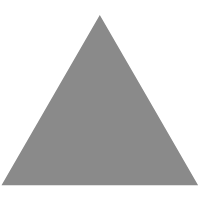
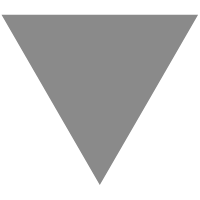
DeepMind says reinforcement learning is 'enough' to reach general AI
source link: https://venturebeat.com/2021/06/09/deepmind-says-reinforcement-learning-is-enough-to-reach-general-ai/
Go to the source link to view the article. You can view the picture content, updated content and better typesetting reading experience. If the link is broken, please click the button below to view the snapshot at that time.
DeepMind says reinforcement learning is ‘enough’ to reach general AI
Elevate your enterprise data technology and strategy at Transform 2021.
In their decades-long chase to create artificial intelligence, computer scientists have designed and developed all kinds of complicated mechanisms and technologies to replicate vision, language, reasoning, motor skills, and other abilities associated with intelligent life. While these efforts have resulted in AI systems that can efficiently solve specific problems in limited environments, they fall short of developing the kind of general intelligence seen in humans and animals.
In a new paper submitted to the peer-reviewed Artificial Intelligence journal, scientists at U.K.-based AI lab DeepMind argue that intelligence and its associated abilities will emerge not from formulating and solving complicated problems but by sticking to a simple but powerful principle: reward maximization.
Titled “Reward is Enough,” the paper, which is still in pre-proof as of this writing, draws inspiration from studying the evolution of natural intelligence as well as drawing lessons from recent achievements in artificial intelligence. The authors suggest that reward maximization and trial-and-error experience are enough to develop behavior that exhibits the kind of abilities associated with intelligence. And from this, they conclude that reinforcement learning, a branch of AI that is based on reward maximization, can lead to the development of artificial general intelligence.
Two paths for AI
One common method for creating AI is to try to replicate elements of intelligent behavior in computers. For instance, our understanding of the mammal vision system has given rise to all kinds of AI systems that can categorize images, locate objects in photos, define the boundaries between objects, and more. Likewise, our understanding of language has helped in the development of various natural language processing systems, such as question answering, text generation, and machine translation.
These are all instances of narrow artificial intelligence, systems that have been designed to perform specific tasks instead of having general problem-solving abilities. Some scientists believe that assembling multiple narrow AI modules will produce higher intelligent systems. For example, you can have a software system that coordinates between separate computer vision, voice processing, NLP, and motor control modules to solve complicated problems that require a multitude of skills.
A different approach to creating AI, proposed by the DeepMind researchers, is to recreate the simple yet effective rule that has given rise to natural intelligence. “[We] consider an alternative hypothesis: that the generic objective of maximising reward is enough to drive behaviour that exhibits most if not all abilities that are studied in natural and artificial intelligence,” the researchers write.
This is basically how nature works. As far as science is concerned, there has been no top-down intelligent design in the complex organisms that we see around us. Billions of years of natural selection and random variation have filtered lifeforms for their fitness to survive and reproduce. Living beings that were better equipped to handle the challenges and situations in their environments managed to survive and reproduce. The rest were eliminated.
This simple yet efficient mechanism has led to the evolution of living beings with all kinds of skills and abilities to perceive, navigate, modify their environments, and communicate among themselves.
“The natural world faced by animals and humans, and presumably also the environments faced in the future by artificial agents, are inherently so complex that they require sophisticated abilities in order to succeed (for example, to survive) within those environments,” the researchers write. “Thus, success, as measured by maximising reward, demands a variety of abilities associated with intelligence. In such environments, any behaviour that maximises reward must necessarily exhibit those abilities. In this sense, the generic objective of reward maximization contains within it many or possibly even all the goals of intelligence.”
