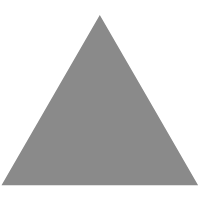
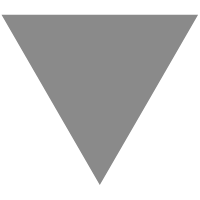
Improving Language Models by Retrieving from Trillions of Tokens
source link: https://deepmind.com/research/publications/2021/improving-language-models-by-retrieving-from-trillions-of-tokens
Go to the source link to view the article. You can view the picture content, updated content and better typesetting reading experience. If the link is broken, please click the button below to view the snapshot at that time.
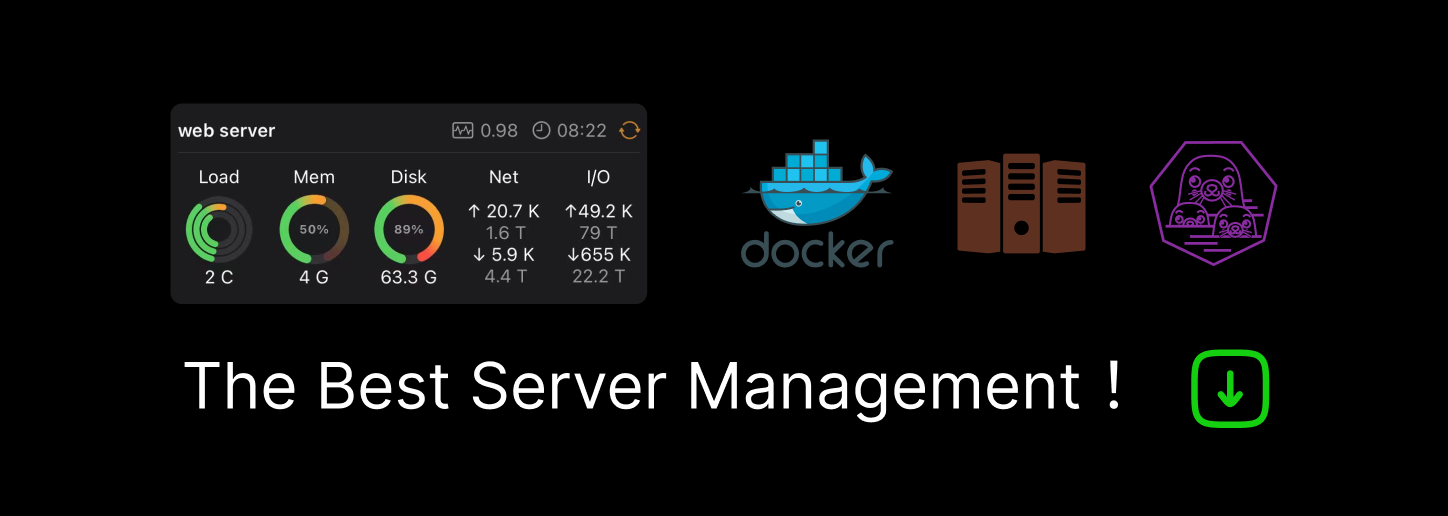
Improving Language Models by Retrieving from Trillions of Tokens
Abstract
We enhance auto-regressive language models by conditioning on document chunks retrieved from a large corpus, based on local similarity with preceding tokens. With a 2 trillion token database, our Retrieval-Enhanced Transformer (RETRO) obtains comparable performance to GPT-3 and Jurassic-1 on the Pile, despite using 25× fewer parameters. After fine-tuning, Retro performance translates to downstream knowledge-intensive tasks such as question answering. Retro combines a frozen Bert retriever, a differentiable encoder and a chunked cross-attention mechanism to predict tokens based on an order of magnitude more data than what is typically consumed during training. We typically train Retro from scratch, yet can also rapidly RETROfit pre-trained transformers with retrieval and still achieve good performance. Our work opens up new avenues for improving language models through explicit memory at unprecedented scale.
Authors' Notes
In recent years, significant performance gains in autoregressive language modeling have been achieved by increasing the number of parameters in Transformer models. This has led to a tremendous increase in training energy cost and resulted in a generation of dense “Large Language Models” (LLMs) with 100+ billion parameters. Simultaneously, large datasets containing trillions of words have been collected to facilitate the training of these LLMs.
We explore an alternate path for improving language models: we augment transformers with retrieval over a database of text passages including web pages, books, news and code. We call our method RETRO, for “Retrieval Enhanced TRansfOrmers”.
In traditional transformer language models, the benefits of model size and data size are linked: as long as the dataset is large enough, language modeling performance is limited by the size of the model. However, with RETRO the model is not limited to the data seen during training– it has access to the entire training dataset through the retrieval mechanism. This results in significant performance gains compared to a standard Transformer with the same number of parameters. We show that language modeling improves continuously as we increase the size of the retrieval database, at least up to 2 trillion tokens – 175 full lifetimes of continuous reading.
For each text passage (approximately a paragraph of a document), a nearest-neighbor search is performed which returns similar sequences found in the training database, and their continuation. These sequences help predict the continuation of the input text. The RETRO architecture interleaves regular self-attention at a document level and cross-attention with retrieved neighbors at a finer passage level. This results in both more accurate and more factual continuations. Furthermore, RETRO increases the interpretability of model predictions, and provides a route for direct interventions through the retrieval database to improve the safety of text continuation. In our experiments on the Pile, a standard language modeling benchmark, a 7.5 billion parameter RETRO model outperforms the 175 billion parameter Jurassic-1 on 10 out of 16 datasets and outperforms the 280B Gopher on 9 out of 16 datasets.
Below, we show two samples from our 7B baseline model and from our 7.5B RETRO model model that highlight how RETRO’s samples are more factual and stay more on topic than the baseline sample.
Related
Blog post
Research
Language modelling at scale
We are releasing three papers on language models, Gopher, ethical considerations, and retrieval.
08 Dec 2021
Featured publication
Ethical and social risks of harm from Language Models
Laura Weidinger, John Mellor, et al. arXiv 2021
Recommend
-
123
-
72
README.md Internal Monologue Attack: Retrieving NTLM Hashes without Touching LSASS Introduction Mimikatz, developed by Benjamin Delpy (@gentilkiwi), is a well-regarded post-exploitatio...
-
58
Commands and functions for retrieving web page content and processing it into and displaying it as Org-mode content. - alphapapa/org-web-tools
-
6
The Four Innovation Phases of Netflix’s Trillions Scale Real-time Data InfrastructureMy name is Zhenzhong Xu. I joined Netflix in 2015 as a founding engineer on the Real-time D...
-
3
To-go coffee cups shed trillions of plastic particles under normal use ...
-
11
Davos FinTech - we lost trillions on crypto, but don’t worry it was unregulated! says WEF By Chris Middleton
-
6
How Discord Stores Trillions of Messages
-
10
El Niños Cause Trillions in Lost Economic...
-
5
Discord Migrates Trillions of Messages from Cassandra to ScyllaDB Jun 22, 2023...
-
4
NASA now believes our galaxy is home to trillions - not billions - of rogue planets Castaways that aren't gravitationally tied to a star system By
About Joyk
Aggregate valuable and interesting links.
Joyk means Joy of geeK