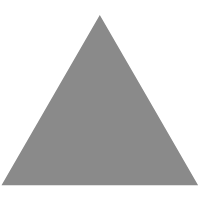
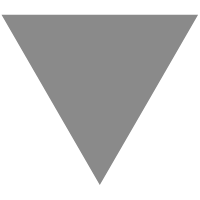
How to avoid unconscious bias in user persona creation
source link: https://uxdesign.cc/how-to-avoid-unconscious-bias-in-user-persona-creation-614d96b4e2e8
Go to the source link to view the article. You can view the picture content, updated content and better typesetting reading experience. If the link is broken, please click the button below to view the snapshot at that time.
How to avoid unconscious bias in user persona creation
Understanding the types of bias can help ensure they do not skew your user personas and research design. Let’s cover some do’s and don’ts!
Personas are like the characters in your everyday commercials. In a time-crunched sneak peek, they tell stories about partially imagined, but still research-based customers.
Representing an ideal, yet biased archetype in most scenarios, they are a particular, merged segment of various demographic and psychographic data. The resulting profiles are semi-fictional, yet realistic blueprints hinting at traits and behaviours of your intended user or consumer.
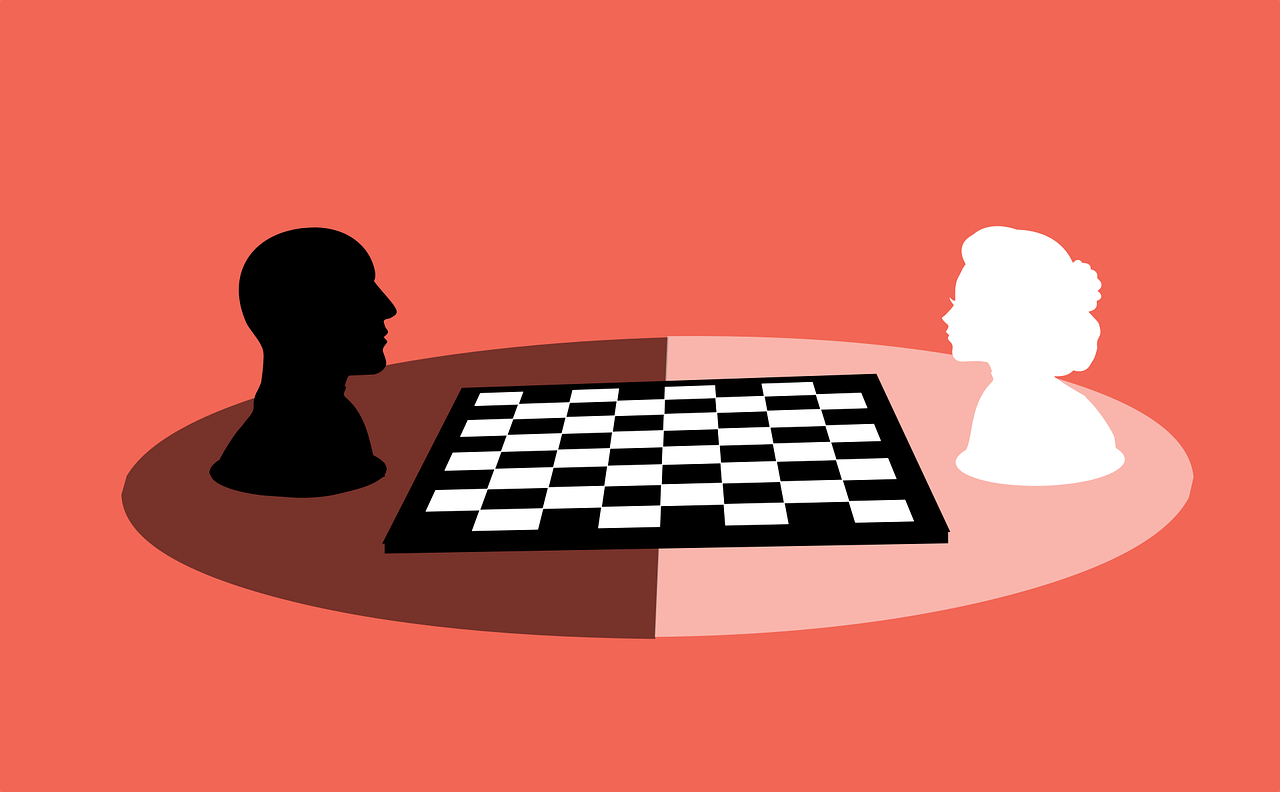
After all, no sensible advertisement features the polar opposite of what they deem their target audience. You can’t sell a product to someone who doesn’t even want it — let alone, if their lifestyle doesn’t require its existence, at all.
