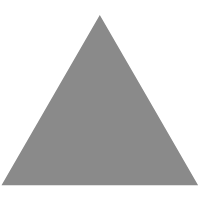
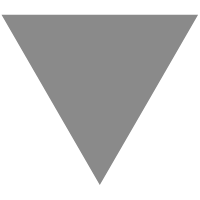
PostGIS vs GPU: Performance and Spatial Joins
source link: https://blog.crunchydata.com/blog/performance-and-spatial-joins
Go to the source link to view the article. You can view the picture content, updated content and better typesetting reading experience. If the link is broken, please click the button below to view the snapshot at that time.
Every once in a while, a post shows up online about someone using GPUs for common spatial analysis tasks, and I get a burst of techno-enthusiasm. Maybe this is truly the new way!
This week it was a post on GPU-assisted spatial joins that caught my eye. In summary, the author took a 9M record set of parking infractions data from Philadelphia and joined it to a 150 record set of Philadelphia neighborhoods. The process involved building up a little execution engine in Python. It was pretty manual but certainly fast.
I wondered: how bad would execution on a conventional environment like PostGIS be, in comparison?
Follow along, if you like!
Server Setup
I grabbed an 8-core cloud server with PostgreSQL 14 and PostGIS 3 from Crunchy Bridge DBaaS, like so:
Data Download
Then I pulled down the data.
#
# Download Philadelphia parking infraction data
#
curl "https://phl.carto.com/api/v2/sql?filename=parking_violations&format=csv&skipfields=cartodb_id,the_geom,the_geom_webmercator&q=SELECT%20*%20FROM%20parking_violations%20WHERE%20issue_datetime%20%3E=%20%272012-01-01%27%20AND%20issue_datetime%20%3C%20%272017-12-31%27" > phl_parking.csv
#
# Download Philadelphia neighborhoods
#
wget https://github.com/azavea/geo-data/raw/master/Neighborhoods_Philadelphia/Neighborhoods_Philadelphia.zip
#
# Convert neighborhoods file to SQL
#
unzip Neighborhoods_Philadelphia.zip
shp2pgsql -s 102729 -D Neighborhoods_Philadelphia phl_hoods > phl_hoods.sql
# Connect to database
psql -d postgres
Data Loading and Preparation
Then I loaded the CSV and SQL data files into the database.
-- Set up PostGIS
CREATE EXTENSION postgis;
-- Read in the neighborhoods SQL file
\i phl_hoods.sql
-- Reproject the neighborhoods to EPSG:4326 to match the
-- parking data
ALTER TABLE phl_hoods
ALTER COLUMN geom
TYPE Geometry(multipolygon, 4326)
USING st_transform(geom, 4326);
-- Create parking infractions table
CREATE TABLE phl_parking (
anon_ticket_number integer,
issue_datetime timestamptz,
state text,
anon_plate_id integer,
division text,
location text,
violation_desc text,
fine float8,
issuing_agency text,
lat float8,
lon float8,
gps boolean,
zip_code text
);
-- Read in the parking data
\copy phl_parking FROM 'phl_parking.csv' WITH (FORMAT csv, HEADER true);
This is the first step that takes any time. Reading in the 9M records from CSV takes about 29 seconds.
Because the parking data lacks a geometry column, I create a second table that does have a geometry column, and then index that.
CREATE TABLE phl_parking_geom AS
SELECT anon_ticket_number,
ST_SetSRID(ST_MakePoint(lon, lat), 4326) AS geom
FROM phl_parking ;
ANALYZE phl_parking_geom;
Making a second copy while creating a geometry column takes about 24 seconds.
Finally, to carry out a spatial join, I will need a spatial index on the parking points. In this case I follow my advice about when to use different spatial indexes and build a "spgist" index on the geometry.
CREATE INDEX phl_parking_geom_spgist_x
ON phl_parking_geom USING spgist (geom);
This is the longest process, and takes about 60 seconds.
