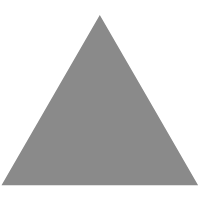
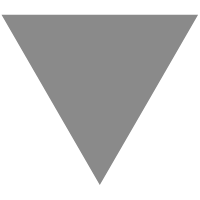
Adapting Predictive Planning to the New Normal of Planning
source link: https://blogs.sap.com/2021/07/02/adapting-predictive-planning-to-the-new-normal-of-planning/
Go to the source link to view the article. You can view the picture content, updated content and better typesetting reading experience. If the link is broken, please click the button below to view the snapshot at that time.
Revealing the Master Plan
It’s now a year and half since the COVID-19 pandemic started. Since then, we do live and work in the new normal. In this new reality, companies like Roche continuously plan the future evolution of their business activities. They rely on predictive capabilities to help them be faster and more accurate when doing so.
When the pandemic broke, circa April-May 2020, we assessed the situation as detailed in the introduction blog to these series:
- What is the nature of the disruptions affecting business time series?
- How can we evolve automated time series forecasting algorithms to provide the best possible insights and mitigate as much as possible the ongoing disruptions?
- How to best guide our customers to handle the corresponding data?
This assessment resulted in several focused improvements that we delivered over the recent SAP Analytics Cloud 2021 releases, forming a cohesive investment theme:
- In the February release (aka 2021.Q1 QRC), we improved the handling of the most recent data and how it influences the overall logic of the time series forecasting model. You can read more to this here.
- In the May release (aka 2021.Q2 QRC), we promoted exponential smoothing techniques as first-class citizens of the internal, automated machine learning competition, leading overall to increased accuracy and simpler models. You can read more to this here. My colleague Thierry Brunet wrote a detailed blog to unveil the cover on this integration. Besides the predictive techniques we use, what’s unique is how we provide insights in our simple and straightforward user interface, providing accuracy alongside trust.
- In the upcoming August release (aka 2021.Q3 QRC), we will deliver the detection of multiple linear trends. This is nicknamed piece-wise linear trend in data science terms. Piece-wise linear trend is an excellent technique when trends fluctuate over time. Please note this improvement is made available with SAP Analytics Cloud wave 2021.13.
Re-Imagining Trend Detection
Showing improvements in SAP Analytics Cloud speak louder than words. I selected a few examples that exemplify the improvements we brought. For each example I am presenting the situation before the improvement and after the improvement.
Evolution of Flights in Cleveland Airport
Before:
- Mean error (Horizon-Wide MAPE): 8,58%
- Predictive Model: quadratic trend and fluctuations – somewhat complex.
After:
- Mean error (Horizon-Wide MAPE): 2,57%
- Predictive Model: different linear trends over time, monthly cycles – easier to understand
- The model error (Horizon-Wide MAPE) is reduced by a factor of 3,3 when comparing the model before and the model after. The predictive forecasts are more realistic given the recent trend evolution.
Passenger Enplanements in the United States
Before:
- Mean error (Horizon-Wide MAPE): 102,47%
- Predictive Model: simple lag and fluctuations – somewhat complex.
After:
- Mean error (Horizon-Wide MAPE): 42,67%
- Predictive Model: different linear trends over time, fluctuations – easier to understand
- Error (Horizon-Wide MAPE) is reduced by a factor of 2,4. We can notice this visually as we do know the actual enplanements from July 2020 to March 2021 (invisible to the predictive model both for the model before and after!) and we can compare the predictive forecasts and the actuals.
Evolution of COVID-19 new cases in Myanmar
Before:
- Mean error (Horizon-Wide MAPE): 1252,37%
- Predictive Model: simple lag model.
After:
- Mean error (Horizon-Wide MAPE): 57,51%
- Predictive Model: different linear trends over time, weekly cycles – easier to understand
- Error (Horizon-Wide MAPE) is reduced by a factor of 21,8.
Please note that not all predictive models will show significant improvement or be modeled with multiple linear trends. This is expected and a particularly good sign. Many of our predictive models are already optimized to handle time series in the best possible way.
So, in terms of average accuracy this specific improvement is bringing a few percentage points, which is already a nice benefit, and sometimes this radically improves predictive models.
The move that is much more impressive and that this change proves is the ability to keep the average performance at a comparable level while replacing more complex models by simpler models that business users will more easily understand and trust.
Finally, while we automate the time series forecasting logic to handle as many time series as possible out of the box, it’s important to remember that the predictive results will differ based on the data used to train the predictive models.
Depending on the exact nature of the disruption (minor impact, major impact, lasting disruption) we provided guidance via the blog here so that you can handle the source data used by our automated predictive engine in the best way and in the best interest of business planning activities.
The Future of Predictive Planning
This recent improvement proves how seriously we consider ongoing events and how they affect the ability of our customers to predict and plan.
We will not rest on our laurels and will continue down the path:
- On one side getting constant feedback from our customers
- On the other side constantly improving our automated time series forecasting logic to provide the best possible combination of accuracy and trust.
Predictive Planning has been designed from day 1 to be accurate, self-service and trustworthy. Predictive Planning is the new normal of Planning.
As part of the plans for the November release, to accelerate on self-service, we will provide support of hierarchies to ease the creation of predictive forecasts at the most adequate aggregation level. More developments are also being planned.
Do not hesitate to leverage our SAP Analytics Cloud Customer Influence portal to raise your specific ideas or vote existing ideas.
Conclusion
I hope this blog post was helpful to you, thanks for your interest!
Feel free to comment and bring your perspective.
If you want to learn more about Predictive Planning in SAP Analytics Cloud:
If you want to learn more about SAP Analytics Cloud:
Recommend
About Joyk
Aggregate valuable and interesting links.
Joyk means Joy of geeK