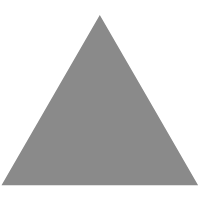
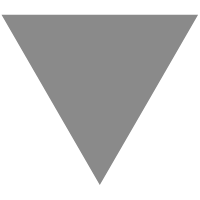
GitHub - bloomberg/scatteract: Project which implements extraction of data from...
source link: https://github.com/bloomberg/scatteract
Go to the source link to view the article. You can view the picture content, updated content and better typesetting reading experience. If the link is broken, please click the button below to view the snapshot at that time.
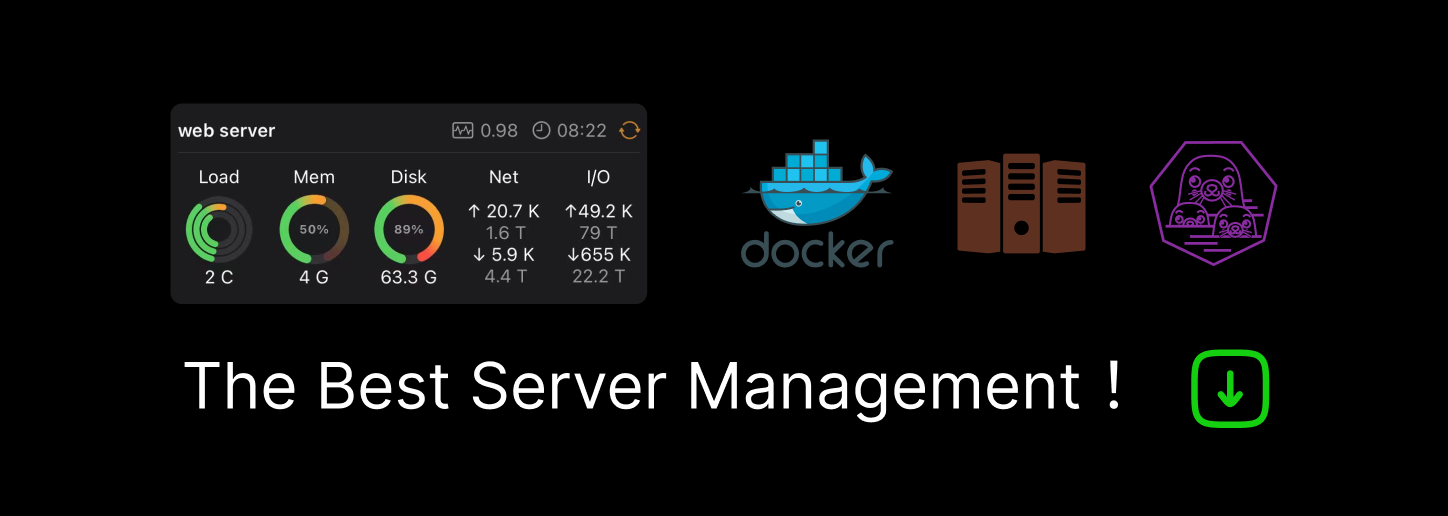
Scatteract
Scatteract is a framework to automatically extract data from the image of scatter plots. We use TensorBox1 to detect the relevant objects (points, tick marks and tick values), Tesseract for the OCR, and several heuristics to extract the points in chart coordinates. See the paper for details. This repository is meant to support the paper and is not an attempt at creating an actual open source project.
1Disclaimer: We vendored the TensorBox dependency into the tensorbox
folder since there is no way to pull it in from pypi. We have made no change to this code.
Requirements:
tensorflow==0.10.0rc0
scipy (tested on 0.17.1)
scikit-learn (tested on 0.17.1)
pandas (tested on 0.18.1)
Pillow (PIL) (tested on 3.2.0)
numpy (tested on 1.10.4)
opencv-python (cv2) (tested on 2.4.10)
matplotlib (tested on 1.5.1)
runcython (tested on 0.25)
pyocr (tested on 0.4.6)
tesseract-ocr (tested on 3.02)
On Python 2.7+, the following compatibility module is also required:
backports.functools_lru_cache
How to use:
Generate training and testing data
$ python generate_random_scatter.py --directory plots_v1 --n_train 25000 --n_test 500
Train object detection models
Note that we are using an older version of TensorBox which crashes once training is done because of threading issues, nevertheless the trained models are successfully saved.
& cd tensorbox/utils/ && make && cd ../..
