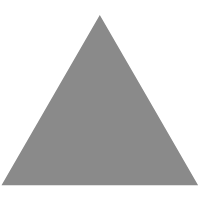
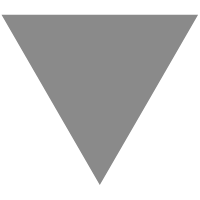
horovod 实现分析
source link: https://ggaaooppeenngg.github.io/zh-CN/2019/08/30/horovod-%E5%AE%9E%E7%8E%B0%E5%88%86%E6%9E%90/
Go to the source link to view the article. You can view the picture content, updated content and better typesetting reading experience. If the link is broken, please click the button below to view the snapshot at that time.
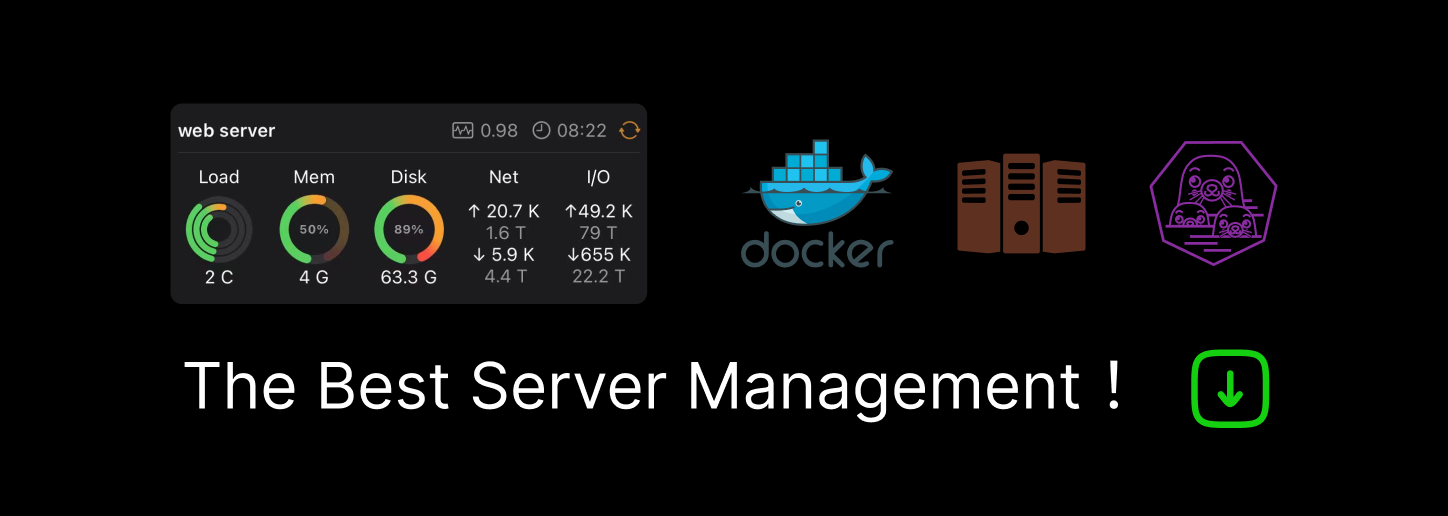
horovod 实现分析
Horovod 是一个兼容主流计算框架的分布式机器学习训练框架,主要基于的算法是 AllReduce,这个是 baidu-research 在17年做的一个实现,这个东西原来是高性能计算范畴里的东西应用了 MPI 并行计算接口来实现,这是并行计算里的一个框架,已经很老了,这里有一个介绍 MPI 的 tutorial 写的比较好。
在介绍 horovod 的之前需要解释一下 AllReduce。在 MapReduce 里面 reduce 被翻译成了规约,在上面提到的 MPI tutorial 里面的解释是
Reduce is a classic concept from functional programming. Data reduction involves reducing a set of numbers into a smaller set of numbers via a function. For example, let’s say we have a list of numbers
[1, 2, 3, 4, 5]
. Reducing this list of numbers with the sum function would producesum([1, 2, 3, 4, 5]) = 15
. Similarly, the multiplication reduction would yieldmultiply([1, 2, 3, 4, 5]) = 120
.