Hence, there is a valid grain of truth in their most basic element of stereotyping; namely that they don’t ignore what you have to offer.
When it comes to avoiding bias in research and design, it can be quite like walking through a minefield. Most of the time you will be unaware of the notions you hold in your mind, just like those sneaky land mines hidden under the surface.
However, accounting for bias is essential, in order to catch out any discrimination that should be wiped out from your sampling and analysis. At its core, it’s a moral obligation and ethical principle to prevent systematic errors that would then carry over into the making of the product.
Areas of Interest
Qualitative persona creation has tremendous potential in helping us understand customers. It’s an undoubtedly useful tool employed in multiple disciplines such as:
- marketing,
- education,
- economics,
- health care,
- and software development, just to name a few.
Ever since American software designer and programmer Alan Cooper popularised this design technique, it has been gaining traction. With the spotlight focused on human-centered design recently, the utilisation of user personas is unlikely to go anywhere soon.
Nonetheless, some fine-tuning may be still required to eliminate preventable toxic errors from their make-up.
Understanding Bias
Chances are you wouldn’t be hard-pressed to find a ripe example of bias in your surroundings. Making judgements about anything and everything is basically an imprint that comes with being human.
These instinctive snippets can be influenced by our:
- background,
- culture,
- fleeting interactions,
- and the media we consume consciously or unconsciously.
But our observations usually have to fit into some pre-calibrated mental mould. This is where shortcuts in thinking and predictive tendencies come to the rescue.
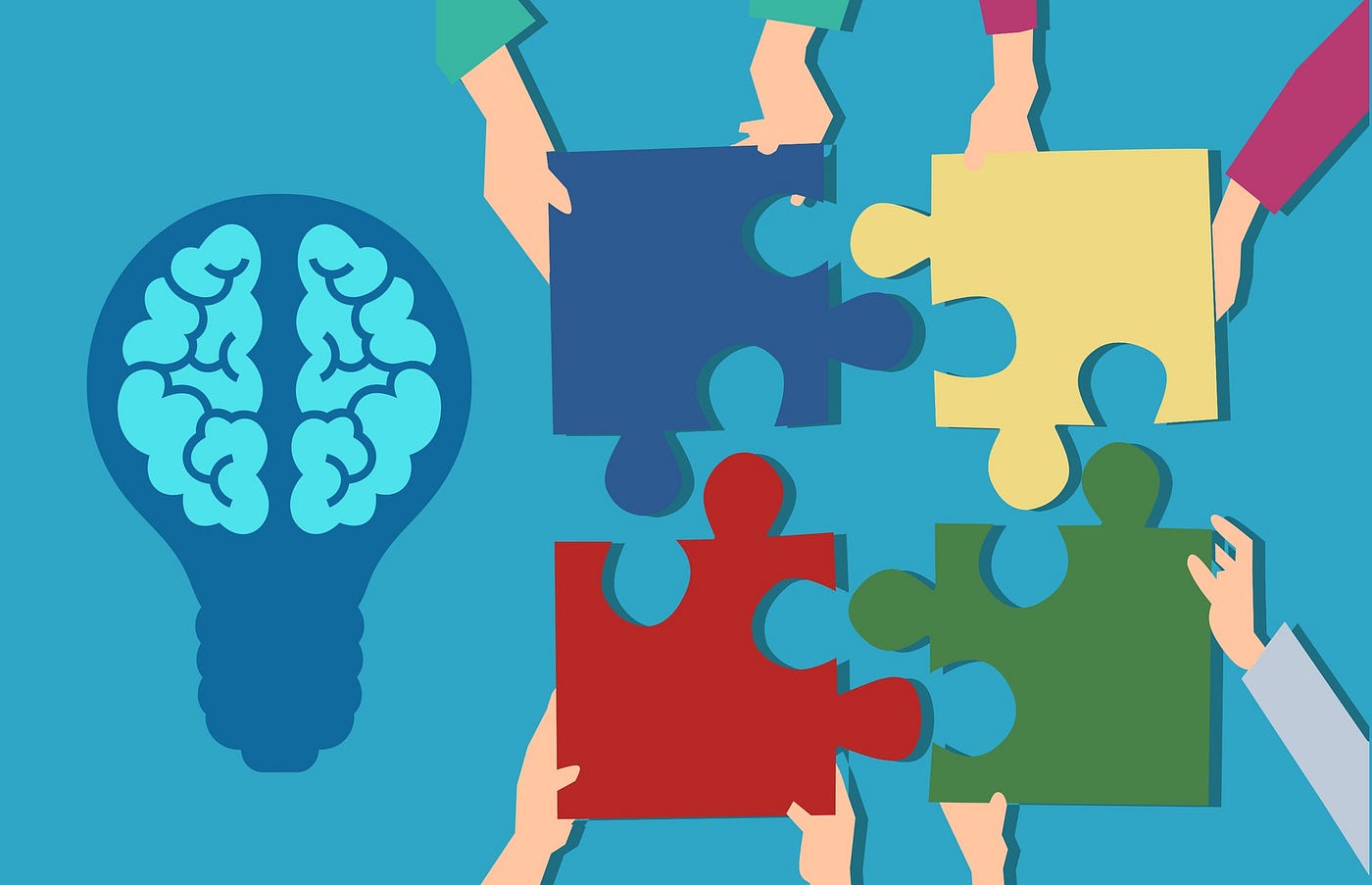
The Nobel Prize-winning Daniel Kahneman and his fellow researcher Amos Tversky have explored this behaviour (and others), in their body of work.
