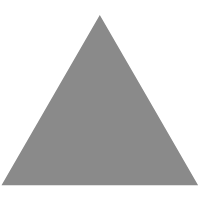
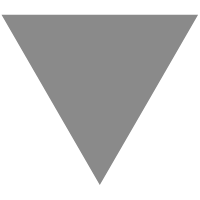
“3 out of 5 users have this issue”
source link: https://uxplanet.org/3-out-of-5-users-have-this-issue-96614be141ce
Go to the source link to view the article. You can view the picture content, updated content and better typesetting reading experience. If the link is broken, please click the button below to view the snapshot at that time.
“3 out of 5 users have this issue”
We talk to 5 users and report that 3 out of 5 have an unmet need. Why shouldn’t UX Researchers report this and what to do instead? How to estimate how many users have a certain problem? You will find here A) a 30-second guide to get confidence intervals and B) an interview technique to get an even better estimation without statistics.
About Zsombor: UX Research Lead at SAP Emarsys. Hiring and mentoring user researchers. Teaching research at his own peril at zombor.io
We know “3 out of 5” is bad, but we can’t resist believing in it
How many users are affected? What’s the Reach? In SAP Emarsys the estimated reach of a feature is one of the key factors when the feature stacks up against other opportunities.
When usability testing we want to know how many users are affected by the issue? We want to rank issues by this.
When interviewing for user needs, we want to find out what portion of our customer base has this and that unmet need. What’s the market size for this feature? Is this going to benefit lots of users or just this one?
UX Researchers can answer these questions. The answer is just not “3 out of 5”.
We all know deep down that “3 out of 5” means nothing. After all, we only talked to 5 people… How could those 5 people characterize the remaining millions?
Yet, we can’t seem to resist using this data point and make prioritization decisions based on it. After all “This is the best data we have. It’s better than nothing.”
Some UX Researchers -feeling the guilt of reporting such a number- slap a warning on the report “Limitation: small sample size.” In my opinion, this is the same as saying “The result is X. Also: I am lying.” What’s the point of reporting the number? At best people ignore this result. At worst, they believe it.
“3 out of 5” is misleading because it gives false confidence in the results
What’s wrong with “3 out of 5”? The problem is not the ratio. The ratio is 60%. And this 60% is truly our best guess (almost) at what the ratio is in the population.
What’s wrong is a false certainty that this number suggests: 60 is 60. It feels solid. It’s higher than 50. It’s even slightly higher than 59.
But when we pick only 5 people of the entire population chances are we pick a special pack of 5 who are not representing the entire user base well (aka Sampling error). And thus if we sampled again and again, the 60% would come out as 40%, or 20% or 90%. That’s the real curse of small sample size: our estimation for the population parameter (60%) is just not accurate.
Here is a table showing the accuracy of such results (I will show its calculation later)

When Product Managers want to strike priority between a “2 out of 5” and a “4 out of 5” the choice seems to be trivial. The “4 out of 5” feels twice as good. But if we look at the intervals (see next chart), we realize that a “4 out of 5” can be anything between 36% and 98%, and that overlaps a lot with the “2 out of 5”-s interval, the 12%–77%. What if the “4 out of 5” is only 36%, but the “2 out of 5” is indeed 77%? See how quickly the tables have turned?

