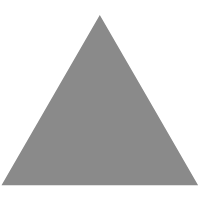
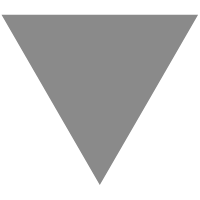
GitHub - bloomberg/fast-noise-aware-topic-clustering: Research code and scripts...
source link: https://github.com/bloomberg/fast-noise-aware-topic-clustering
Go to the source link to view the article. You can view the picture content, updated content and better typesetting reading experience. If the link is broken, please click the button below to view the snapshot at that time.
FANATIC: FAst Noise-Aware TopIc Clustering
Authors: Ari Silburt, Anja Subasic, Evan Thompson, Carmeline Dsilva, Tarec Fares
General
This repo contains the research code and scripts used in the Silburt et al. (2021) paper "FANATIC: FAst Noise-Aware TopIc Clustering", and provides a basic overview of the code structure and its major components. For more questions, please directly contact the authors.
In particular, this repo allows a user to:
- Download the reddit data
- Train a word2vec embedding model
- Use
FANATIC
to cluster the reddit data and dump results for downstream analysis.
Note that the original paper results used an in-house preprocessor, but a very similar open-source one has been provided (see fanatic/preprocess/nltk_preprocessor.py
).
License
Please read the LICENSE
.
How-to
Setup
It is recommended to create a fresh python virual environment and run the following commands from the base repo:
pip install --upgrade pip
pip install -r requirements.txt
And then in a python shell do:
import nltk nltk.download('stopwords')
This repo has been tested against python3.7
.
Download the Reddit Data
Data can be downloaded from pushshift using wget
, e.g. wget https://files.pushshift.io/reddit/submissions/RS_2017-11.zst
. If data files are downloaded to the data/
directory, subsequent scripts are already set up to look there.
Training a Word2vec Embedding
A new word2vec model can be trained using embedding_driver.py
, and it is recommended to carefully inspect the arguments before running. In particular, a Reddit data file(s) must first be downloaded and specified in the --data-files
argument.
Cluster via FANATIC
Once the data has been downloaded and word2vec model trained, a clustering run can be performed using the clustering_driver.py
script. All input arguments are specified in the parse_args
function of fanatic/arguments.py
including data, label, preprocessing, clustering algorithm and output arguments.
See the Silburt et al. (2021) paper for a detailed explanation of FANATIC's hyperparameters.
Clustering labels
By default, the data is clustered against the data/subreddit_labels.json
labels file, which indicates:
- what subreddits are considered for clustering (all other subreddits are discarded).
- whether the subreddit is a "coherent" or "noise" topic, where all noise topics are assigned the same
NOISE
label.
The --subreddit-noise-percentage
argument sets the fraction of documents that come from noise subreddits. In the case where --num-docs-read
and --subreddit-noise-percentage
are incompatible with each other, honouring --subreddit-noise-percentage
is prioritized. If --subreddit-noise-percentage
is set to None
, the noise percentage is set by the natural data distribution.
The data/subreddit_labels.json
labels file can be substituted for a different one, or ignored entirely by setting --subreddit-labels-file None
. When --subreddit-labels-file
is set to None
all encountered subreddits are used, each subreddit becomes its own coherent topic, and the concept of "topic noise" disappears. Thus, the --subreddit-noise-percentage
argument becomes irrelevant.
Clustering Outputs
After a successful clustering run, the files that are output are:
fanatic_<dataset-id>_<seed-run>_labels_and_assignments.json
: this file is generated for each seed run and contains, for each document-id, the assignment (what cluster the document ended up in) and label (the label associated with the document). Therefore, the full clustering result is contained within this file for downstream analysis. Document-ids can be mapped back to the original datafile should additional metadata be desired.fanatic_<dataset-id>_<seed-run>_sample_clusters.txt
- this file is generated for each seed run and contains the first 10 documents from each cluster and associated label. Format is<text> -> <label>
. This gives the user a qualitative sense of what each cluster contains.fanatic_<dataset-id>_<seed-run>_summary.txt
- this file is generated for each seed run and contains all input parameters and clustering stats/metrics. It is effectively a summary of the entire clustering run, allowing you to quickly parse results and/or recreate the job if needed. It can be consumed by configparser.fanatic_<dataset-id>_summary_averaged.txt
- this file is generated once for a dataset-id and contains the input arguments and averaged clustering stats/metrics across the seed runs.
In addition, the full clustering model can be dumped via pickle for deeper investigation by adding --flag-save-clusteringmodel
. Warning: this file can become large, especially for big datasets.
Custom Preprocessor / Featurizer
Results from the paper were generated using an in-house preprocessor that is not available to the public. Using nltk we created a very similar preprocessor, located at fanatic/preprocess/nltk_preprocessor.py
, and inherits from fanatic/preprocess/generic_preprocessor.py
. Users are free to create their own custom preprocessors that also inherit from generic_preprocessor.py
and experiment with more sophisticated features (e.g. BERT embeddings). See generic_preprocessor.py
for additional documentation and requirements.
Non-Reddit Datasets
Users are encouraged to substitute or modify fanatic/preprocess/read_data.py
to read in different kinds of data. In particular, DATASET_INPUT_FIELD
, DATASET_LABEL_FIELD
and DATASET_ID_FIELD
must be changed to extract the relevant content from the new dataset for downstream preprocessing and clustering.
Tests
Some basic unit tests can be run from the home directory with python3.7 -m pytest tests/unit/
Recommend
About Joyk
Aggregate valuable and interesting links.
Joyk means Joy of geeK