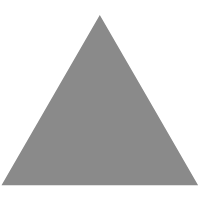
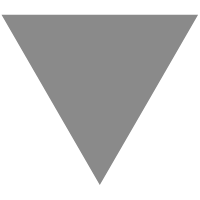
GitHub - babysor/MockingBird: 🚀AI拟声: 5秒内克隆您的声音并生成任意语音内容 Clone...
source link: https://github.com/babysor/MockingBird
Go to the source link to view the article. You can view the picture content, updated content and better typesetting reading experience. If the link is broken, please click the button below to view the snapshot at that time.
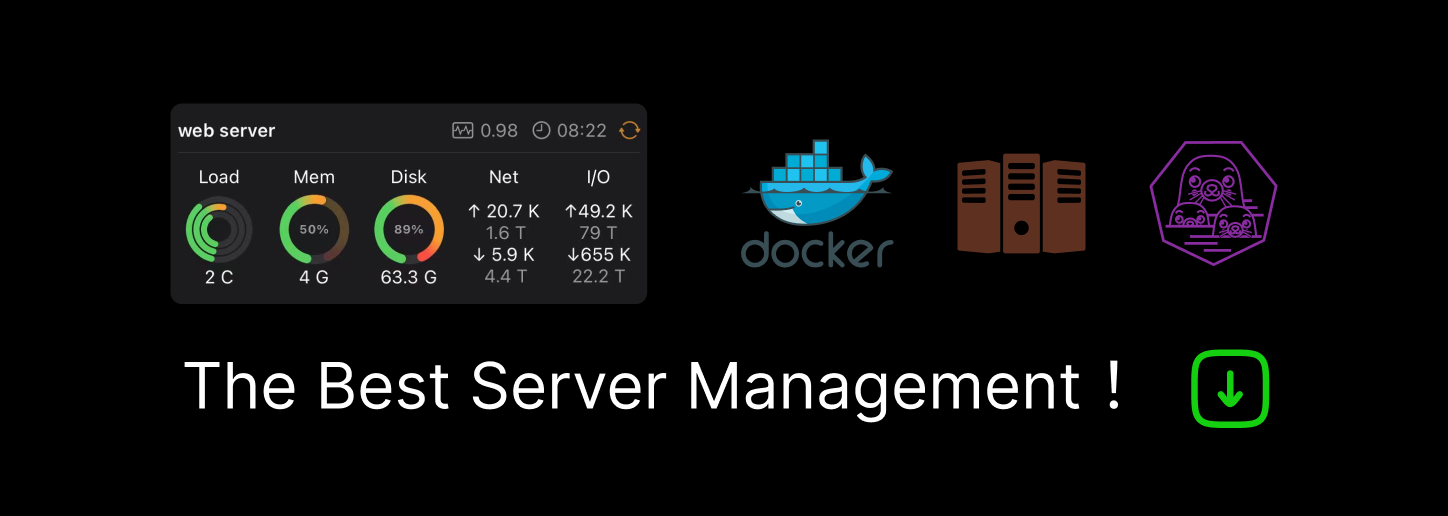
English | 中文
Features
Chinese supported mandarin and tested with multiple datasets: aidatatang_200zh, magicdata, aishell3, and etc.
PyTorch worked for pytorch, tested in version of 1.9.0(latest in August 2021), with GPU Tesla T4 and GTX 2060
Windows + Linux run in both Windows OS and linux OS (even in M1 MACOS)
Easy & Awesome effect with only newly-trained synthesizer, by reusing the pretrained encoder/vocoder
DEMO VIDEO
Quick Start
1. Install Requirements
Follow the original repo to test if you got all environment ready. **Python 3.7 or higher ** is needed to run the toolbox.
- Install PyTorch.
If you get an
ERROR: Could not find a version that satisfies the requirement torch==1.9.0+cu102 (from versions: 0.1.2, 0.1.2.post1, 0.1.2.post2 )
This error is probably due to a low version of python, try using 3.9 and it will install successfully
- Install ffmpeg.
- Run
pip install -r requirements.txt
to install the remaining necessary packages. - Install webrtcvad
pip install webrtcvad-wheels
(If you need)
Note that we are using the pretrained encoder/vocoder but synthesizer, since the original model is incompatible with the Chinese sympols. It means the demo_cli is not working at this moment.
2. Train synthesizer with your dataset
- Download aidatatang_200zh or other dataset and unzip: make sure you can access all .wav in train folder
- Preprocess with the audios and the mel spectrograms:
python pre.py <datasets_root>
Allow parameter--dataset {dataset}
to support aidatatang_200zh, magicdata, aishell3
If it happens
the page file is too small to complete the operation
, please refer to this video and change the virtual memory to 100G (102400), for example : When the file is placed in the D disk, the virtual memory of the D disk is changed.
-
Train the synthesizer:
python synthesizer_train.py mandarin <datasets_root>/SV2TTS/synthesizer
-
Go to next step when you see attention line show and loss meet your need in training folder synthesizer/saved_models/.
FYI, my attention came after 18k steps and loss became lower than 0.4 after 50k steps.
