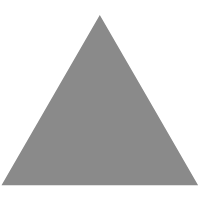
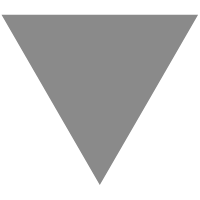
How to Add Value as a Data Analyst
source link: https://towardsdatascience.com/how-to-add-value-as-a-data-analyst-8a6ae900b82a
Go to the source link to view the article. You can view the picture content, updated content and better typesetting reading experience. If the link is broken, please click the button below to view the snapshot at that time.
How to Add Value as a Data Analyst
The journey to becoming a “real” data analyst
Let’s start with a quick summary of three common misconceptions about analytics:
- Analytics is statistics. (No.)
- Analytics is data journalism / marketing / storytelling. (No.)
- Analytics is decision-making. (No!)
Photo by Alexander Sinn on Unsplash
Misconception #1: Analytics versus statistics
While the tools and equations they use are similar, analysts and statisticians are trained to do very different jobs (learn more here):
Analytics helps you form hypotheses, improving the quality of your questions.
Statistics helps you test hypotheses, improving the quality of your answers.
Misconception #2: Analytics versus journalism/marketing
Analytics is not marketing. The difference is that analytics is about expanding the decision-maker’s perspective while marketing is about narrowing it.
Analytics is about expanding the decision-maker’s perspective while marketing is about narrowing it. The analyst serves their decision-maker(s) first and foremost.
Similarly, data journalism/storytelling is about capturing the interest of many people in a small way, while analytics is about serving the needs of a few people in a big way. The analyst serves their decision-maker(s) first and foremost. (Learn more here.)
Data journalism/storytelling is about capturing the interest of many people in a small way, while analytics is about serving the needs of a few people in a big way.
Misconception #3: Analytics versus decision-making
Analytics serves decision-making, but it’s a separate role.*
If I’m your analyst, I’m not here to choose for you (even though I might have more domain expertise than you). You’d have to promote me to decision-maker for that to be an ethical thing to do.
If you want someone to work as an analyst-decision-maker hybrid, understand that you’re asking for two roles rolled into one and assign that responsibility explicitly. (Learn more here.)
*A question I get asked frequently is, “What should we do if the decision-maker I’ve been asked to serve is incompetent?” Quite right — things get tricky if your decision-maker is unskilled. Head over here for my answer.
Amateurs versus professional analysts
Now that we’ve reviewed some misconceptions about the role, we’re ready to get back to our journey through the list of differences between an amateur and a professional analyst. We covered the first 7 in detail in my previous articles [1] [2] [3] and now we’re ready for #8.
Data pro vs amateur difference #8 — Knowing how to add value
People keep listing “knowing the business” as part of an analyst’s responsibilities, but there’s rarely a clear explanation of how this relates to their value.
Those who are employed in hybrid roles do a hell of a job of confusing those who aren’t.
Part of the confusion comes from misunderstanding the difference between a decision-maker’s job and an analyst’s job (see above). Those of you who are employed as decision-maker-analyst hybrids do a hell of a job of confusing those who aren’t. This might help clarify things:
- An analyst serves their decision-maker(s).
- A decision-maker serves their business.
If you’re a hybrid of both roles, knowing the business is table stakes. As a decision-maker, defining how value should be added, setting priorities, and seizing opportunities to optimize the business is your direct responsibility. I could write reams on the topic, but this blog post is about analysts, so let’s focus on the non-hybrid version.
The what and the why - that’s on the decision-maker. The how - that’s on the analyst.
When does a decision-maker need an analyst? When they don’t have the time to process, explore, and summarize all available potentially useful information themselves. In other words, the analyst acts as a sort of sensory organ for their decision-maker. A decision-maker’s job is to turn information into better action, which is hopeless if they can’t access much information. That’s where the analyst comes in.
Applying your listening skills is another kind of analytics, as I explain in this video.