就是说把一个大的集合“缩减”成了小的集合,这里要注意的是这种缩减的计算是要满足交换律的,也就是减法或者除法是不行的,因为在并行计算当中不太好去控制计算的顺序。Reduce 就是这个意思,具体到 MPI_Reduce 就是把不同节点的数字“缩减”到一个节点上,支持的计算方式有加法乘法和取大小值等。
教程中给出的 Reduce 是求和。
AllReduce 就是在每个节点都获得 Reduce 的结果
基于这个标准就有很多的 All-Reduce 的实现,比如 Ring-Reduce,这个实现分两部分,一部分是 Scatter-Reduce 另一部分是 All-Gather。最早是在这篇 post里提到的。这个算法的好处是可以摆脱之前 PS 非常依赖 Parameter-Server 的带宽,Parameter-Server 的带宽会成为计算瓶颈的问题,而 AllReduce 可以让每个节点在带宽传输中的位置是对等的,并且减少传输次数。具体的算法可以看文章的解释,scatter-reduce 就是让每个节点有 K/N 的一个 reduce(也就是 sum),然后把自己的一个 K/N 的 reduce 再传递给其他节点,每个节点只和自己相邻的节点通信。
In the system we described, each of the N GPUs will send and receive values N-1 times for the scatter-reduce, and N-1 times for the allgather. Each time, the GPUs will send K / N values, where K is the total number of values in array being summed across the different GPUs. Therefore, the total amount of data transferred to and from every GPU is
Data Transferred=2(N−1)KN
数据传输量在 N 比较大的时候越没有影响,这就消弭了多节点给 Parameter-Server 造成的瓶颈。
还有一些其他术语,假设有 4 台 4 卡的 GPU 服务器。size 是工作进程(GPU)的数量(6),rank 是所有工作进程的 id(0-15),local rank 是当前服务器上的 id(0-3)。
Horovod 的介绍
使用 horovod 有一定的侵入性,代码需要一定的修改才能变成适配分布式训练,但是有一个好处就是适配的成本不高,并且 horovod 提供的各种框架的支持可以让 horovod 比较好的在各个框架的基础上使用,他支持 tensorflow/keras/mxnet/pytorch,MPI 的实现也有很多,比如 OpenMPI 还有 Nvidia 的 NCCL,还有 facebook 的 gloo,他们都实现了一种并行计算的通信和计算方式。而且 horovod 的本身的实现也很简单。
以 Keras 用 ResNet50 训练 ImageNet 为例,主要侵入了几部分 hvd.init()
这个是 MPI 的初始化,让并行进程能够知道自己的 rank/local_rank 等信息。
第二部根据 local_rank(相当于单节点上的第n张卡),并且设置不占用全部显存,按需分配(可能因内没有统一管理导致显存碎片),然后传递给 keras 设置 session。
# Horovod: pin GPU to be used to process local rank (one GPU per process)
config = tf.ConfigProto()
config.gpu_options.allow_growth = True
config.gpu_options.visible_device_list = str(hvd.local_rank())
K.set_session(tf.Session(config=config))
然后在 rank 0 上恢复一个 checkpoint 并且广播给其他节点,这里的 broadcast 后面会介绍。
# If set > 0, will resume training from a given checkpoint.
resume_from_epoch = 0
for try_epoch in range(args.epochs, 0, -1):
if os.path.exists(args.checkpoint_format.format(epoch=try_epoch)):
resume_from_epoch = try_epoch
break
# Horovod: broadcast resume_from_epoch from rank 0 (which will have
# checkpoints) to other ranks.
resume_from_epoch = hvd.broadcast(resume_from_epoch, 0, name='resume_from_epoch')
# Horovod: print logs on the first worker.
verbose = 1 if hvd.rank() == 0 else 0
设定传输的压缩函数,具体的压缩后面会提到,然后要么从之前的模型恢复要么重新训练。关键的 wrapper 在 opt
上,会给本地的 opt
包装一个 DistributedOptimizer
。
# Horovod: (optional) compression algorithm.
compression = hvd.Compression.fp16 if args.fp16_allreduce else hvd.Compression.none