2.2 Use pretrained model of synthesizer
Thanks to the community, some models will be shared:
A link to my early trained model: Baidu Yun Code:aid4
2.3 Train vocoder (Optional)
-
Preprocess the data:
python vocoder_preprocess.py <datasets_root>
-
Train the wavernn vocoder:
python vocoder_train.py mandarin <datasets_root>
-
Train the hifigan vocoder
python vocoder_train.py mandarin <datasets_root> hifigan
3. Launch the Toolbox
You can then try the toolbox:
python demo_toolbox.py -d <datasets_root>
or
python demo_toolbox.py
Good news
: Chinese Characters are supported
Reference
URL Designation Title Implementation source 2010.05646 HiFi-GAN (vocoder) Generative Adversarial Networks for Efficient and High Fidelity Speech Synthesis This repo 1806.04558 SV2TTS Transfer Learning from Speaker Verification to Multispeaker Text-To-Speech Synthesis This repo 1802.08435 WaveRNN (vocoder) Efficient Neural Audio Synthesis fatchord/WaveRNN 1703.10135 Tacotron (synthesizer) Tacotron: Towards End-to-End Speech Synthesis fatchord/WaveRNN 1710.10467 GE2E (encoder) Generalized End-To-End Loss for Speaker Verification This repoThis repository is forked from Real-Time-Voice-Cloning which only support English.
1.Where can I download the dataset?
aidatatang_200zh、magicdata、aishell3
After unzip aidatatang_200zh, you need to unzip all the files under
aidatatang_200zh\corpus\train
2.What is<datasets_root>
?
If the dataset path is D:\data\aidatatang_200zh
,then <datasets_root>
isD:\data
3.Not enough VRAM
Train the synthesizer:adjust the batch_size in synthesizer/hparams.py
//Before
tts_schedule = [(2, 1e-3, 20_000, 12), # Progressive training schedule
(2, 5e-4, 40_000, 12), # (r, lr, step, batch_size)
(2, 2e-4, 80_000, 12), #
(2, 1e-4, 160_000, 12), # r = reduction factor (# of mel frames
(2, 3e-5, 320_000, 12), # synthesized for each decoder iteration)
(2, 1e-5, 640_000, 12)], # lr = learning rate
//After
tts_schedule = [(2, 1e-3, 20_000, 8), # Progressive training schedule
(2, 5e-4, 40_000, 8), # (r, lr, step, batch_size)
(2, 2e-4, 80_000, 8), #
(2, 1e-4, 160_000, 8), # r = reduction factor (# of mel frames
(2, 3e-5, 320_000, 8), # synthesized for each decoder iteration)
(2, 1e-5, 640_000, 8)], # lr = learning rate
Train Vocoder-Preprocess the data:adjust the batch_size in synthesizer/hparams.py
//Before
### Data Preprocessing
max_mel_frames = 900,
rescale = True,
rescaling_max = 0.9,
synthesis_batch_size = 16, # For vocoder preprocessing and inference.
//After
### Data Preprocessing
max_mel_frames = 900,
rescale = True,
rescaling_max = 0.9,
synthesis_batch_size = 8, # For vocoder preprocessing and inference.
Train Vocoder-Train the vocoder:adjust the batch_size in vocoder/wavernn/hparams.py
//Before
# Training
voc_batch_size = 100
voc_lr = 1e-4
voc_gen_at_checkpoint = 5
voc_pad = 2
//After
# Training
voc_batch_size = 6
voc_lr = 1e-4
voc_gen_at_checkpoint = 5
voc_pad =2
4.If it happens RuntimeError: Error(s) in loading state_dict for Tacotron: size mismatch for encoder.embedding.weight: copying a param with shape torch.Size([70, 512]) from checkpoint, the shape in current model is torch.Size([75, 512]).
Please refer to issue #37
5. How to improve CPU and GPU occupancy rate?
Adjust the batch_size as appropriate to improve
Recommend
-
16
Using recursive combinators to enhance functional composition In this essay we’re going to look at the mockingbird , also called the M combinator. The mockingbird is one of the
-
34
In To Grok a Mockingbird , we were introduced to the Mockingbird, a recursive combinator that decouples recursive functions from themselves. Decoup...
-
6
这个 GitHub 项目能克隆你的声音 (查看原文) 今天推荐一个黑科技开源项目,只需要你 5 秒钟的声音对话,就能克隆出你的声音,而且...
-
24
This repository is forked from Real-Time-Voice-Cloning which only support English. English |
-
9
大家好,这里是每周更新的Github精彩分享周刊,我是每周都在搬砖的蛮三刀酱。我会从Github热门趋势榜里选出 高质量、有趣,牛B 的开源项目进行分享。1.
-
5
麻省理工科技评论-全民声音克隆时代将到来!30分钟克可完成声音克隆,家人都无法分辨真假全民声音克隆时代将到来!30分钟克可完成声音克隆,家人都无法分辨真假在过去的几十年里,许多经典的 TVB 影视片都离不开配音,如果你认为周星驰...
-
6
GitHub 出现超 35000 个恶意攻击文件 GitHub 出现超 35000 个恶意攻击文件 / 克隆仓库 作者:OSCHINA 2022-08-05 15:35:12 安全
-
5
2023-12-12 06:26 Meta 推出可克隆声音并生成环境音效的人工智能 Audiobox 据 VentureBeat 报道,12 月 12 日,Meta 旗下 Facebook 人工智能研究(FAIR)实验室推出可克隆声音并生成环境音效的人工智能 Audiobox。它被描述为“...
-
7
Clone-Voice :简易的AI声音克隆工具,免费开源下载 1月 3, 2024 发表于: 优秀设计资源.
-
6
Home / News / McAfee introduces AI-powered deepfake audio detection tool, P...
About Joyk
Aggregate valuable and interesting links.
Joyk means Joy of geeK