Running the Query
The spatial join query is a simple inner join using ST_Intersects as the join condition.
SELECT h.name, count(*)
FROM phl_hoods h
JOIN phl_parking_geom p
ON ST_Intersects(h.geom, p.geom)
GROUP BY h.name;
Before running it, though, I took a look at the EXPLAIN
output for the query, which is this.
HashAggregate (cost=4031774.83..4031776.41 rows=158 width=20)
Group Key: h.name
-> Nested Loop (cost=0.42..4024339.19 rows=1487128 width=12)
-> Seq Scan on phl_hoods h (cost=0.00..30.58 rows=158 width=44)
-> Index Scan using phl_parking_geom_spgist_x on phl_parking_geom p
(cost=0.42..25460.90 rows=941 width=32)
Index Cond: (geom && h.geom)
Filter: st_intersects(h.geom, geom)
This is all very good, a nested loop on the smaller neighborhood table against the large indexed parking table, except for one thing: I spun up an 8 core server and my plan has no parallelism!
What is up?
SHOW max_worker_processes; -- 8
SHOW max_parallel_workers; -- 8
SHOW max_parallel_workers_per_gather; -- 2
SHOW min_parallel_table_scan_size; -- 8MB
Aha! That minimum table size for parallel scans seems large compared to our neighborhoods table.
philly=# select pg_relation_size('phl_hoods');
-- 237568
Yep! So, first we will set the min_parallel_table_scan_size
to 1kb, and then increase the max_parallel_workers_per_gather
to 8 and see what happens.
SET max_parallel_workers_per_gather = 8;
SET min_parallel_table_scan_size = '1kB';
The EXPLAIN
output for the spatial join now parallelized, but unfortunately only to 4 workers.
Finalize GroupAggregate (cost=1319424.81..1319505.22 rows=158 width=20)
Group Key: h.name
-> Gather Merge (cost=1319424.81..1319500.48 rows=632 width=20)
Workers Planned: 4
-> Sort (cost=1318424.75..1318425.14 rows=158 width=20)
Sort Key: h.name
-> Partial HashAggregate
(cost=1318417.40..1318418.98 rows=158 width=20)
Group Key: h.name
-> Nested Loop
(cost=0.42..1018842.71 rows=59914937 width=12)
-> Parallel Seq Scan on phl_hoods h
(cost=0.00..29.39 rows=40 width=1548)
-> Index Scan using phl_parking_geom_spgist_x
on phl_parking_geom p
(cost=0.42..25460.92 rows=941 width=32)
Index Cond: (geom && h.geom)
Filter: st_intersects(h.geom, geom)
Now we still get a nested loop join on neighborhoods, but this time the planner recognizes that it can scan the table in parallel. Since the spatial join is fundamentally CPU bound and not I/O bound this is a much better plan choice.
SELECT h.name, count(*)
FROM phl_hoods h
JOIN phl_parking_geom p
ON ST_Intersects(h.geom, p.geom)
GROUP BY h.name;
The final query running with 4 workers takes 24 seconds to execute the join of the 9 million parking infractions with the 150 neighborhoods.
This is pretty good!
Is it faster than the GPU though? No, the GPU post says his custom python/GPU solution took just 8 seconds to execute. Still, the differences in environment are important:
- The data in PostgreSQL is fully editable in place, by multiple users and applications.
- The execution plan in PostgreSQL is automatically optimized. If the next query involves neighborhood names and plate numbers, it will be just as fast and involve no extra custom code.
- The PostGIS engine is capable of answering 100s of other spatial questions about the database.
- With a web service like pg_tileserv or pg_featureserv, the data are immediately publishable and remote queryable.
Conclusion
Basically, it is very hard to beat a bespoke performance solution with a general purpose tool. Yet, PostgreSQL/PostGIS comes within "good enough" range of a high end GPU solution, so that counts as a "win" to me.
Recommend
About Joyk
Aggregate valuable and interesting links.
Joyk means Joy of geeK