For example, consider a squirrel that seeks the reward of minimizing hunger. On the one hand, its sensory and motor skills help it locate and collect nuts when food is available. But a squirrel that can only find food is bound to die of hunger when food becomes scarce. This is why it also has planning skills and memory to cache the nuts and restore them in winter. And the squirrel has social skills and knowledge to ensure other animals don’t steal its nuts. If you zoom out, hunger minimization can be a subgoal of “staying alive,” which also requires skills such as detecting and hiding from dangerous animals, protecting oneself from environmental threats, and seeking better habitats with seasonal changes.
“When abilities associated with intelligence arise as solutions to a singular goal of reward maximisation, this may in fact provide a deeper understanding since it explains why such an ability arises,” the researchers write. “In contrast, when each ability is understood as the solution to its own specialised goal, the why question is side-stepped in order to focus upon what that ability does.”
Finally, the researchers argue that the “most general and scalable” way to maximize reward is through agents that learn through interaction with the environment.
Developing abilities through reward maximization
In the paper, the AI researchers provide some high-level examples of how “intelligence and associated abilities will implicitly arise in the service of maximising one of many possible reward signals, corresponding to the many pragmatic goals towards which natural or artificial intelligence may be directed.”
For example, sensory skills serve the need to survive in complicated environments. Object recognition enables animals to detect food, prey, friends, and threats, or find paths, shelters, and perches. Image segmentation enables them to tell the difference between different objects and avoid fatal mistakes such as running off a cliff or falling off a branch. Meanwhile, hearing helps detect threats where the animal can’t see or find prey when they’re camouflaged. Touch, taste, and smell also give the animal the advantage of having a richer sensory experience of the habitat and a greater chance of survival in dangerous environments.
Rewards and environments also shape innate and learned knowledge in animals. For instance, hostile habitats ruled by predator animals such as lions and cheetahs reward ruminant species that have the innate knowledge to run away from threats since birth. Meanwhile, animals are also rewarded for their power to learn specific knowledge of their habitats, such as where to find food and shelter.
The researchers also discuss the reward-powered basis of language, social intelligence, imitation, and finally, general intelligence, which they describe as “maximising a singular reward in a single, complex environment.”
Here, they draw an analogy between natural intelligence and AGI: “An animal’s stream of experience is sufficiently rich and varied that it may demand a flexible ability to achieve a vast variety of subgoals (such as foraging, fighting, or fleeing), in order to succeed in maximising its overall reward (such as hunger or reproduction). Similarly, if an artificial agent’s stream of experience is sufficiently rich, then many goals (such as battery-life or survival) may implicitly require the ability to achieve an equally wide variety of subgoals, and the maximisation of reward should therefore be enough to yield an artificial general intelligence.”
Reinforcement learning for reward maximization

Reinforcement learning is a special branch of AI algorithms that is composed of three key elements: an environment, agents, and rewards.
By performing actions, the agent changes its own state and that of the environment. Based on how much those actions affect the goal the agent must achieve, it is rewarded or penalized. In many reinforcement learning problems, the agent has no initial knowledge of the environment and starts by taking random actions. Based on the feedback it receives, the agent learns to tune its actions and develop policies that maximize its reward.
In their paper, the researchers at DeepMind suggest reinforcement learning as the main algorithm that can replicate reward maximization as seen in nature and can eventually lead to artificial general intelligence.
“If an agent can continually adjust its behaviour so as to improve its cumulative reward, then any abilities that are repeatedly demanded by its environment must ultimately be produced in the agent’s behaviour,” the researchers write, adding that, in the course of maximizing for its reward, a good reinforcement learning agent could eventually learn perception, language, social intelligence and so forth.
In the paper, the researchers provide several examples that show how reinforcement learning agents were able to learn general skills in games and robotic environments.
However, the researchers stress that some fundamental challenges remain unsolved. For instance, they say, “We do not offer any theoretical guarantee on the sample efficiency of reinforcement learning agents.” Reinforcement learning is notoriously renowned for requiring huge amounts of data. For instance, a reinforcement learning agent might need centuries worth of gameplay to master a computer game. And AI researchers still haven’t figured out how to create reinforcement learning systems that can generalize their learnings across several domains. Therefore, slight changes to the environment often require the full retraining of the model.
