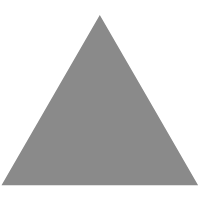
3
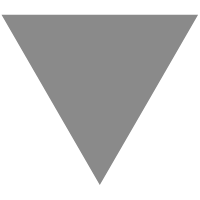
pytorch 实用工具总结 - 极市社区
source link: https://bbs.cvmart.net/articles/269
Go to the source link to view the article. You can view the picture content, updated content and better typesetting reading experience. If the link is broken, please click the button below to view the snapshot at that time.
pytorch 实用工具总结
有用
pytorch 实用工具总结精选
工具
Find me · 发表于 2019-01-30 11:01:48 文章来源: Awsome-Github 资源列表
知乎博主Big Fish从各种资料加上自己实践整理出来的pytorch工具汇总,非常实用,欢迎收藏~
作者:Big Fish
https://zhuanlan.zhihu.com/p/33992733
以下工具主要包括:
模型层数:print_layers_num
模型参数总量:print_model_parm_nums
模型的计算图:def print_autograd_graph():或者参见tensorboad
模型滤波器可视化:show_save_tensor
模型在具体的输入下的尺寸信息summary以及参数量:show_summary
模型计算量:print_model_parm_flops
格式较混乱,但上述代码均可用,后续会继续整理。
#coding:utf8
import torch
import torchvision
import torch.nn as nn
from torch.autograd import Variable
import torchvision.models as models
import numpy as np
def test():
model = models.resnet18()
print model.layer1[0].conv1.weight.data
print model.layer1[0].conv1.__class__#<class 'torch.nn.modules.conv.Conv2d'>
print model.layer1[0].conv1.kernel_size
input = torch.autograd.Variable(torch.randn(20, 16, 50, 100))
print input.size()
print np.prod(input.size())
def print_model_parm_nums():
model = models.alexnet()
total = sum([param.nelement() for param in model.parameters()])
print(' + Number of params: %.2fM' % (total / 1e6))
def print_model_parm_flops():
# prods = {}
# def save_prods(self, input, output):
# print 'flops:{}'.format(self.__class__.__name__)
# print 'input:{}'.format(input)
# print '_dim:{}'.format(input[0].dim())
# print 'input_shape:{}'.format(np.prod(input[0].shape))
# grads.append(np.prod(input[0].shape))
prods = {}
def save_hook(name):
def hook_per(self, input, output):
# print 'flops:{}'.format(self.__class__.__name__)
# print 'input:{}'.format(input)
# print '_dim:{}'.format(input[0].dim())
# print 'input_shape:{}'.format(np.prod(input[0].shape))
# prods.append(np.prod(input[0].shape))
prods[name] = np.prod(input[0].shape)
# prods.append(np.prod(input[0].shape))
return hook_per
list_1=[]
def simple_hook(self, input, output):
list_1.append(np.prod(input[0].shape))
list_2={}
def simple_hook2(self, input, output):
list_2['names'] = np.prod(input[0].shape)
multiply_adds = False
list_conv=[]
def conv_hook(self, input, output):
batch_size, input_channels, input_height, input_width = input[0].size()
output_channels, output_height, output_width = output[0].size()
kernel_ops = self.kernel_size[0] * self.kernel_size[1] * (self.in_channels / self.groups) * (2 if multiply_adds else 1)
bias_ops = 1 if self.bias is not None else 0
params = output_channels * (kernel_ops + bias_ops)
flops = batch_size * params * output_height * output_width
list_conv.append(flops)
list_linear=[]
def linear_hook(self, input, output):
batch_size = input[0].size(0) if input[0].dim() == 2 else 1
weight_ops = self.weight.nelement() * (2 if multiply_adds else 1)
bias_ops = self.bias.nelement()
flops = batch_size * (weight_ops + bias_ops)
list_linear.append(flops)
list_bn=[]
def bn_hook(self, input, output):
list_bn.append(input[0].nelement())
list_relu=[]
def relu_hook(self, input, output):
list_relu.append(input[0].nelement())
list_pooling=[]
def pooling_hook(self, input, output):
batch_size, input_channels, input_height, input_width = input[0].size()
output_channels, output_height, output_width = output[0].size()
kernel_ops = self.kernel_size * self.kernel_size
bias_ops = 0
params = output_channels * (kernel_ops + bias_ops)
flops = batch_size * params * output_height * output_width
list_pooling.append(flops)
def foo(net):
childrens = list(net.children())
if not childrens:
if isinstance(net, torch.nn.Conv2d):
# net.register_forward_hook(save_hook(net.__class__.__name__))
# net.register_forward_hook(simple_hook)
# net.register_forward_hook(simple_hook2)
net.register_forward_hook(conv_hook)