To be valuable, an analyst must learn to serve their decision-maker(s) effectively. You know what’s even better than business knowledge for this? Listening skills. Yes, the job involves another kind of analysis: conversations with real people, not just a pile of data. You’ll also need great communication skills, since you’ll be augmenting your decision-maker’s senses… so you’ll need to get that information smoothly from your screen into your decision-maker’s brain. The what and the why, that’s on them. The how, that’s on you.
Of the three breeds of data science professional, analysts are the most likely heirs to the decision throne.
A basic way for an analyst to serve a decision-maker is as their search engine. The decision-maker wants to know some factoid about the business and you look it up for them. This is reactive analytics and we tend to think of it as the beginner stuff in analytics — the expert work is proactive: it’s all about extracting inspiration from data. (Learn more here.)
Analysts thrive in ambiguity. Their talent is exploration, which makes them particularly good at foreseeing and responding to crises.
The most valuable contribution an analyst can make is inspiring a decision-maker to consider courses of action they didn’t know they needed to think about. In other words, successfully hunting for unknown unknowns worth knowing about.
Business leaders who strive to be true innovators invest in analytics.
In good times, we call this kind of work “fueling innovation” and “finding new opportunities to take advantage of.” When the going gets tough, we start talking about “identifying threats” and “preventing disasters before they happen.” In hard times, what looked like a nice-to-have innovation booster turns into a must-have safety net, but both are two sides of the same coin: exploring information to inspire leaders to ask questions they might not have considered otherwise. (Getting those questions answered is a different job.) Learn more here.
An analyst’s job is to inspire executives with thought-provoking yet qualified possibilities.
An analyst who is laser-focused on serving their decision-maker works hard to be as useful as possible: they do proactive data exploration, anticipate follow-up questions, and generally avoid wasting their decision-maker’s time. To do this, they must gradually develop a keen nose for what’s important in addition to what’s interesting, which means that they naturally absorb some of their decision-maker’s expertise.
The most valuable contribution an analyst can make is inspiring a decision-maker to consider courses of action they didn’t know they needed to think about.
You can’t produce an actionable insight if you don’t understand anything about how your decision-maker uses information to select their actions. Although the actionability of an insight is technically not the analyst’s responsibility — it’s the decision-maker’s — in practice the line gets blurry over time.
That’s why, of the three breeds of data science professional, analysts are the most likely heirs to the decision throne… if that’s a job they want. (There’s plenty more to learn that won’t come for free and making the switch isn’t recommended if data is your true love.)
When it comes to developing the ability to figure out where things are heading and respond nimbly to a changing environment, nothing is more important than analytics.
To summarize this section, amateurs often miss the point of analytics and lack a coherent understanding of where the value comes from. Professional analysts understand it like this:
If you’re a decision-maker hybrid, your value is judged by the quality of your decisions and the actions you take. Your analytical skills are a means to that end — analytics is just one of many weapons in your arsenal.
If you’re a pure analyst, your value is tied to your ability to serve your decision-maker, both reactively (by looking up data that answers their specific questions) and proactively (by exploring data to inspire them to consider new directions, making them more innovative and threat-proof). Starting anywhere except with your decision-maker’s needs and priorities is bound to lead you astray.
Photo by Tim Mossholder on Unsplash
That’s it for this article. In the next one, we’ll continue with the last two differences between amateurs and professional analysts:
Data pro vs amateur difference #9 — Thinking differently about time
Data pro vs amateur difference #10 — Nuanced view of excellence
Let me know if you’re enjoying this topic and don’t forget to share your favorite insights with your community!
Data pro vs amateur differences #1-#3
Software skills; handling lots of data with ease; immunity to data science bias. Covered in part 1.
Data pro vs amateur differences #4–#6
Understanding the career; refusing to be a data charlatan; resistance to confirmation bias. Covered in part 2.
Data pro vs amateur difference #7
Realistic expectations of data. Covered in part 3.
Recommend
About Joyk
Aggregate valuable and interesting links.
Joyk means Joy of geeK