The researchers also acknowledge that learning mechanisms for reward maximization is an unsolved problem that remains a central question to be further studied in reinforcement learning.
Strengths and weaknesses of reward maximization
Patricia Churchland, neuroscientist, philosopher, and professor emerita at the University of California, San Diego, described the ideas in the paper as “very carefully and insightfully worked out.”
However, Churchland pointed it out to possible flaws in the paper’s discussion about social decision-making. The DeepMind researchers focus on personal gains in social interactions. Churchland, who has recently written a book on the biological origins of moral intuitions, argues that attachment and bonding is a powerful factor in social decision-making of mammals and birds, which is why animals put themselves in great danger to protect their children.
“I have tended to see bonding, and hence other-care, as an extension of the ambit of what counts as oneself—‘me-and-mine,’” Churchland said. “In that case, a small modification to the [paper’s] hypothesis to allow for reward maximization to me-and-mine would work quite nicely, I think. Of course, we social animals have degrees of attachment—super strong to offspring, very strong to mates and kin, strong to friends and acquaintances etc., and the strength of types of attachments can vary depending on environment, and also on developmental stage.”
This is not a major criticism, Churchland said, and could likely be worked into the hypothesis quite gracefully.
“I am very impressed with the degree of detail in the paper, and how carefully they consider possible weaknesses,” Churchland said. “I may be wrong, but I tend to see this as a milestone.”
Data scientist Herbert Roitblat challenged the paper’s position that simple learning mechanisms and trial-and-error experience are enough to develop the abilities associated with intelligence. Roitblat argued that the theories presented in the paper face several challenges when it comes to implementing them in real life.
“If there are no time constraints, then trial and error learning might be enough, but otherwise we have the problem of an infinite number of monkeys typing for an infinite amount of time,” Roitblat said. The infinite monkey theorem states that a monkey hitting random keys on a typewriter for an infinite amount of time may eventually type any given text.
Roitblat is the author of Algorithms are Not Enough, in which he explains why all current AI algorithms, including reinforcement learning, require careful formulation of the problem and representations created by humans.
“Once the model and its intrinsic representation are set up, optimization or reinforcement could guide its evolution, but that does not mean that reinforcement is enough,” Roitblat said.
In the same vein, Roitblat added that the paper does not make any suggestions on how the reward, actions, and other elements of reinforcement learning are defined.
“Reinforcement learning assumes that the agent has a finite set of potential actions. A reward signal and value function have been specified. In other words, the problem of general intelligence is precisely to contribute those things that reinforcement learning requires as a pre-requisite,” Roitblat said. “So, if machine learning can all be reduced to some form of optimization to maximize some evaluative measure, then it must be true that reinforcement learning is relevant, but it is not very explanatory.”
Ben Dickson is a software engineer and the founder of TechTalks. He writes about technology, business, and politics.
This story originally appeared on Bdtechtalks.com. Copyright 2021
VentureBeat
VentureBeat's mission is to be a digital town square for technical decision-makers to gain knowledge about transformative technology and transact.
Our site delivers essential information on data technologies and strategies to guide you as you lead your organizations. We invite you to become a member of our community, to access:
- up-to-date information on the subjects of interest to you
- our newsletters
- gated thought-leader content and discounted access to our prized events, such as Transform 2021: Learn More
- networking features, and more
It’s time to move mission-critical applications to the cloud. Here’s how to do it right

Presented by Intel
Wild price changes. Fast-changing consumer demands. Shortages of everything from toilet paper to key industrial parts. The challenges of the Covid-19 pandemic — and the proven benefits and competitive advantage gained by organizations that forge ahead with digitalization — leave no doubt: It’s no longer a question of if or when, but how to best create a resilient, flexible foundation for business transformation including ERP, supply chain, and other bedrock technology.