The main message here is that our minds operate on incomplete information. Hence, there has been solid evolutionary value in using mental models to fill any gaps in our thinking.
System 1 & System 2 Thinking
Kahneman took this further in his best-selling book, “Thinking Fast and Slow.” I won’t dive into that in much depth here, but in a very brief nutshell, he divides our thinking into two sub-systems.
➤ System 1 is fast, automatic, and does 98% of our thinking. It is our unconscious, auto-pilot mode. Because this type of thinking seeks out intuitive shortcuts, it saves mental energy. However, it can lead to more mistakes in complex situations as it’s more impulsive.
➤ System 2 is slow, deliberate, and does the lesser part (merely 2%) of our thinking. It is logical and seeks out new or missing information to paint a sound, rational picture. Because it involves more agency and in-depth analysis, this is the more effortful method.
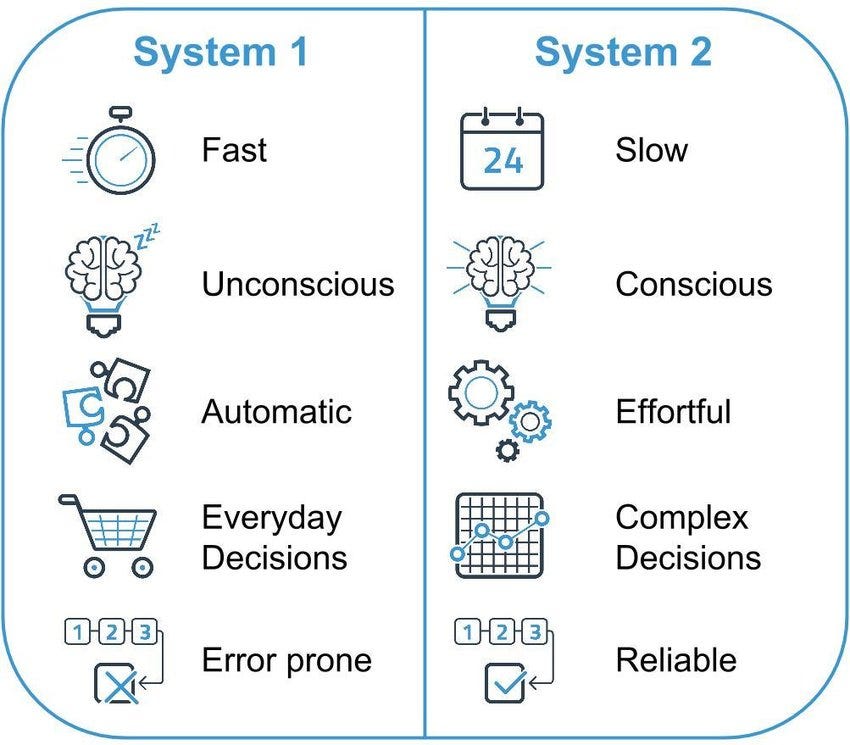
These two types of thinking work in tandem, despite System 1 preferring to run the show for comfort and ease. Now, both systems are susceptible to cognitive biases.
For example, confirmation bias (seeking out information that supports our beliefs) can show up in either, albeit in different forms. That is to say, there is not a “good” or “bad” type of thinking system, as such.
“Intelligence is not only the ability to reason; it is also the ability to find relevant material in memory and to deploy attention when needed.”
- Daniel Kahneman
Bias exists in research just like in most undertakings in life. The issue here is that wonky design implementations can have widespread consequences.