# Restore from a previous checkpoint, if initial_epoch is specified.
# Horovod: restore on the first worker which will broadcast both model and optimizer weights
# to other workers.
if resume_from_epoch > 0 and hvd.rank() == 0:
model = hvd.load_model(args.checkpoint_format.format(epoch=resume_from_epoch),
compression=compression)
else:
# ResNet-50 model that is included with Keras is optimized for inference.
# Add L2 weight decay & adjust BN settings.
model_config = model.get_config()
for layer, layer_config in zip(model.layers, model_config['layers']):
if hasattr(layer, 'kernel_regularizer'):
regularizer = keras.regularizers.l2(args.wd)
layer_config['config']['kernel_regularizer'] = \
{'class_name': regularizer.__class__.__name__,
'config': regularizer.get_config()}
if type(layer) == keras.layers.BatchNormalization:
layer_config['config']['momentum'] = 0.9
layer_config['config']['epsilon'] = 1e-5
model = keras.models.Model.from_config(model_config)
# Horovod: adjust learning rate based on number of GPUs.
opt = keras.optimizers.SGD(lr=args.base_lr * hvd.size(),
momentum=args.momentum)
# Horovod: add Horovod Distributed Optimizer.
opt = hvd.DistributedOptimizer(opt, compression=compression)
model.compile(loss=keras.losses.categorical_crossentropy,
optimizer=opt,
metrics=['accuracy', 'top_k_categorical_accuracy'])
然后设置一些回调函数,hvd.callbacks.BroadcastGlobalVariablesCallback(0)
保证的是 rank 0 上的所有参数只在 rank 0 初始化,然后广播给其他节点,后面是学习率 decay 的设置和一些统计信息的回调打印。
callbacks = [
# Horovod: broadcast initial variable states from rank 0 to all other processes.
# This is necessary to ensure consistent initialization of all workers when
# training is started with random weights or restored from a checkpoint.
hvd.callbacks.BroadcastGlobalVariablesCallback(0),
# Horovod: average metrics among workers at the end of every epoch.
#
# Note: This callback must be in the list before the ReduceLROnPlateau,
# TensorBoard, or other metrics-based callbacks.
hvd.callbacks.MetricAverageCallback(),
# Horovod: using `lr = 1.0 * hvd.size()` from the very beginning leads to worse final
# accuracy. Scale the learning rate `lr = 1.0` ---> `lr = 1.0 * hvd.size()` during
# the first five epochs. See https://arxiv.org/abs/1706.02677 for details.
hvd.callbacks.LearningRateWarmupCallback(warmup_epochs=args.warmup_epochs, verbose=verbose),
# Horovod: after the warmup reduce learning rate by 10 on the 30th, 60th and 80th epochs.
hvd.callbacks.LearningRateScheduleCallback(start_epoch=args.warmup_epochs, end_epoch=30, multiplier=1.),
hvd.callbacks.LearningRateScheduleCallback(start_epoch=30, end_epoch=60, multiplier=1e-1),
hvd.callbacks.LearningRateScheduleCallback(start_epoch=60, end_epoch=80, multiplier=1e-2),
hvd.callbacks.LearningRateScheduleCallback(start_epoch=80, multiplier=1e-3),
]