The pandemic subjected enterprises worldwide to intense dual pressures. “Companies juggled between maintaining ‘business as usual’ operations and accelerating their digital roadmaps,” says Paul Cooper, chairman of the SAP UK and Ireland user group. A recent group survey found that 30% of organizations delayed their move to SAP S/4HANA ERP due to the pandemic. A similar U.S. poll found 18% had put plans on hold. Says Cooper: “It’s been an extremely challenging 12 months for most organizations.”
Enterprises, full speed ahead
Now, a McKinsey global survey reports that market leaders and laggards alike are moving full-speed ahead. The firm says the pandemic has accelerated digitization of customer and supply-chain interactions and internal operations by three to four years, and digital products in company portfolios by a “shocking” seven years. Researchers say the findings suggest executives recognize the technology’s strategic importance beyond cost efficiencies, including the value of speedy experimentation and innovation. They conclude: “Digital adoption has taken a quantum leap at both the organizational and industry levels.”

Above: Companies with decreasing revenues were most aggressive in stepping up focus on digital (top blue bar). Revenue leaders sped up “digitalization” efforts already underway (bottom black bar). Credit: McKinsey
A new report by Gartner predicts the focus on core applications will continue. The firm, which in 2018 projected that 80% of workloads would run in clouds, forecasts big spending increases on enterprise software in 2021 (10.8%) and 2022 (10.6%) — the highest two-year total of any category studied.
Cloud spending is getting a boost because emerging technologies such as containerization, virtualization, and edge computing are becoming more mainstream, explains Sid Nag, research vice president at Gartner. “The events of last year allowed CIOs to overcome any reluctance of moving mission-critical workloads from on-premises to the cloud,” Nag wrote in the forecast.
For many organizations worldwide, “enterprise” and “business-critical” applications means SAP and Intel. Some 92% of the Forbes Global 2000 companies are SAP customers; more than 90% of the world’s cloud computing is powered by Intel.
As organizations mobilize for a massive global effort to produce and distribute COVID-19 vaccinations and “return to normal,” SaaS-based applications that enable essential tasks such as automation and supply chain are critical. That these applications demonstrate reliability in scaling vaccine management will help CIOs validate the ongoing shift to cloud, Gartner says.
Many paths to many benefits
Whether the roadmap is to migrate, convert, rehost, re-platform or re-architect, enterprises seek similar benefits from running business-critical software in the cloud.
Both existing “brown-field” and new “green-field” deployments can cut costs and increase global agility and transformational business value. To this end, pilot and production projects are now accelerating. The goals are to drive enhanced performance and agility, lower TCO and better ROI, better security and standardization by integrating, simplifying, and modernizing core digital infrastructure and applications. Many are working to build an “Intelligent Enterprise” to unlock the value of data and better drive split-second decisions and actions.

Above: While migrating core applications is complex and carries risks, the rewards are significant. Credit: Intel
But many business and technology leaders are unsure about the best path for deploying SAP HANA in-memory databases, a cornerstone of ERP, and other highly demanding core technology for cloud-based transformation. To get the best performance and ROI from these key foundations requires two key things: Optimizing performance and operation, and optimizing deployment.
Key 1: Optimizing performance and operation
For many reasons, it’s very difficult for enterprises to handle today’s workloads without optimization of applications and infrastructure. Chief among them: The growing complexity of use requirements and multi-tier data, with huge increases in data velocity and volume. Other factors include workloads that need more, larger nodes as they scale up, and the demands of high availability/disaster recovery (HA/DR).
New technologies also have upped requirements. For example, modern ERP systems must include advanced analytics on live transactions and real-time operational reporting. Complex predictions are now derived from broad and diverse datasets, such as the contextual data analysis of massive volumes of IoT sensor data or detailed geospatial and graph analyses. And AI and ML need fast, sophisticated language processing to automatically respond to user behavior.
The solution: Technology that more tightly integrates and collaborates across the traditional layers of the IT stack. Apps don’t just rely on the OS; they also talk directly to the chips to take advantage of more specialized features. This whole-stack approach is needed to address performance, resilience, and cost management challenges.