Misinterpretation of data is never good news, especially once it goes live in practice. The result? Enforcing stereotypes and one’s bias, paying little to no heed to ethical practices and inclusivity.
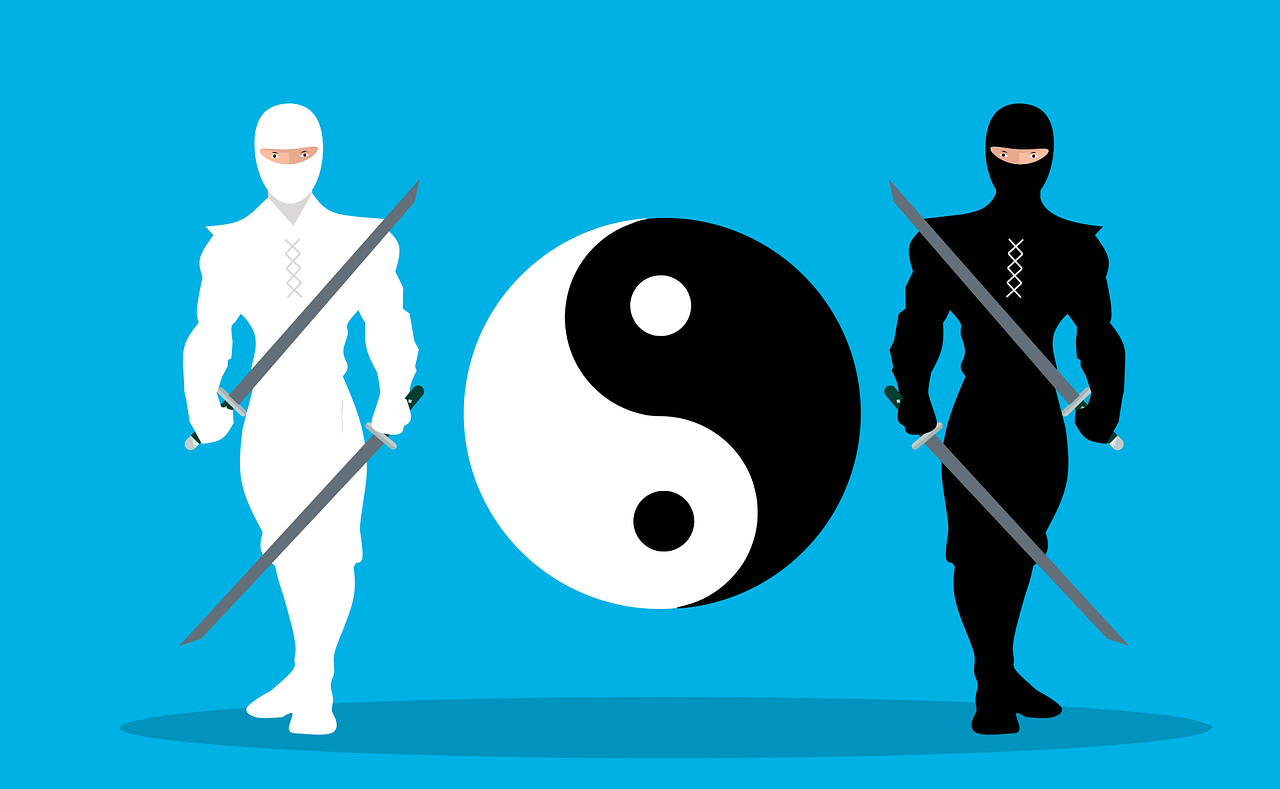
Types of Bias in Qualitative Research
Different courses, curriculums, books and articles will focus on a distinct collection of terms. This varying ensemble of bias categories can seem quite confusing at first glance.
Here I am going to focus on those specifically featured on the researcher’s side that can impede the research design itself.
➊ Design bias.
This happens when the researcher doesn’t notice or acknowledge bias in the research design. This could apply to the research method, along with its utility and suitability. There is a clear trade-off in quality if bias is ignored in favour of speedy, cheap data collection.
➋ Sampling / participant bias. (Also known as selection bias.)
When recruiting participants, you will likely aim for a sample that fits relevant categories, i.e. one that checks as many boxes as possible. That is all fair and well. However, note when certain classes of individuals or certain experiences are shown more consideration than others.
Omission bias happens when you exclude people from, say, selected age groups. Inclusivity bias is the opposite of this: it’s the method of selecting a sample for the sake of convenience. One way to fix this is by developing effective screening questions for participant selection.
