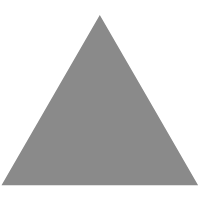
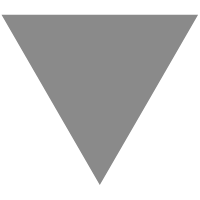
Inferences from logistic regression models in the presence of small samples, rar...
source link: https://www.tandfonline.com/doi/abs/10.1080/02664763.2017.1282441
Go to the source link to view the article. You can view the picture content, updated content and better typesetting reading experience. If the link is broken, please click the button below to view the snapshot at that time.
Inferences from logistic regression models in the presence of small samples, rare events, nonlinearity, and multicollinearity with observational data
The logistic regression model has been widely used in the social and natural sciences and results from studies using this model can have significant policy impacts. Thus, confidence in the reliability of inferences drawn from these models is essential. The robustness of such inferences is dependent on sample size. The purpose of this article is to examine the impact of alternative data sets on the mean estimated bias and efficiency of parameter estimation and inference for the logistic regression model with observational data. A number of simulations are conducted examining the impact of sample size, nonlinear predictors, and multicollinearity on substantive inferences (e.g. odds ratios, marginal effects) when using logistic regression models. Findings suggest that small sample size can negatively affect the quality of parameter estimates and inferences in the presence of rare events, multicollinearity, and nonlinear predictor functions, but marginal effects estimates are relatively more robust to sample size.
Recommend
About Joyk
Aggregate valuable and interesting links.
Joyk means Joy of geeK