Intel and SAP: Not all critical cloud instances are created equal
Only software and systems tuned for top performance can deliver the best benefits.Unfortunately, not every Cloud Service Provider (CSP) does this equally well or broadly across a wide range of SAP applications. It’s not about simply fielding servers powered by chips with the most raw power; the specific silicon matters here. So does, crucially, whether your provider offers optimized SAP instances and infrastructures.
That’s the latest focus of the newly expanded partnership between Intel and SAP. These industry leaders are offering optimized cloud instances to ensure optimal performance of SAP HANA and SAP HANA based applications, like S/4HANA ERP.
A multi-year effort with cloud providers and ecosystem partners is developing new ways to help enterprises get the most from their investments though high-performance, highly optimized SAP cloud applications. At the core is tight integration and tuning of SAP HANA and Intel Xeon Scalable processors and Optane persistent memory (PMem). Key goals are to provide best-in-class performance, reduce operational risk through improved resilience, and deliver open and extensible frameworks.
Maximizing the most-wanted benefits
Industry benchmarks and real-world user results provide striking proof that the latest advances outperform unoptimized and previous instances of SAP cloud applications. These gains are crucial to getting the most from your deployments, so pay special attention to each as you evaluate solutions and providers.
Performance. Faster processing of much bigger volumes of data is at the core of high-performance cloud-based critical applications. The latest Intel Xeon Scalable processors offer 50% higher performance and higher memory-to-processor ratio than previous generations. Coupling with Optane PMem enables performance far surpassing DRAM and conventional storage. (That’s why all SAP HANA speed records are on Intel platforms.)
At BP, thousands of employees worldwide used multiple SAP applications for supply chain, procurement, and other essential functions. But the global energy supplier was not fully utilizing its infrastructure, and getting a new project up and running took months, according to Steve Fortune, group CIO.
A pilot project that moved one division’s central SAP ERP production system to Amazon Web Services (AWS) yielded a 40% performance improvement, thanks to the ability of optimized instances to handle workloads. What’s more, Fortune says, new business initiatives are now much faster rolling out. And the company has realized big savings in annual licensing, hardware, support, and maintenance costs. Based on this success, BP is moving its entire SAP landscape into AWS. Explains Fortune: “BP needs the agility to be competitive when prices, policy, technology, and customer preferences are changing.”
Agility. The ability of cloud and digital architectures to quickly scale and adapt to changing conditions becomes even more important takes in enterprise cloud applications. For example, when Covid-19 hit, Rémy Cointreau Group needed to adjust quickly to support direct-to-consumer sales, while preparing for an influx of commercial orders once bars and restaurants start opening again, explains CTO Sébastien Huet.
Ironically, the pandemic struck just before the planned go-live date of a major SAP deployment on Google Cloud, forcing team members to finish working remotely in different corners of the world. Huet says the migration was a huge, timely advantage. “The Covid-19 pandemic has shown us the importance of agility,” he says. “Having the flexibility to make a decision even an hour earlier can mean the difference between whether or not a shipment makes it to China in time for a holiday.” With people staying at home, ecommerce sales for the global wine and spirits maker have doubled, the company reports.
At TomTom, running SAP on Azure has cut the time needed to spin up new systems from weeks to hours, says Ron Hogeboom, senior manager IT, Finance and Logistics, increasing agility with much less staff time. Spending less time on monitoring, maintenance, upgrades, troubleshooting, and backups also can help reduce IT administrative costs, he notes.
Business continuity and resilience. Because critical workloads are deeply intertwined with key business processes, downtime or performance hits are massively disruptive. So how fast a system restarts after a shutdown, planned or unplanned, is crucial. Data stored in Intel Optane PMem is persistent, meaning it stays in memory even if there’s no power. So when an SAP HANA instance needs to reboot, there’s no need to reload data from disks or slower storage tiers. That enables a dramatically faster return to normal operations compared to DRAM-only systems. Speedier reload and recovery times can shorten service windows and downtime for upgrades or patches.