Whenever I have a “4 out of 5” result I feel compelled to tell the Product Manager about this because this is powerful. “4 out of 5” is very persuasive. My research is making an impact.
It’s just not the right kind of impact. Would users be better off if I push through the “4 out of 5” user need? Not so sure. There is a chance that they would be better off with the “2 out of 5”.
Reporting the right amount of confidence
Of course, the right way of reporting reach numbers based on a sample is to calculate the confidence interval. Instead of reporting “3 out of 5”, I report “23%–88%” so stakeholders know how much uncertainty there is in the results.
The confidence interval calculation is made fairly simple by some awesome online calculators, such as this one from MeasuringU. See the 30-second guide on the below image.
Please note:The above calculator works for the two specific use cases I am talking about in this article: (1) Reach of a usability issue, (2) Reach of an unmet customer need. Both usability issues and unmet customer needs are binary variables (1 or 0, the user either has it or doesn’t). If it’s not a binary variable that you are working with, e.g. a usability score ranging from 1 to 5, you will need another statistical method. That, you can easily pick with the help of Sauro and Lewis’ book.
Is this even significant?
When stakeholders hear results from a usability test or an interview, they may ask “Is this even significant?”. In my experience, many of them don’t really know what significance means, but they have a concern about the sample size and this is how they can give voice to their concern. They want to know whether our findings are a peculiarity of these 5 participants, or they hold true for the entire user base.
The confidence interval answers exactly this question (and there is no need to calculate statistical significance).
Take the “3 out of 5” as an example. The confidence interval is 23%–88% on a 95% confidence level. That 95% means, that if we tested another random set of 5 participants 100 times, we would see the usability issue appearing between 23%–88% among the participants in 95 cases of those 100. So this usability issue is not an accidental quirk of our first sample.
On the other hand, if we take the “1 out of 5”, the confidence interval is 2% — 64%, so in this case, there is a chance that this issue only affects a minuscule portion of the population.
Doesn’t population size matter in these estimates?
Let’s take two situations.
- I am an early-stage startup, having only 500 users. I am trying to estimate the Reach of a user problem based on a sample of 5.
- I am an established tech giant, having 5 billion users. I am trying to estimate the Reach of a user problem based on a sample of 5.
Intuitively, the first scenario seems much more viable. Inferring the characteristics of 500 based on 5 seems much more plausible than do the same for 5.000.000.000 based on 5.
Despite our intuition, they aren’t any different. The estimation based on five users would be just as good (or bad) in both cases.
Think of the classic probability thought experiment. You have a drawer full of socks. There are two kinds of socks: black and white. Let’s say the proportion is 1:4 black and white. So every time you close your eyes and pull a random sock from the drawer, there is a 20% chance of getting a black one and 80% of getting a white one. And this is completely independent of whether you have 500 socks in the drawer or 5.000.000.000.
A technique to get an accurate Reach estimate from only a handful of interviews
Even if we calculate the confidence interval from our 5 interviews, the interval is just too wide. It’s not a very accurate estimation for reach. Luckily we have a better technique to estimate reach, and it has nothing to do with statistics.
Here is the solution:
- Talk to your 5 customers as you usually would. For each customer try to detect whether they have the user problem.
- Identify the cause of the problem: Why do these 3 customers have the problem? Why the other 2 don’t? What causes the 3 to have that problem?
- If the root cause is measurable, quantify the prevalence of that.
- If the root cause is not measurable, find something that correlates with it and measure that.
Example 1
Let’s say I have a customer problem in mind. My business users want to see a report on their dashboard. I talk to 5 users and see that 2 of those didn’t really care about this. But the other 3 care so much that they already cobbled together their interim solution: they all have a clutter of sheets held together by the manual work of a devoted intern.
I want to estimate the reach: the number of similar businesses that also have the same problem.
As a first step, I need to identify the root cause of the problem. What makes that 3 need the report and why the remaining 2 don’t care? So I continue the interview and it turns out the 3 are all in the European Union and all in the automotive industry. They need the report because they have a regulatory obligation to make those numbers available for external auditors. The other 2: one is in Asia where they don’t have this regulation. The other is from an entirely different industry that has different regulations.
Now I can go to my company’s CRM and see how many customers we have that are in the EU and in the automotive industry. I can even estimate the total available market for this dashboard feature: open a public registry and count all EU-based automotive companies in it.
Note: all these are just estimations. We can’t tell the Reach exactly, there will always be variables that remain unaccounted for (e.g. a special segment of EU automotive that are exempt from auditing for some reason and we just don’t know because there wasn’t any such in our sample). But this estimation is as good as it gets. We get the estimation, we go with it and refine as we talk to more users.
Example 2
There are cases when it’s easy to quantify the root cause (e.g. industry and location). And there are cases when it’s not feasible.
Let’s say I research whether my users need a collaboration feature within my app. I talk to 5 users, 3 out of 5 need the feature. The differentiating factor seems to be team size. Larger teams need the collaboration feature. Small teams don’t really need it because they don’t have complex review-approval-handover processes. So the root cause behind the customer problem is the complexity of communication or team size. But I can’t measure any of that. I have no way to measure the complexity of communication of my clients and I have no data on my clients’ team size either.
What I can do in this case is try to find another variable that correlates with team size and one that I can measure. E.g. I assume that bigger companies tend to have bigger teams. So now I can hit up Linkedin and check which of my 5 clients have how many employees. The 3 who needed the tool are above 500, the 2 who didn’t are below 500. Based on this I will assume that all companies above 500 employees will need my collaboration feature. Now I can query my CRM or a public registry to learn how many companies there are above 500.
Up to my knowledge, this is the best way to estimate reach from interview data. It doesn’t matter if 1 out of 5, or 4 out of 5 customers have the problem in my sample. The only thing that matters is that I have the root cause behind their problem and I can measure the prevalence of that accurately in some other database.
Let me know if you have other ideas!
Use Telemetry to get accurate Reach estimation for Usability Tests
The above technique works well for estimating the reach of unmet needs. But what about the reach of usability issues? There is a better way to estimate reach for usability issues as well.
Disclaimer: I work in a SaaS that does agile and lean product development. In this context, we rarely need an accurate reach estimation of usability issues. It’s mostly:
- Me: Here is the list of issues. These are the top 10 in my opinion.
- Product Team: Ok, thanks. That’s good enough, we will fix those first.
If you MUST give a Reach number for usability issues, there is a terrific source for that. It is your telemetry tool. Hotjar, Mixpanel, Google Analytics, or something similar.
While these telemetry tools can’t tell how many users ran into a specific usability issue, they can absolutely tell how many users visited the page that has the usability issue. They can tell how many users used the feature that has the usability issue. Some issues happen to be in features that only 13 users used in the last 30 days, some issues come up in features that are being used by thousands and thousands every month. This is enough information to rank usability issues based on their estimated Reach.
Summary
Sometimes we need to tell how prevalent a usability issue or a user need is. Their reach.
For usability issues, we can
- Calculate confidence interval in 10 seconds. If the sample size is small, this only gives a rough idea of Reach.
- Use telemetry data to get a sense of how many users are potentially affected by the issue.
For customer needs, we can
- Calculate confidence interval in 10 seconds. If the sample size is small, this only gives a rough idea of Reach.
- We can identify and then quantify the root cause behind the need. Or if that’s not feasible, we can find a variable that correlates with the root cause and quantify that.
Over to you! Let me know how do you get Reach estimations!
Ask: I am working on a book that is essentially a “Usability Testing mentor.” Helping you self-assess and uplevel your usability testing even when no one can mentor you. Are you interested? Reach out here or Sign-up for early access.

Recommend
About Joyk
Aggregate valuable and interesting links.
Joyk means Joy of geeK