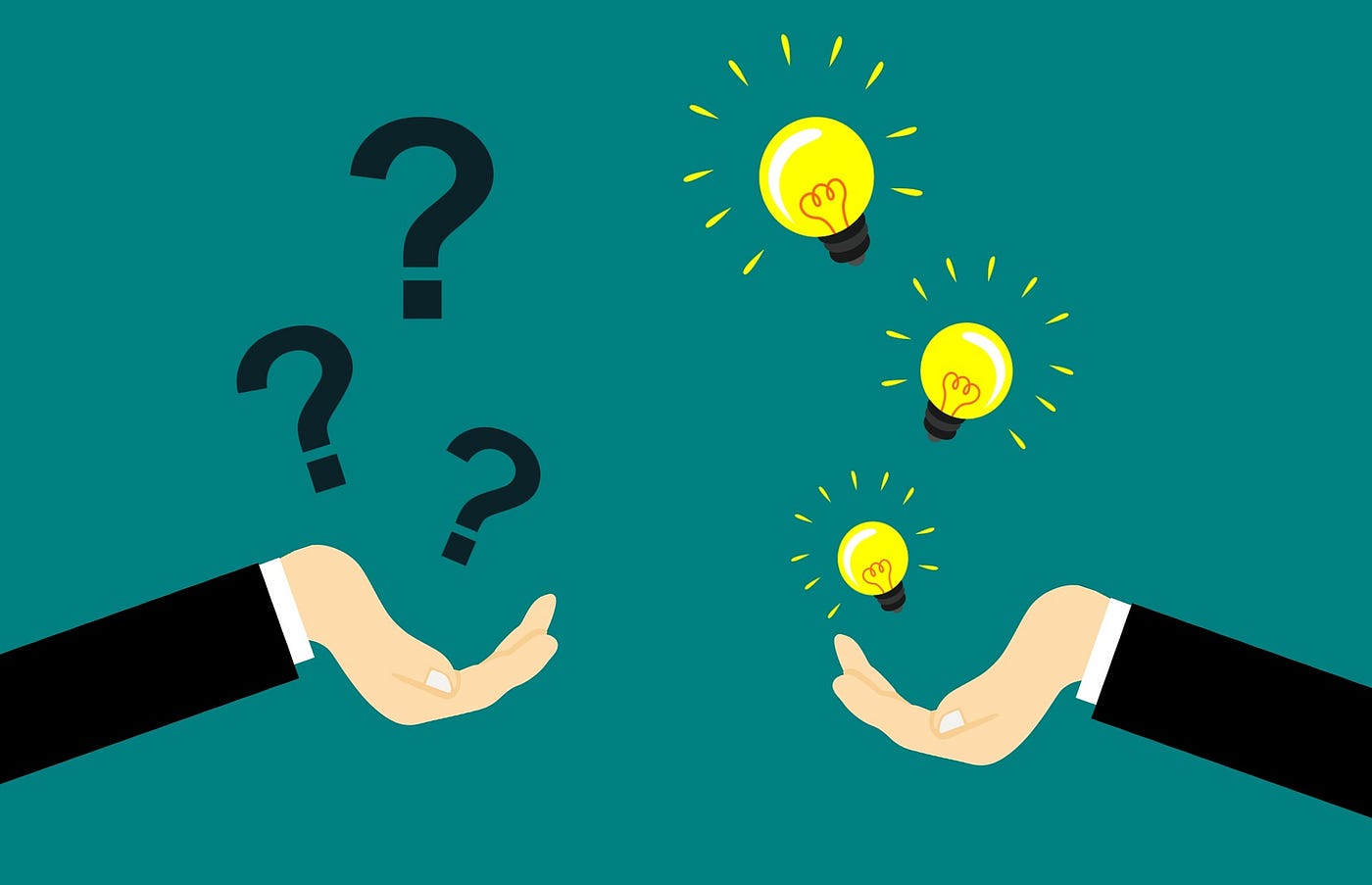
➌ Data collection bias.
How leading are your questions during interviews or when framed in surveys? Rather than asking “did you enjoy our excellent service?”, aim for a far more neutral “how would your rate our service?”
It is quite an extreme example, but it shows that questions should not come with a pre-set or preferred answer. Invest in interviewer or moderator training to help curb these errors.
➍ Measurement bias.
Can you vouch for the accuracy of your collected information? If data is ambiguously measured or classified, consider it a red flag. For example, participants may not recall and report facts properly when relying on memory, skewing or undermining the overall standard of information.
➎ Analysis bias.
