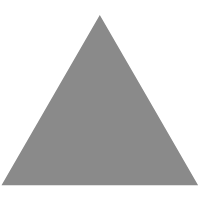
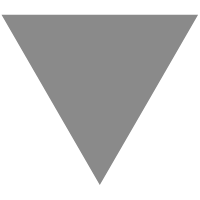
[1906.02896] Adversarial Explanations for Understanding Image Classification Dec...
source link: https://arxiv.org/abs/1906.02896
Go to the source link to view the article. You can view the picture content, updated content and better typesetting reading experience. If the link is broken, please click the button below to view the snapshot at that time.
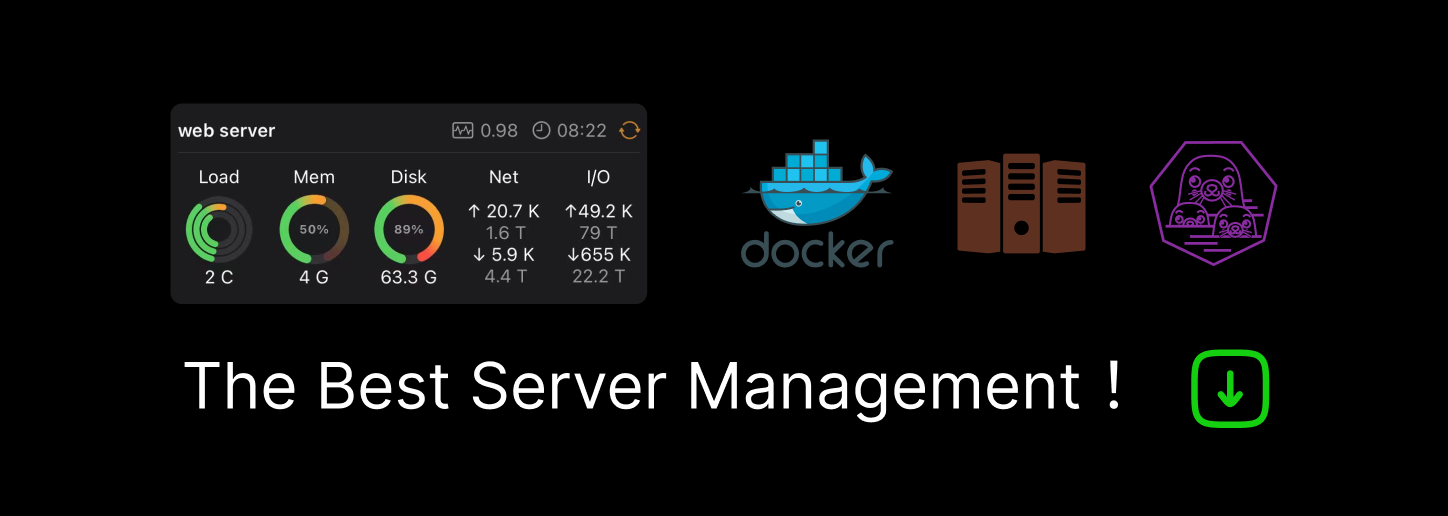
[Submitted on 7 Jun 2019 (v1), last revised 6 Aug 2019 (this version, v2)]
Adversarial Explanations for Understanding Image Classification Decisions and Improved Neural Network Robustness
For sensitive problems, such as medical imaging or fraud detection, Neural Network (NN) adoption has been slow due to concerns about their reliability, leading to a number of algorithms for explaining their decisions. NNs have also been found vulnerable to a class of imperceptible attacks, called adversarial examples, which arbitrarily alter the output of the network. Here we demonstrate both that these attacks can invalidate prior attempts to explain the decisions of NNs, and that with very robust networks, the attacks themselves may be leveraged as explanations with greater fidelity to the model. We show that the introduction of a novel regularization technique inspired by the Lipschitz constraint, alongside other proposed improvements, greatly improves an NN's resistance to adversarial examples. On the ImageNet classification task, we demonstrate a network with an Accuracy-Robustness Area (ARA) of 0.0053, an ARA 2.4x greater than the previous state of the art. Improving the mechanisms by which NN decisions are understood is an important direction for both establishing trust in sensitive domains and learning more about the stimuli to which NNs respond.
Comments: | 23 pages with a 14 page appendix. Submitted to Nature ML for peer review |
Subjects: | Machine Learning (cs.LG); Cryptography and Security (cs.CR); Machine Learning (stat.ML) |
Cite as: | arXiv:1906.02896 [cs.LG] |
(or arXiv:1906.02896v2 [cs.LG] for this version) | |
https://doi.org/10.48550/arXiv.1906.02896 | |
Journal reference: | Nature Machine Intelligence (2019) |
Related DOI: |
Recommend
-
85
README.markdown
-
71
README.md JavaScript Algorithms and Data Structures
-
81
README.md Adversarially Constrained Autoencoder Interpolations (ACAI) Code for the paper "Understanding and I...
-
105
README.md AI Fairness 360 (AIF360 v0.1.0)
-
24
GAN dissection: visualizing and understanding generative adversarial networks Bau et al., arXiv’18 Earlier this week we looked at visualisations to aid u...
-
16
– Fighting mass image recognition Choose JPG or PNG (up to 1 Mb) a...
-
6
Introduction Thanks to the Predictive Scenarios you were already able to answer predictive questions like “which employees are at risk of leaving my company” (classification) or “how long will it take to get my invoices paid” (regres...
-
5
Born On This Day In 1906 Grace Hopper - Born On This Day In 1906 Written by Sue Gee Thursday, 09 December 2021 Today, December 9th 2021, is the 115th annive...
-
10
The 1906 San Francisco Earthquake and Fire
-
9
Announcing Experimental `valuable` Support #1906 ...
About Joyk
Aggregate valuable and interesting links.
Joyk means Joy of geeK