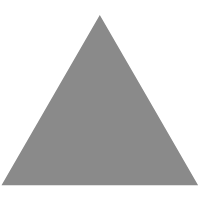
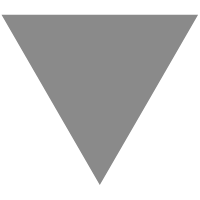
[2004.00412] Total Variation Regularization for Compartmental Epidemic Models wi...
source link: https://arxiv.org/abs/2004.00412
Go to the source link to view the article. You can view the picture content, updated content and better typesetting reading experience. If the link is broken, please click the button below to view the snapshot at that time.
[Submitted on 1 Apr 2020 (v1), last revised 30 May 2020 (this version, v2)]
Total Variation Regularization for Compartmental Epidemic Models with Time-Varying Dynamics
Compartmental epidemic models are among the most popular ones in epidemiology. For the parameters (e.g., the transmission rate) characterizing these models, the majority of researchers simplify them as constants, while some others manage to detect their continuous variations. In this paper, we aim at capturing, on the other hand, discontinuous variations, which better describe the impact of many noteworthy events, such as city lockdowns, the opening of field hospitals, and the mutation of the virus, whose effect should be instant. To achieve this, we balance the model's likelihood by total variation, which regulates the temporal variations of the model parameters. To infer these parameters, instead of using Monte Carlo methods, we design a novel yet straightforward optimization algorithm, dubbed Iterated Nelder--Mead, which repeatedly applies the Nelder--Mead algorithm. Experiments conducted on the simulated data demonstrate that our approach can reproduce these discontinuities and precisely depict the epidemics.
Recommend
About Joyk
Aggregate valuable and interesting links.
Joyk means Joy of geeK