if isinstance(net, torch.nn.Linear):
net.register_forward_hook(linear_hook)
if isinstance(net, torch.nn.BatchNorm2d):
net.register_forward_hook(bn_hook)
if isinstance(net, torch.nn.ReLU):
net.register_forward_hook(relu_hook)
if isinstance(net, torch.nn.MaxPool2d) or isinstance(net, torch.nn.AvgPool2d):
net.register_forward_hook(pooling_hook)
return
for c in childrens:
foo(c)
resnet = models.alexnet()
foo(resnet)
input = Variable(torch.rand(3,224,224).unsqueeze(0), requires_grad = True)
out = resnet(input)
total_flops = (sum(list_conv) + sum(list_linear) + sum(list_bn) + sum(list_relu) + sum(list_pooling))
print(' + Number of FLOPs: %.2fG' % (total_flops / 1e9))
# print list_bn
# print 'prods:{}'.format(prods)
# print 'list_1:{}'.format(list_1)
# print 'list_2:{}'.format(list_2)
# print 'list_final:{}'.format(list_final)
def print_forward():
model = torchvision.models.resnet18()
select_layer = model.layer1[0].conv1
grads={}
def save_grad(name):
def hook(self, input, output):
grads[name] = input
return hook
select_layer.register_forward_hook(save_grad('select_layer'))
input = Variable(torch.rand(3,224,224).unsqueeze(0), requires_grad = True)
out = model(input)
# print grads['select_layer']
print grads
def print_value():
grads = {}
def save_grad(name):
def hook(grad):
grads[name] = grad
return hook
x = Variable(torch.randn(1,1), requires_grad=True)
y = 3*x
z = y**2
# In here, save_grad('y') returns a hook (a function) that keeps 'y' as name
y.register_hook(save_grad('y'))
z.register_hook(save_grad('z'))
z.backward()
print 'HW'
print("grads['y']: {}".format(grads['y']))
print(grads['z'])
def print_layers_num():
# resnet = models.resnet18()
resnet = models.resnet18()
def foo(net):
childrens = list(net.children())
if not childrens:
if isinstance(net, torch.nn.Conv2d):
print ' '
#可以用来统计不同层的个数
# net.register_backward_hook(print)
return 1
count = 0
for c in childrens:
count += foo(c)
return count
print(foo(resnet))
def check_summary():
def torch_summarize(model, show_weights=True, show_parameters=True):
"""Summarizes torch model by showing trainable parameters and weights."""
from torch.nn.modules.module import _addindent
tmpstr = model.__class__.__name__ + ' (\n'
for key, module in model._modules.items():
# if it contains layers let call it recursively to get params and weights
if type(module) in [
torch.nn.modules.container.Container,
torch.nn.modules.container.Sequential
]:
modstr = torch_summarize(module)
else:
modstr = module.__repr__()
modstr = _addindent(modstr, 2)
params = sum([np.prod(p.size()) for p in module.parameters()])
weights = tuple([tuple(p.size()) for p in module.parameters()])
tmpstr += ' (' + key + '): ' + modstr
if show_weights:
tmpstr += ', weights={}'.format(weights)
if show_parameters:
tmpstr += ', parameters={}'.format(params)
tmpstr += '\n'
tmpstr = tmpstr + ')'
return tmpstr
# Test
import torchvision.models as models
model = models.alexnet()
print(torch_summarize(model))
#https://gist.github.com/wassname/0fb8f95e4272e6bdd27bd7df386716b7
#summarize a torch model like in keras, showing parameters and output shape
def show_summary():
from collections import OrderedDict
import pandas as pd
import numpy as np
import torch
from torch.autograd import Variable
import torch.nn.functional as F
from torch import nn
def get_names_dict(model):
"""
Recursive walk to get names including path
"""
names = {}
def _get_names(module, parent_name=''):
for key, module in module.named_children():
name = parent_name + '.' + key if parent_name else key
names[name]=module
if isinstance(module, torch.nn.Module):
_get_names(module, parent_name=name)
_get_names(model)
return names
def torch_summarize_df(input_size, model, weights=False, input_shape=True, nb_trainable=False):
"""
Summarizes torch model by showing trainable parameters and weights.
