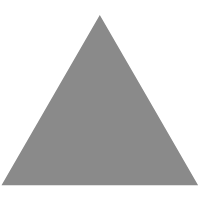
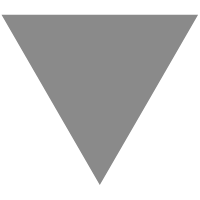
A Lagrangian Approach to Information Propagation in Graph Neural Networks
source link: https://mtiezzi.github.io/publication/2020-03-17-lpgnn
Go to the source link to view the article. You can view the picture content, updated content and better typesetting reading experience. If the link is broken, please click the button below to view the snapshot at that time.
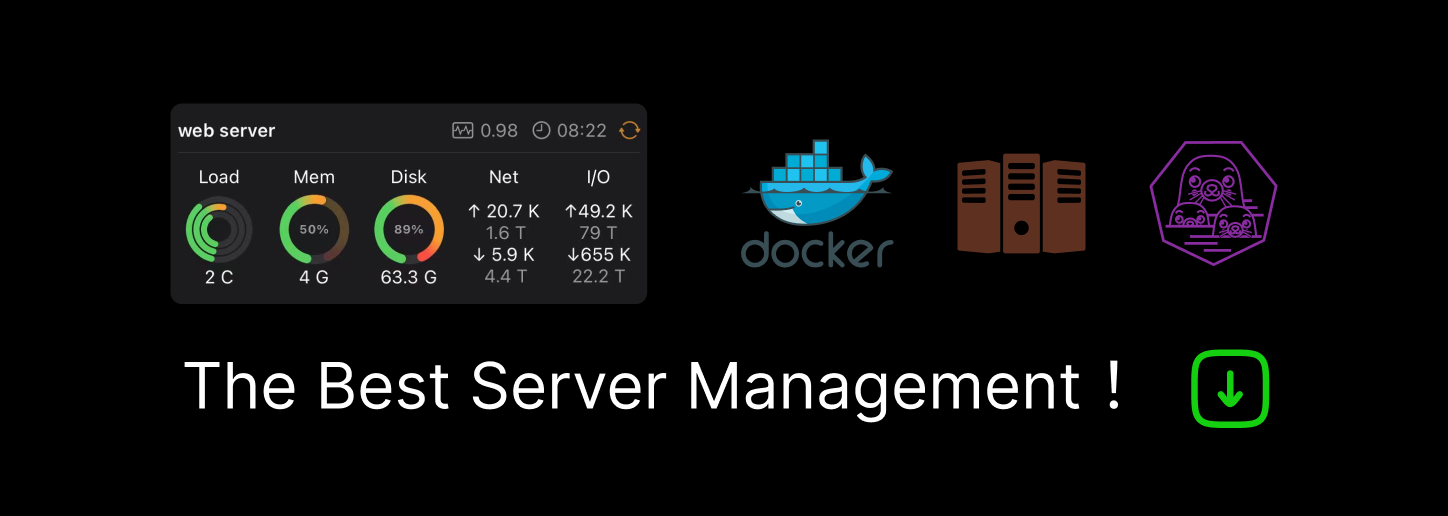
A Lagrangian Approach to Information Propagation in Graph Neural Networks
Published in ECAI2020, 2020
Recommended citation: Matteo Tiezzi, Giuseppe Marra, Stefano Melacci, Marco Maggini and Marco Gori (2020). "A Lagrangian Approach to Information Propagation in Graph Neural Networks; ECAI2020 - http://ebooks.iospress.nl/publication/55057
In many real world applications, data are characterized by a complex structure, that can be naturally encoded as a graph. In the last years, the popularity of deep learning techniques has renewed the interest in neural models able to process complex patterns. In particular, inspired by the Graph Neural Network (GNN) model, different architectures have been proposed to extend the original GNN scheme. GNNs exploit a set of state variables, each assigned to a graph node, and a diffusion mechanism of the states among neighbor nodes, to implement an iterative procedure to compute the fixed point of the (learnable) state transition function. In this paper, we propose a novel approach to the state computation and the learning algorithm for GNNs, based on a constraint optimisation task solved in the Lagrangian framework. The state convergence procedure is implicitly expressed by the constraint satisfaction mechanism and does not require a separate iterative phase for each epoch of the learning procedure. In fact, the computational structure is based on the search for saddle points of the Lagrangian in the adjoint space composed of weights, neural outputs (node states), and Lagrange multipliers. The proposed approach is compared experimentally with other popular models for processing graphs.
Recommended citation:
@inproceedings{DBLP:conf/ecai/TiezziMMMG20,
author = {Matteo Tiezzi and
Giuseppe Marra and
Stefano Melacci and
Marco Maggini and
Marco Gori},
title = {A Lagrangian Approach to Information Propagation in Graph Neural Networks},
booktitle = {ECAI 2020 - 24th European Conference on Artificial Intelligence},
series = {Frontiers in Artificial Intelligence and Applications},
volume = {325},
pages = {1539--1546},
publisher = {IOS Press},
year = {2020},
doi = {10.3233/FAIA200262},
}
Recommend
-
23
Graph Neural Networks: An overview Over the past decade, we’ve seen that Neural Networks can perform tremendously well in structured data like images and text. Most of the popular models like convolutional netw...
-
10
Engineer friends often ask me: Graph Deep Learning sounds great, but are there any big commercial success stories? Is it being deployed in practical applications? Besides the obvious ones–recommendation systems...
-
36
Graph4NLP Graph4NLP is an easy-to-use library for R&D at the intersection of Deep Learning on Graphs and Natural Language Processing (i.e., DLG4NLP). It provides both
-
12
How to tap into India’s exploding gaming marketLearn how developers and studios of every size can successfully grow their titles in one of the fastest-growing markets in the world.
-
11
A Gentle Introduction to Graph Neural NetworksA Gentle Introduction to Graph Neural NetworksA Gentle Introduction to Graph Neural Networks Neural networks have been adapted to leverage the structure and properties of graphs. We exp...
-
12
GNNs Recipe Graph neural networks (GNNs) are rapidly advancing progress in ML for complex graph data applications. I've composed this concise recipe dedicated to students who are lookin to learn and keep up-to-date with GNNs. It's no...
-
8
LinkedIn creates PASS to tailor graph neural networks for social media Image Credit: TechTalks We are excited to bring Transform...
-
11
Deep Constraint-based Propagation in Graph Neural NetworksPublished in TPAMI, 2021Recommended citation: Matteo Tiezzi, Giuseppe Marra, Stefano Melacci, Marco Maggini (2021). "Deep Constraint-based Propagation in Grap...
-
6
An overview of Graph Neural Networks up to Lagrangian Propagation GNNsDate: December 04, 2020Several real-world applications are characterized by data that exhibit a complex s...
-
11
Abstract + Introduction GNNs 大都遵循一个递归邻居聚合的方法,经过 k 次迭代聚合,一个节点所表征的特征向量能够捕捉到距离其 k-hop 邻域的邻居节点的特征,然后还可以通过 pooling 获取到整个图的表征(比如将所有节点的表...
About Joyk
Aggregate valuable and interesting links.
Joyk means Joy of geeK