$ python tensorbox/train_obj_model.py --hypes hypes/lstm_rezoom_plot_points.json --gpu 0 --logdir output --name points_v1
$ python tensorbox/train_obj_model.py --hypes hypes/lstm_rezoom_plot_ticks.json --gpu 0 --logdir output --name ticks_v1
$ python tensorbox/train_obj_model.py --hypes hypes/lstm_rezoom_plot_labels.json --gpu 0 --logdir output --name labels_v1
IPython Notebook
The ipython notebook can be used after the object detection models are trained. It allows you to interact with the building blocks of Scatteract and visualize their outputs.
Test object detection models
On the randomly generated test set:
$ chmod +x tensorbox -R
$ python test_obj_model.py --model_dir output/points_v1 --iteration 125000 --image_dir data/plots_v1 --true_idl data/plots_v1/test_points.idl
$ python test_obj_model.py --model_dir output/ticks_v1 --iteration 125000 --image_dir data/plots_v1 --true_idl data/plots_v1/test_ticks.idl
$ python test_obj_model.py --model_dir output/labels_v1 --iteration 125000 --image_dir data/plots_v1 --true_idl data/plots_v1/test_labels.idl
On a currated randomly generated test set:
$ chmod +x tensorbox -R
$ python test_obj_model.py --model_dir output/points_v1 --iteration 125000 --image_dir data/plot_test --true_idl data/plot_test/points.idl
$ python test_obj_model.py --model_dir output/ticks_v1 --iteration 125000 --image_dir data/plot_test --true_idl data/plot_test/ticks.idl
$ python test_obj_model.py --model_dir output/labels_v1 --iteration 125000 --image_dir data/plot_test --true_idl data/plot_test/labels.idl
Test OCR
On the randomly generated test set:
$ python generate_test_tesseract.py --image_dir data/plots_v1 --label_values_idl data/plots_v1/test_label_values.idl
$ python tesseract.py --tsv_truth data/plots_v1/label_image_values.tsv --image_dir data/plots_v1/label_images
On a currated randomly generated test set:
$ python tesseract.py --tsv_truth data/plot_test/label_image_values.tsv --image_dir data/plot_test/label_images
Test end-to-end system
On a currated randomly generated test set:
& python scatter_extract.py --model_dict '{"ticks":"./output/ticks_v1", "labels":"./output/labels_v1","points":"./output/points_v1"}' \
--true_idl_dict '{"ticks":"./data/plot_test/ticks.idl","labels":"./data/plot_test/labels.idl", "points":"./data/plot_test/points.idl"}' \
--image_output_dir image_output --csv_output_dir csv_output --true_coord_idl ./data/plot_test/coords.idl \
--iteration 125000 --image_dir data/plot_test/
Predict with end-to-end system (i.e. extract data points from plots with no ground truth)
On a set of plots scrapped from the web:
$ python scatter_extract.py --model_dict '{"ticks":"./output/ticks_v1", "labels":"./output/labels_v1","points":"./output/points_v1"}' \
--iteration 125000 --image_dir data/plot_real/ --predict_idl ./data/plot_real/test_real.idl --image_output_dir image_output --csv_output_dir csv_output
Recommend
-
100
ea-async - EA Async implements async-await methods in the JVM.
-
34
README.md
-
54
README.md attention some attention implements 《Attention is All You Need》中的Attention机制的实现
-
25
When not blogging about data science, I work as a Senior Data Scientist at an e-commerce company called ShopRunner. Over the past year, our team has been building out large multi-task deep learning ensembles to predict r...
-
5
TRex TRex makes OCR easy and accessible on a Mac. But what is OCR anyway? Imagine you have a PDF file or a Web page where you can't...
-
7
WikipediaQL: querying structured data from Wikipedia WikipediaQL is an experimental query language and Python library for querying structured data from Wikipedia. It looks like this: from wikip...
-
5
bloomberg/setuptools: Official project repository for the Setuptools build system This branch is 6646 commits behind pypa/setuptools:main. Contribute...
-
12
What is this repository? This repository is a fork of Project Calico's felix repository, created so that Bloomberg's engineering team can collaborate with each other (and the rest of the Project Calico community) on enha...
-
3
wsk.example A sample starter project using wsk. View at: https://bloomberg.github.io/wsk.example ...
-
5
HTML table data extractor for Go htmltable enables structured data extraction from HTML tables and URLs and requires almost no external dependencies. Tested with Go 1.18.x and 1.19.x. Installation go g...
About Joyk
Aggregate valuable and interesting links.
Joyk means Joy of geeK