author: wassname
url: https://gist.github.com/wassname/0fb8f95e4272e6bdd27bd7df386716b7
license: MIT
Modified from:
- https://github.com/pytorch/pytorch/issues/2001#issuecomment-313735757
- https://gist.github.com/wassname/0fb8f95e4272e6bdd27bd7df386716b7/
Usage:
import torchvision.models as models
model = models.alexnet()
df = torch_summarize_df(input_size=(3, 224,224), model=model)
print(df)
# name class_name input_shape output_shape nb_params
# 1 features=>0 Conv2d (-1, 3, 224, 224) (-1, 64, 55, 55) 23296#(3*11*11+1)*64
# 2 features=>1 ReLU (-1, 64, 55, 55) (-1, 64, 55, 55) 0
# ...
"""
def register_hook(module):
def hook(module, input, output):
name = ''
for key, item in names.items():
if item == module:
name = key
#<class 'torch.nn.modules.conv.Conv2d'>
class_name = str(module.__class__).split('.')[-1].split("'")[0]
module_idx = len(summary)
m_key = module_idx + 1
summary[m_key] = OrderedDict()
summary[m_key]['name'] = name
summary[m_key]['class_name'] = class_name
if input_shape:
summary[m_key][
'input_shape'] = (-1, ) + tuple(input[0].size())[1:]
summary[m_key]['output_shape'] = (-1, ) + tuple(output.size())[1:]
if weights:
summary[m_key]['weights'] = list(
[tuple(p.size()) for p in module.parameters()])
# summary[m_key]['trainable'] = any([p.requires_grad for p in module.parameters()])
if nb_trainable:
params_trainable = sum([torch.LongTensor(list(p.size())).prod() for p in module.parameters() if p.requires_grad])
summary[m_key]['nb_trainable'] = params_trainable
params = sum([torch.LongTensor(list(p.size())).prod() for p in module.parameters()])
summary[m_key]['nb_params'] = params
if not isinstance(module, nn.Sequential) and \
not isinstance(module, nn.ModuleList) and \
not (module == model):
hooks.append(module.register_forward_hook(hook))
# Names are stored in parent and path+name is unique not the name
names = get_names_dict(model)
# check if there are multiple inputs to the network
if isinstance(input_size[0], (list, tuple)):
x = [Variable(torch.rand(1, *in_size)) for in_size in input_size]
else:
x = Variable(torch.rand(1, *input_size))
if next(model.parameters()).is_cuda:
x = x.cuda()
# create properties
summary = OrderedDict()
hooks = []
# register hook
model.apply(register_hook)
# make a forward pass
model(x)
# remove these hooks
for h in hooks:
h.remove()
# make dataframe
df_summary = pd.DataFrame.from_dict(summary, orient='index')
return df_summary
# Test on alexnet
import torchvision.models as models
model = models.alexnet()
df = torch_summarize_df(input_size=(3, 224, 224), model=model)
print(df)
# # Output
# name class_name input_shape output_shape nb_params
# 1 features=>0 Conv2d (-1, 3, 224, 224) (-1, 64, 55, 55) 23296#nn.Conv2d(3, 64, kernel_size=11, stride=4, padding=2),
# 2 features=>1 ReLU (-1, 64, 55, 55) (-1, 64, 55, 55) 0
# 3 features=>2 MaxPool2d (-1, 64, 55, 55) (-1, 64, 27, 27) 0
# 4 features=>3 Conv2d (-1, 64, 27, 27) (-1, 192, 27, 27) 307392
# 5 features=>4 ReLU (-1, 192, 27, 27) (-1, 192, 27, 27) 0
# 6 features=>5 MaxPool2d (-1, 192, 27, 27) (-1, 192, 13, 13) 0
# 7 features=>6 Conv2d (-1, 192, 13, 13) (-1, 384, 13, 13) 663936
# 8 features=>7 ReLU (-1, 384, 13, 13) (-1, 384, 13, 13) 0
# 9 features=>8 Conv2d (-1, 384, 13, 13) (-1, 256, 13, 13) 884992
# 10 features=>9 ReLU (-1, 256, 13, 13) (-1, 256, 13, 13) 0
# 11 features=>10 Conv2d (-1, 256, 13, 13) (-1, 256, 13, 13) 590080
# 12 features=>11 ReLU (-1, 256, 13, 13) (-1, 256, 13, 13) 0
# 13 features=>12 MaxPool2d (-1, 256, 13, 13) (-1, 256, 6, 6) 0
# 14 classifier=>0 Dropout (-1, 9216) (-1, 9216) 0
# 15 classifier=>1 Linear (-1, 9216) (-1, 4096) 37752832
# 16 classifier=>2 ReLU (-1, 4096) (-1, 4096) 0
# 17 classifier=>3 Dropout (-1, 4096) (-1, 4096) 0
# 18 classifier=>4 Linear (-1, 4096) (-1, 4096) 16781312
# 19 classifier=>5 ReLU (-1, 4096) (-1, 4096) 0
# 20 classifier=>6 Linear (-1, 4096) (-1, 1000) 4097000
def show_save_tensor():
