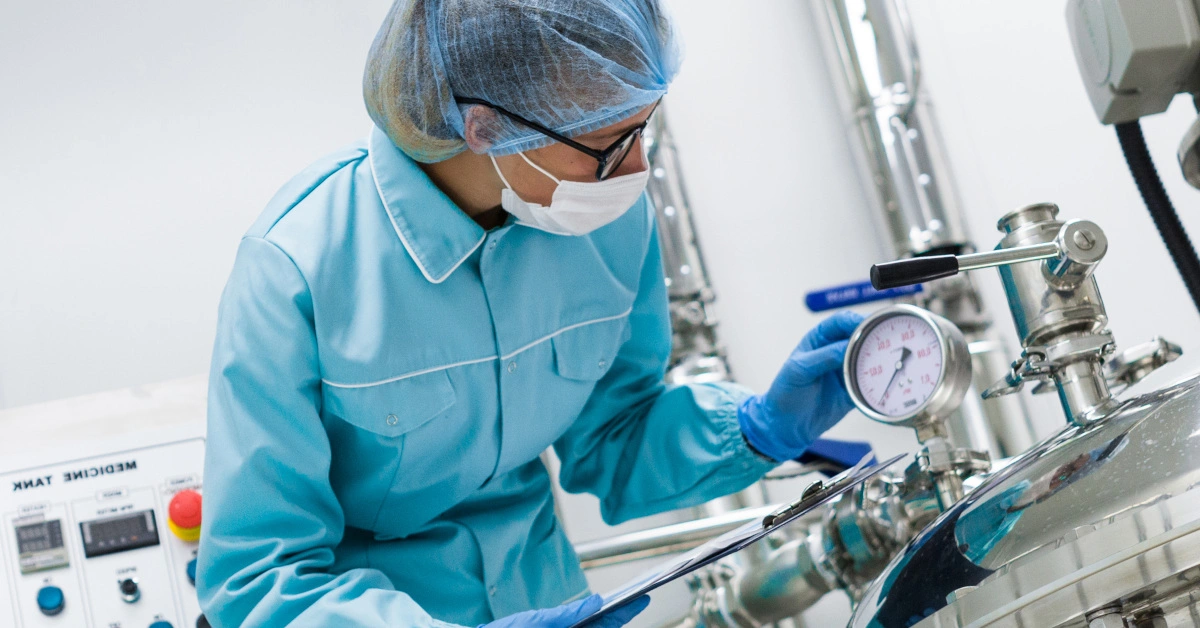
Quality improvement investigates the relationship between the features of a product and how well it complies with or conforms to its design.
This article explains how machine learning and Neural Designer can be used to model engineering systems and optimize industrial processes.
Contents:
On the other hand, the quality index can be objective (a physical or chemical test) or subjective (the evaluation by a person).
The data set contains measurements of our product.
It comprises the design and quality variables.
Design variables are those inputs that determine the quality of the product.
Some examples of design variables are:
- Proportions of product components.
- Physical or chemical measures of the product.
Quality variables are the outputs of the product and depend on the design variables.
Product quality optimization can consider different targets:
PRODUCT FEATURES
HUMAN PREFERENCES
Usually, we will have a single quality variable, although there may be several.
Mathematical model
The model of the quality of a product is a mathematical description that adequately predicts the quality of the product from the design variables.
More specifically, it relates the quality variables with the design variables.
quality_variables=function(design_variables)quality_variables=function(design_variables)
Neural networks are algorithms used to fit multi-dimensional and non-linear functions from data sets.
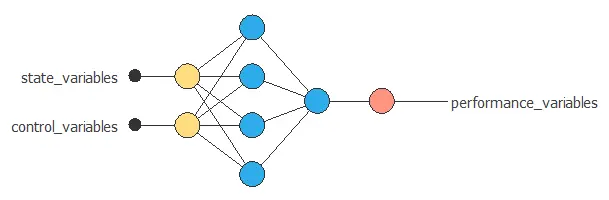
The inputs to the neural network include the design variables. The outputs from the neural network are the predicted quality of the product.
Response optimization
The objective of the response optimization algorithm is to exploit the mathematical model to look for optimal design variables.
Indeed, the predictive model allows us to simulate different possible products and adjust the design variables to improve quality.
More specifically, product quality optimization can be formulated as follows:
Determine the design variables that maximize the quality variables.
The next figure illustrates the response optimization process.
As we can see, the design values, (x1*,x2*), maximize the quality value.
A quality optimization problem might also be specified by a set of constraints on the product's inputs and outputs.
An example is to maximize the compressive strength of concrete while maintaining the amount of cement at the desired value.
Conclusions
Machine learning can predict the quality of a product based on its design.
That allows us to simulate different products and adjust the design to improve quality.
Some examples are to model wine preferences from physicochemical properties or to model the compressive strength of high performance concretes.
Neural Designer uses neural networks to model the quality of products. It also contains response optimization algorithms to fine-tune the design variables and optimize quality.
Do you need help? Contact us | FAQ | Privacy | © 2022, Artificial Intelligence Techniques, Ltd.
Recommend
About Joyk
Aggregate valuable and interesting links.
Joyk means Joy of geeK