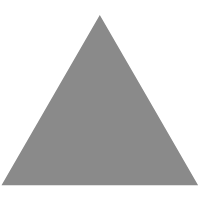
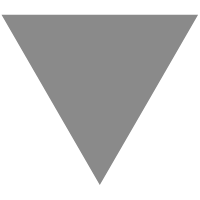
[1712.07495] A Distributed Frank-Wolfe Framework for Learning Low-Rank Matrices...
source link: https://arxiv.org/abs/1712.07495
Go to the source link to view the article. You can view the picture content, updated content and better typesetting reading experience. If the link is broken, please click the button below to view the snapshot at that time.
[Submitted on 20 Dec 2017 (v1), last revised 11 May 2018 (this version, v2)]
A Distributed Frank-Wolfe Framework for Learning Low-Rank Matrices with the Trace Norm
We consider the problem of learning a high-dimensional but low-rank matrix from a large-scale dataset distributed over several machines, where low-rankness is enforced by a convex trace norm constraint. We propose DFW-Trace, a distributed Frank-Wolfe algorithm which leverages the low-rank structure of its updates to achieve efficiency in time, memory and communication usage. The step at the heart of DFW-Trace is solved approximately using a distributed version of the power method. We provide a theoretical analysis of the convergence of DFW-Trace, showing that we can ensure sublinear convergence in expectation to an optimal solution with few power iterations per epoch. We implement DFW-Trace in the Apache Spark distributed programming framework and validate the usefulness of our approach on synthetic and real data, including the ImageNet dataset with high-dimensional features extracted from a deep neural network.
Recommend
-
47
README.md 概述 图是表达能力很强的通用数据结构,可以用来刻画现实世界中的很多问题。图神经网络等基于图的学习方法在很多领域取得了非常好的效果。 Eule...
-
33
README.md KungFu Easy, adaptive and fast distributed machine learning.
-
31
Acme: A research framework for reinforcement learning ||
-
2
Mike Wolfe @nolongerset Advanced techniques and unique perspectives...
-
1
Urbit with Galen Wolfe-Pauly By SE Daily Podcast Friday, December 17 2021
-
1
Distributed Frank-Wolfe Framework for Trace Norm Minimization This package solves trace norm minimization in a distributed way. It is written in Python and works on clusters deployed with Apache SPARK. Dependency
-
2
celery基本介绍为什么选择celery? 可以后台运行任务。 在请求完成后,执行后续的任务 通过异步执行和重试保证任务的完成 。。。等
-
8
Gene Wolfe Was Sci-Fi’s Most Enigmatic Writer
-
3
What I’m reading lately: Dogs and Wolfe It’s been a whil...
-
4
Whitney Wolfe Herd is coming to the Code Conference / The founder and CEO of Bumble runs a series of apps about getting people into relationships (and even just friendships).By
About Joyk
Aggregate valuable and interesting links.
Joyk means Joy of geeK