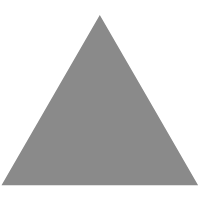
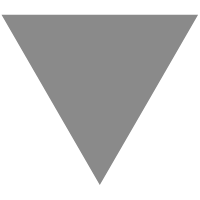
Spark – Cannot perform Merge as multiple source rows matched… | SQL with Manoj
source link: https://sqlwithmanoj.com/2021/06/18/spark-cannot-perform-merge-as-multiple-source-rows-matched/
Go to the source link to view the article. You can view the picture content, updated content and better typesetting reading experience. If the link is broken, please click the button below to view the snapshot at that time.
Spark – Cannot perform Merge as multiple source rows matched…
In SQL when you are syncing a table (target) from an another table (source) you need to make sure there are no duplicates or repeated datasets in either of the Source or Target tables, otherwise you get following error:
UnsupportedOperationException: Cannot perform Merge as multiple source rows matched and attempted to modify the same target row in the Delta table in possibly conflicting ways. By SQL semantics of Merge, when multiple source rows match on the same target row, the result may be ambiguous as it is unclear which source row should be used to update or delete the matching target row. You can preprocess the source table to eliminate the possibility of multiple matches. Please refer to https://docs.microsoft.com/azure/databricks/delta/delta-update#upsert-into-a-table-using-merge
The above error says that while doing MERGE operation on the Target table there shouldn’t be any duplicates in the Source table. This check is applied implicitly by the SQL engine to avoid unnecessary updates and avoid inconsistent data.
So, to avoid this issue make sure you have de-duplication logic before the MERGE operation.
Below is a small demo to reproduce this error.
Let’s create two sample tables (Source & Target) for our demo purpose:
val
df
1
=
Seq((
1
,
"Brock"
,
30
),
(
2
,
"John"
,
31
),
(
2
,
"Andy"
,
35
),
//duplicate ID = 2
(
3
,
"Jane"
,
25
),
(
4
,
"Maria"
,
30
)).toDF(
"Id"
,
"name"
,
"age"
)
spark.sql(
"drop table if exists tblPerson"
)
df
1
.write.format(
"delta"
).saveAsTable(
"tblPerson"
)
val
df
2
=
Seq((
1
,
"Jane"
,
30
),
(
2
,
"John"
,
31
)).toDF(
"Id"
,
"name"
,
"age"
)
spark.sql(
"drop table if exists tblPersonTarget"
)
df
2
.write.format(
"delta"
).saveAsTable(
"tblPersonTarget"
)
Next we will try to MERGE the tables and running the query will result in an error:
val
mergeQuery
=
s
""
"MERGE INTO tblPersonTarget As tgt
Using tblPerson as src
ON src.Id = tgt.ID
WHEN MATCHED
THEN UPDATE
SET
tgt.name = src.name,
tgt.age = src.age
WHEN NOT MATCHED
THEN INSERT (
ID,
name,
age
)
VALUES (
src.ID,
src.name,
src.age
)"
""
spark.sql(mergeQuery)
To remove duplicates you can simply try removing by using window functions or some logic as per your business requirement:
import
org.apache.spark.sql.expressions.Window
import
org.apache.spark.sql.functions.
_
val
df
2
=
df
1
.withColumn(
"rn"
, row
_
number().over(window.partitionBy(
"Id"
).orderBy(
"name"
)))
val
df
3
=
df
2
.filter(
"rn = 1"
)
display(df
3
)
Related
OUTPUT clause and MERGE statement in SQL Server
Just responded to a post in MSDN forum, link: http://social.msdn.microsoft.com/Forums/en-US/transactsql/thread/c06e1db4-7fd6-43c4-8569-5335d555dac8 Accroding to MS-BOL, OUTPUT clause returns information from, or expressions based on, each row affected by an INSERT, UPDATE, DELETE, or MERGE statement. These results can be returned to the processing application for use in such things as confirmation messages,…
November 25, 2010In "SQL Server 2008"
Recommend
About Joyk
Aggregate valuable and interesting links.
Joyk means Joy of geeK