When looking at your research topic and data, you may feel inclined to observe outcomes in favour of your claims. You may rightfully deduce certain patterns and behaviours confirming your assumptions.
Still, be aware of your ingrained ideas and aim to retain your objectivity when analysing results. (In other words, pay attention to your own cognitive biases.)
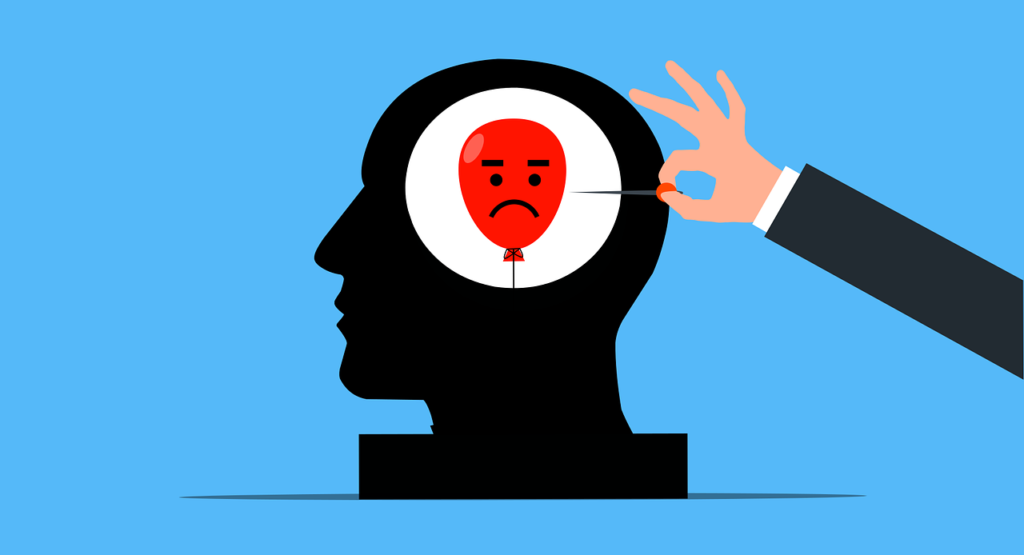
Flawed Construction of User Personas
Reaching for (oftentimes falsely) trusted archetypes carries a risk from the beginning. Research based on a single, fictitious person that should be representative of an entire audience is a strict no-go in science.
In contrast, more commercial settings seem to favour a quick answer and an easily deployable solution. The by-product of vague conclusions derived from these muddy summaries tends to be ignored or not even grasped.
“People only see what they are prepared to see.”
- Ralph Waldo Emerson
Controversies can arise when a user persona is created vastly based on imagination rather than facts. If the foundation is not solid, empathy for real humans can get erased in the process. This then gives rise to a product designed for only a chunk of your actual target audience. The better outcome is that you will simply be catering to a set of super-customers you deem relevant, whilst others receive a lesser, but still manageable treatment.
Casting aside your edge cases is not particularly problematic. In fact, it is what we deem best practice. However, ignoring a filtered-out share of users means your study was not conducted properly from the beginning.
Financial loss and wasted time yielding limited results is one issue here. The other is a bit more significant in the long term. Incomplete research then equates to social deficiencies in what was supposed to be your attempt at gauging your customer base.
