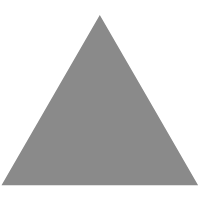
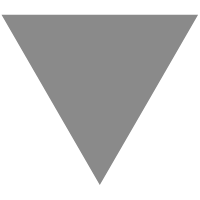
INLG: What real-world NLG users want
source link: https://ehudreiter.com/2021/09/27/inlg-what-real-world-users-want/
Go to the source link to view the article. You can view the picture content, updated content and better typesetting reading experience. If the link is broken, please click the button below to view the snapshot at that time.
Ehud Reiter's Blog
Ehud's thoughts and observations about Natural Language Generation
INLG was last week, organised from Aberdeen. It ended up being almost entirely online, which was a real shame, but anyways lots of really interesting and thought-provoking stuff. Some excellent papers, my personal favourites were van Miltenburg et al’s paper on error reporting, and Ciora et al’s paper on covert gender bias. As usual, though, the real “value-added” of the conference to me was not so much the papers (which I can read without attending INLG), but the community events: invited speakers, panel, generation challenges, and discussion/social sessions. Despite INLG being online, I still managed to have some really interesting discussions with people, I think I’m getting better at online events.
Anyways, there is a lot I can write blogs about, but I wanted to the start with the panel on “What users want from real-world NLG”. The panel, which was moderated by Mike White , had four people who worked for small-medium companies, all of whom are practitioners who build systems: Adam Sam (Monok), Ross Turner (Arria), Robert Weißgraeber (Ax Semantics), and Michelle Zhou (Juji). They were asked to discuss what real-world NLG users wanted, partially in the hope that this perspective could influence the academic research agenda. A lot of really interesting points were made, I summarise a few of them below. Please note this is *my* interpretation, and blame me (not the panelists) if I get something wrong!
The panel pointed out that we need to distinguish between two types of users
- Companies which buy (or commission) NLG systems for their clients or employees
- End-users who read and interact with NLG system
Trust
It is absolutely essential that users trust NLG systems. Trust is built up over time when a systems does a good job; it is very rapidly lost if the system makes mistakes by producing inaccurate or ungrammatical texts (accuracy errors can be especially damaging). This applies to both companies and end users: if a company thinks an NLG system is damaging its brand or opening the possibility of lawsuits, it will not use NLG! Quality assurance is extremely important, indeed sometimes companies ask to see all possible texts that could be produced by the NLG system. Transparency also matters; owners should have good understanding of what an NLG system can and cannot do.
Researcher perspective: Trust, quality assurance, and transparency are all interesting research areas. Also, NLG systems which reliably produce decent texts are better than systems which produces a mixture of excellent and dreadful texts, even if the latter have better average/mean text quality.
Authoring
Subject matter experts (SMEs), knowledge workers, and copywriters should be able to create or at least configure NLG systems. Hence the creation/configuration process needs to be accessible to people who do not have a background in linguistics, software development, or machine learning.
Researcher perspective: Develop system-building approaches which do not require PhDs in ML, AI, CS, or linguistics.
Configuration and Control
A related point is that companies that deploy NLG system needs to be able to configure and adapt them. If they dont like the way a particular kind of text reads, they need to be able to modify wording, and perhaps change the length of a text. In some contexts it is also essential to be able to easily update a system when the world changes (domain shift). This point was also made by the invited speaker Tim Bickmore for heath chatbots about Covid; since Covid situation and rules are constantly changing, it needs to be easy to update the system accordingly.
Researcher perspective: How can we make NLG systems configurable, and move away from black boxes which cannot be configured? What is the best way of configuring systems from a UI/UX (user experience) perspective? How do we update systems when the world changes and our corpora are no longer appropriate?
Human in Loop
In many contexts NLG systems are best used to help human writers, instead of automatically generate final texts. This can be done by a “human in the loop” architecture where the NLG system generates a draft which a person post-edits. Success is measured by the time taken by the post-editor to produce a high-quality text, not by the quality of the drafts produced by the NLG system.
Researcher perspective: NLG systems which are used in this manner should be designed to produce draft texts which are easy to post-edit. What are the characteristics of such text? How should post-editing be done from a UI/UX perspective?
Other selected points (briefly)
- NLG systems need to support a company’s brand. If a company’s brand is based on a particular personality or language style, texts produced by NLG systems must conform to this brand.
- In some contexts it is useful to personalise texts according to the end user’s circumstances, personality, emotional state, etc. How can this be done?
- NLG systems must solve real business problem. Most academic systems address artificial made-up problems, can more emphasis be put on real problems?
- NLG systems must be maintainable, like any other software system. Are there specific challenges for maintaining NLG systems (compared to generic software systems)?
- Content is often more important than expression. However, the research community currently does not do a lot of work on content/insights for NLG.
- We need evaluation techniques for the above criteria. Ideally these would evaluate different aspects separately, so we get a multi-faceted evaluation instead of a single number.
- What can NLG learn from the human writing process?
- Hype around AI and GPT3 is not helpful, because it gives customers very unrealistic expectations. Can also damage trust in NLG technology when people realise reality does not meet hype.
- Variation and paraphrasing are really important in some contexts. In other contexts, language is constrained or even regulated, so variation is not desirable.
- NLG systems are usually integrated with other systems to form complete solutions. Hence they need integration APIs as well as user interfaces.
- Responsible NLG: In marketing contexts, NLG is sometimes used to “upsell” and try to get customer to spend more money. Should this be regulated/controlled?
Final Thoughts
I was on a panel at the GEM workshop this year, and someone asked if neural NLG technology (in 2021) was usable in real applications. I responded that in general neural NLG tech was not usable (with a few exceptions), because of accuracy/hallucination problems. Looking back, this response was too narrow. The NLG community needs to address all of the above issues, not just accuracy/hallucination. And these issues impact rule-based as well as neural NLG.
What is depressing to me is that a large chunk of the research community ignores the above issues, and instead fixates on creating models which win leaderboards based on artificial tasks, dubious data sets, and evaluation criteria which ignore all of the above factors. But having said this, I am seeing more work recently on issues related to trust (such as safety) which is encouraging. I also know of people working on some of the other issues mentioned above, including configuration and personalisation.
One of the commercial/practitioner attendees at INLG (not on the panel) told me that she struggled with a lot of the INLG papers, but liked the shared task on evaluating accuracy, because this addressed a real “pain point” related to trustworthiness, and she would love to see new ideas from the research community. This shows that it is possible to create shared tasks which encourage research in the above issues, I hope to see more such in the future!
Related
Recommend
About Joyk
Aggregate valuable and interesting links.
Joyk means Joy of geeK