import torch
from torchvision import utils
import torchvision.models as models
from matplotlib import pyplot as plt
def vis_tensor(tensor, ch = 0, all_kernels=False, nrow=8, padding = 2):
'''
ch: channel for visualization
allkernels: all kernels for visualization
'''
n,c,h,w = tensor.shape
if all_kernels:
tensor = tensor.view(n*c ,-1, w, h)
elif c != 3:
tensor = tensor[:, ch,:,:].unsqueeze(dim=1)
rows = np.min((tensor.shape[0]//nrow + 1, 64 ))
grid = utils.make_grid(tensor, nrow=nrow, normalize=True, padding=padding)
# plt.figure(figsize=(nrow,rows))
plt.imshow(grid.numpy().transpose((1, 2, 0)))#CHW HWC
def save_tensor(tensor, filename, ch=0, all_kernels=False, nrow=8, padding=2):
n,c,h,w = tensor.shape
if all_kernels:
tensor = tensor.view(n*c ,-1, w, h)
elif c != 3:
tensor = tensor[:, ch,:,:].unsqueeze(dim=1)
utils.save_image(tensor, filename, nrow = nrow,normalize=True, padding=padding)
vgg = models.resnet18(pretrained=True)
mm = vgg.double()
filters = mm.modules
body_model = [i for i in mm.children()][0]
# layer1 = body_model[0]
layer1 = body_model
tensor = layer1.weight.data.clone()
vis_tensor(tensor)
save_tensor(tensor,'test.png')
plt.axis('off')
plt.ioff()
plt.show()
def print_autograd_graph():
from graphviz import Digraph
import torch
from torch.autograd import Variable
def make_dot(var, params=None):
""" Produces Graphviz representation of PyTorch autograd graph
Blue nodes are the Variables that require grad, orange are Tensors
saved for backward in torch.autograd.Function
Args:
var: output Variable
params: dict of (name, Variable) to add names to node that
require grad (TODO: make optional)
"""
if params is not None:
#assert all(isinstance(p, Variable) for p in params.values())
param_map = {id(v): k for k, v in params.items()}
node_attr = dict(style='filled',
shape='box',
align='left',
fontsize='12',
ranksep='0.1',
height='0.2')
dot = Digraph(node_attr=node_attr, graph_attr=dict(size="12,12"))
seen = set()
def size_to_str(size):
return '('+(', ').join(['%d' % v for v in size])+')'
def add_nodes(var):
if var not in seen:
if torch.is_tensor(var):
dot.node(str(id(var)), size_to_str(var.size()), fillcolor='orange')
elif hasattr(var, 'variable'):
u = var.variable
#name = param_map[id(u)] if params is not None else ''
#node_name = '%s\n %s' % (name, size_to_str(u.size()))
node_name = '%s\n %s' % (param_map.get(id(u.data)), size_to_str(u.size()))
dot.node(str(id(var)), node_name, fillcolor='lightblue')
else:
dot.node(str(id(var)), str(type(var).__name__))
seen.add(var)
if hasattr(var, 'next_functions'):
for u in var.next_functions:
if u[0] is not None:
dot.edge(str(id(u[0])), str(id(var)))
add_nodes(u[0])
if hasattr(var, 'saved_tensors'):
for t in var.saved_tensors:
dot.edge(str(id(t)), str(id(var)))
add_nodes(t)
add_nodes(var.grad_fn)
return dot
from torchvision import models
torch.manual_seed(1)
inputs = torch.randn(1,3,224,224)
model = models.resnet18(pretrained=False)
y = model(Variable(inputs))
#print(y)
g = make_dot(y, params=model.state_dict())
g.view()
#g
if __name__=='__main__':
import fire
fire. Fire()
3
0
6420

Recommend
About Joyk
Aggregate valuable and interesting links.
Joyk means Joy of geeK