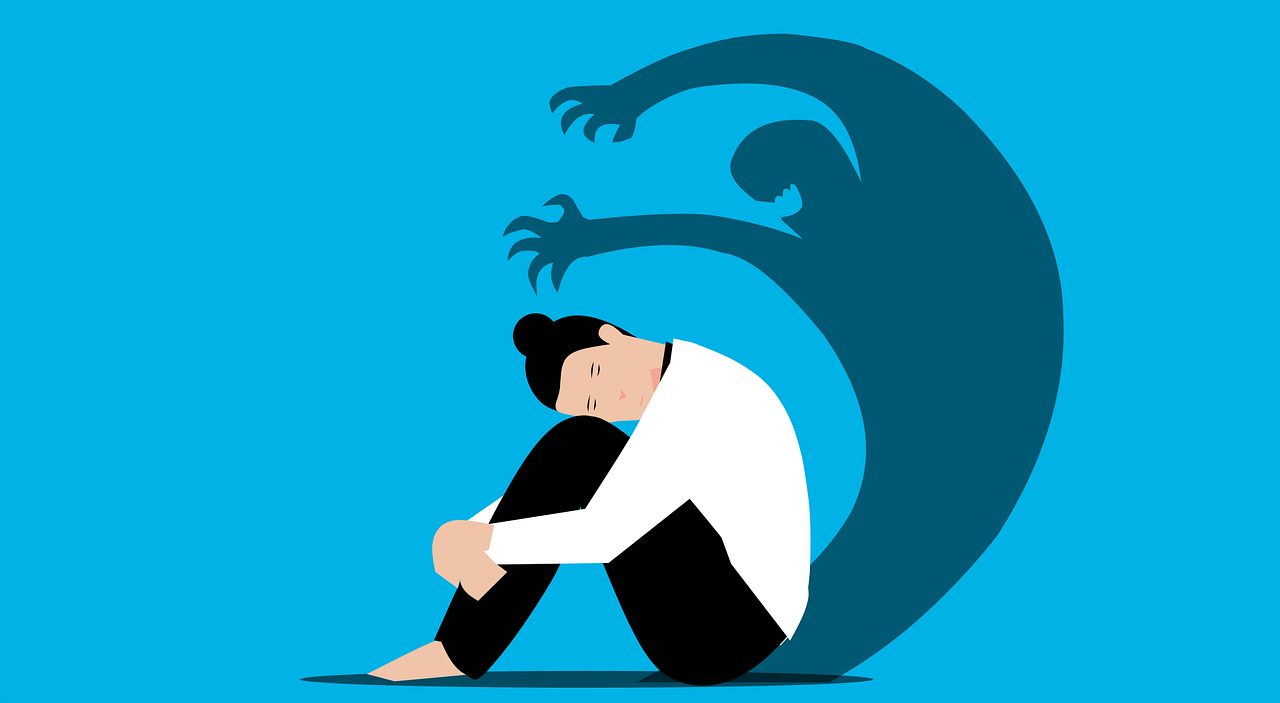
Do This, Not That
Below are some pointers to keep in mind when researching and developing user personas.
1) Sampling & Categories:
➤ Aim for a larger sample size. This may well be one of the most important steps. To even-out imbalances and spot shortcomings in your research, the depth and diversity of the sampling itself is vital.
✘ Too much demographic data creates bias. Focus on the necessary details only. If not essential, leaving out gender, age, race, relationship status and/or education could be beneficial to reduce implicit bias. Other factors could include location or country of origin. Leverage this data with care and employ what is truly useful, not hindering.
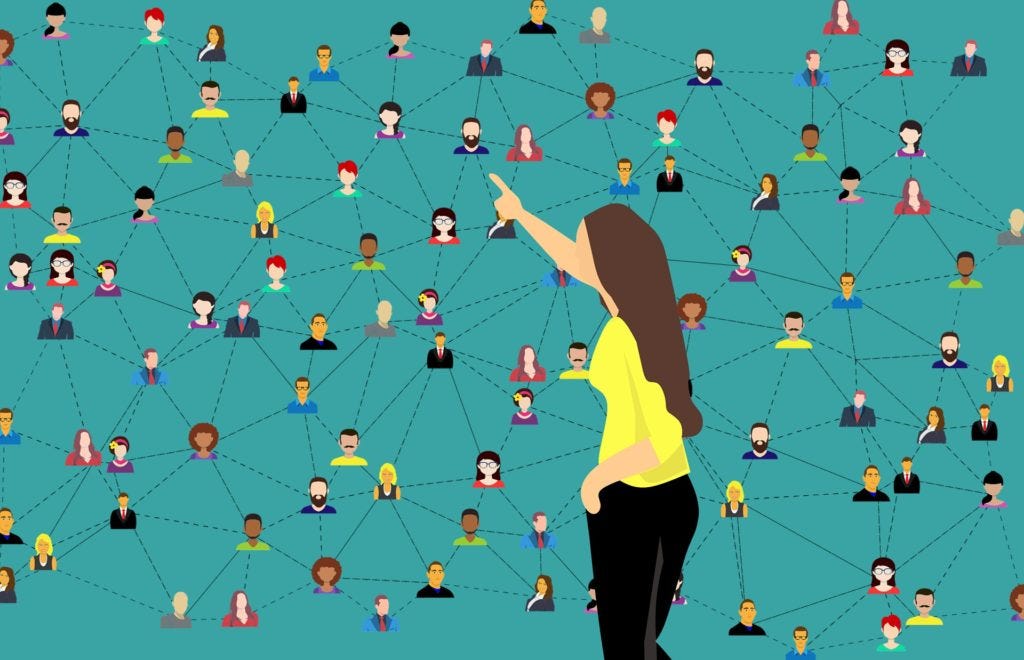
2) Persona: Keeping It Timely and Relevant
➤ Keep your persona fresh and relevant. Conduct additional research on a set timely basis (e.g. annually), and update your documentation so they don’t become outdated. Sharing new findings is important, so your persona stays suitable for your product and representative of your target audience.