最后直接用 allreduce 计算一个 evaluation score。
# Evaluate the model on the full data set.
score = hvd.allreduce(model.evaluate_generator(input_fn(False, args.train_dir, args.val_batch_size),NUM_IMAGES['validation']))
适配层和压缩算法
horovod 的实现主要分几部分,第一部分是一个适配层,用于兼容各种框架,比如 tensorflow 的适配就是实现一个新的 Op,这个可以参考 add new op,里面规范了 Tensorflow 自定义算子的实现。
请注意,生成的函数将获得一个蛇形名称(以符合 PEP8)。因此,如果您的操作在 C++ 文件中命名为 ZeroOut,则 Python 函数将称为 zero_out。
C++ 的定义是驼峰的,生成出来的 python 函数是下划线小写的,所以最后对应的是,适配Op的代码在 horovod/tensorflow 目录下面
C++ | Python |
---|---|
HorovodAllgather | horovod_allgather |
HorovodAllreduce | horovod_allreduce |
HorovodBroadcast | horovod_broadcast |
另外在适配层可以加入一些压缩算法(在 horovod/[framework]/compression.py
),我觉得压缩算法和框架无关的,放到适配层下面可能有别的原因,比如 tensorflow 默认带了一个 float16 压缩,具体的其他压缩算法比如3LC,可以通过有损压缩或者无损压缩提高带宽利用率。
这一层的实现是统一的,所有的适配层最后都是发出一些 Op+Tensor 的 Message 到队列中,后台初始化的时候会有一个专门的线程专门消费这个队列。他有一个同步消息的过程,相当于这个 tensor 在所有节点上都就绪以后就可以开始计算了,主体的流程是:
// The coordinator currently follows a master-worker paradigm. Rank zero acts
// as the master (the "coordinator"), whereas all other ranks are simply
// workers. Each rank runs its own background thread which progresses in ticks.
// In each tick, the following actions happen:
//
// a) The workers send a Request to the coordinator, indicating what
// they would like to do (which tensor they would like to gather and
// reduce, as well as their shape and type). They repeat this for every
// tensor that they would like to operate on.
//
// b) The workers send an empty "DONE" message to the coordinator to
// indicate that there are no more tensors they wish to operate on.
//
// c) The coordinator receives the Requests from the workers, as well
// as from its own TensorFlow ops, and stores them in a [request table]. The
// coordinator continues to receive Request messages until it has
// received MPI_SIZE number of empty "DONE" messages.
//
// d) The coordinator finds all tensors that are ready to be reduced,
// gathered, or all operations that result in an error. For each of those,
// it sends a Response to all the workers. When no more Responses
// are available, it sends a "DONE" response to the workers. If the process
// is being shutdown, it instead sends a "SHUTDOWN" response.
//
// e) The workers listen for Response messages, processing each one by
// doing the required reduce or gather, until they receive a "DONE"
// response from the coordinator. At that point, the tick ends.
// If instead of "DONE" they receive "SHUTDOWN", they exit their background
// loop.
简单来讲就是说 coordinator 集 size 个 request DONE,然后找出就绪的 tensor (在 message_table 里面查找)构造出一个 read_to_reduce 的列表,然后发出 size 个 request 告知进程进行计算,然后 worker 接受到 response 开始真正的计算过程(通过 op_manager 具体执行)。
这是整体同步的过程,如果打开 horovod 的 trace log(HOROVOD_LOG_LEVEL=trace
) 就能看到同步的过程。horovod 的主要 Op 除了 AllReduce 之外还有 allgather 和 broadcast。
算子实现层
具体的 op 在 common/op 可以看到有 NCCL/Gloo/MPI 等等的,这些由 op_manager 管理,他会根据优先级找到可以用来计算的 op 进行计算,比如 MPI 用的就是 MPI_Allreduce,具体 scatter-gather 和 all-gather openMPI 有现成的实现,NCCL 就直接调用 ncclAllReduce
,比较新的 nccl 也支持跨节点的 allreduce 了,不用自己再套一层。
除了 allreduce 之外,还有两个比较重要的算子。
allgather 主要是比 allreduce 少一层 reduce,所有数据被发送到所有进程就可以。allreduce 的第二步就是把每个进程的 scatter-reduce 的 reduce 结果发送到所有进程。
broadcast 的作用是一对多的广播,主要是把初始化的参数同步给其他进程的时候使用。
Recommend
About Joyk
Aggregate valuable and interesting links.
Joyk means Joy of geeK