✘ Don’t create too many personas. Your personas should be relevant and have good portrayals of your customers. Note that this doesn’t mean you have to create a new one for each characteristic you identify during your research.
3) Persona: Generic vs Specific
➤ Make your persona relatable. Instead of using picture-perfect stock images, you could omit their visuals entirely. Perhaps you could use more realistic photos taken from research interviews. Alternatively, replace it with a simple silhouette or another minimal graphic.
✘ Don’t let your persona become either over-generic or over-specific. You’ll be walking a tightrope here. Overloading your persona’s profile with superfluous details, irrelevant to the product, will not help your case.
However, this also goes for the other extreme end of the scale. If your persona could be applied to any and every person you pass on the street, that renders it unusable.
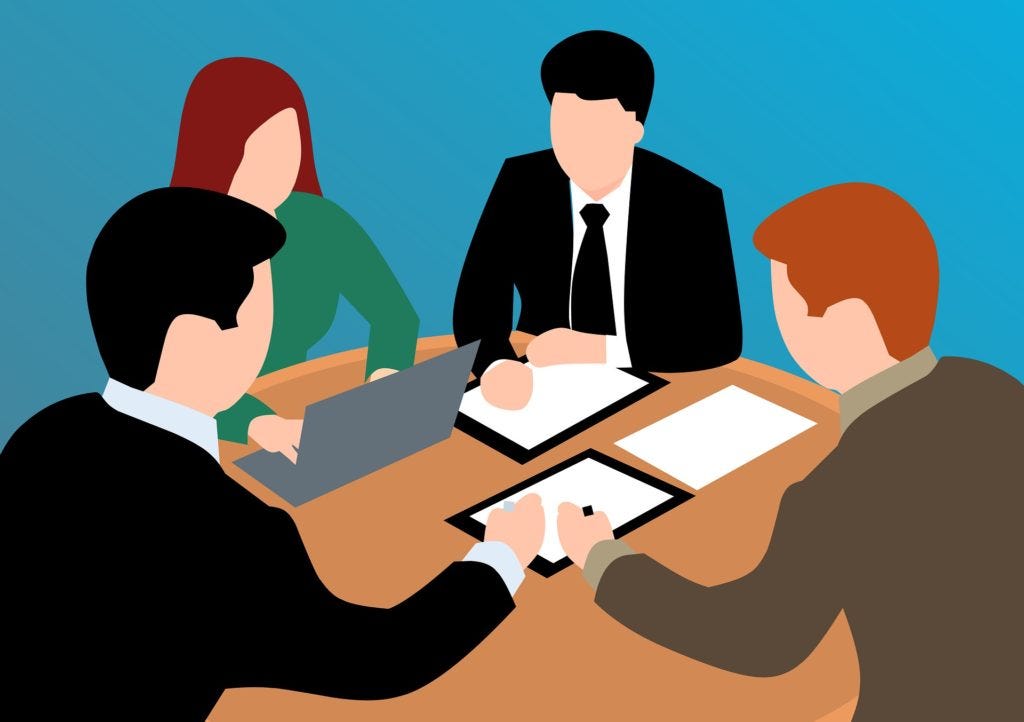
4) Teamwork Makes The Dream Work
➤ Involve your stakeholders in the process. It is important that management and teams are aware of the aim and use of the personas you are creating. This helps avoid confusion and misinterpretation down the line.
✘ Don’t ignore motivations and expectations. Naturally, you shouldn’t treat personas’ motives like bunnies whipped out of a magician’s hat. Base them on interviews, surveys and other types of qualitative research methods. Your own assumptions should not take the lead here, either.
The Bottom Line
As meta as it may sound, keeping track of your own mind’s thinking models is a valuable skill to possess. Since they can extend to your qualitative research, try to stay wary of bias and its many shades, so they don’t show up to skew your analysis.
Further Reading
References & Credits
- Figure 1: SAVING TAX DOLLARS; SAVING LIVES: USING NUDGE THEORY TO ELIMINATE OUTDATED EMERGENCY LOCATOR TRANSMITTERS (ELTs) — Scientific Figure on ResearchGate. Available from: https://www.researchgate.net/figure/System-1-vs-System-2-Thinking-upfrontanalyticscom-nd_fig3_335840928 [accessed 3 May, 2022]
- Images by Mohamed Hassan, Pixabay
Recommend
About Joyk
Aggregate valuable and interesting links.
Joyk means Joy of geeK