Running SAP S/4HANA on Microsoft Azure has greatly helped Walgreens Boots Alliance overcome business continuity challenges, says Francesco Tinto, senior vice president, global chief information officer. The optimized system provides “real-time visibility into our inventory, which is crucial for us as a pharmacy and health care retail company during the pandemic,” he says. Being able to access all data also lets the company “offer the best possible customer experience online and in stores.”

Above: Credit: Intel
TCO / ROI. Moving to an SAP S/4 HANA Cloud private edition from traditional ERP yields a 20% reduction in TCO over five years, according to Gartner. Platform consolidation and simplification yield big savings. Here’s why: The higher system memory density (up 6x for analytical workloads, 3x for transactional) of new Intel Xeon chips and Intel Optane PMem enable scale-up or scale-out with fewer servers and instances of SAP HANA, with much lower cost-per-terabyte processed. That, in turn, helps organizations consolidate platform footprint and reduce operational complexity and costs.
One of the world’s leading providers of electric utilities and renewable energy, Engie planned to integrate dozens of group ERP systems worldwide. The goal, says Finance division CIO Thierry Langer, was to help drive better insight with agility, data, measure business performance, and reduce costs. The company decided to modernize and migrate to SAP S/4HANA, which would require a new hosting provider. They chose AWS EC2 X1e instances powered by Intel Xeon processors. From initial decision-making to go-live, the project took approximately six months. As a result of migrating to the new technology, Langers says, Engie’s secondary database shrank from 4 TB to around 200 GB, dramatically reducing the company’s storage footprint. Ramp-up time for new users has been reduced from three days to one. Overall, Langer says of the move, “We feel it is an enormous advantage.”
Combined, these savings are a big reason why SAP HANA on Intel platforms offers 53% better TCO compared to IBM Power-based solutions.
Security and compliance. Intel technology provides a scalable, security-enabled foundation for SAP workloads via built-in, hardware-enhanced features. Take the Intel SGX safeguard extensions on the new Intel Xeon processor. These encrypt and keep data in the wallet, then safely encrypted into the server at the CPU level to perform collective risk calculation, for end-to-end protection. Intel TSX improves lock scalability as users scale with multi-user concurrent usages in the cloud. For cloud-based instances, where mission- critical information leaves the traditional IT environment, a more widely usable and secure encryption standard such as Intel AES New Instructions (Intel AES-NI) protects data in flight and at rest. While no product or component can be absolutely secure, these and other technologies help provide crucial privacy and security for highly sensitive data that are the lifeblood of enterprise systems.
Key 2: Optimizing deployment
Choosing an optimized, high-performing SAP instance is the first key to success. To fully capitalize, though, enterprises also must thoughtfully plan and manage deployment in the fastest, surest way that matches their unique starting point and needs.
Because of their centrality and importance, rolling out core enterprise applications in the cloud can be highly complex. Many have extensive customizations, which must be migrated or subsumed. While “cloudification” ultimately can simplify operations, it may temporarily present new complexity. And business transformation isn’t limited to applications and infrastructure: It also includes major changes to business processes and finances (Opex vs Capex, chargebacks, licensing, etc.). Eliminating roadblocks in all these areas is crucial to realizing the opportunity of the intelligent enterprise.
And of course, digitalization and business transformation are not “one-and-done’s,” but ongoing ventures. So it’s important to engage with vendors and suppliers who can provide a variety of products and services that evolve with your enterprise. Again, here’s where the Intel-SAP end-to-end ecosystem provides a huge advantage. Industry validation and adoption of optimized SAP cloud applications and infrastructure has been strong.
Flexibility, standardization, future proofing
Certified, pre-validated SAP HANA instances are standard but flexible building blocks. They make it simpler to grow modern, mission-critical cloud infrastructure across diverse geographies and networks around the world. Co-development by SAP and Intel and a large, best-in-class ecosystem accelerates innovation while reducing risk.
Many organizations will work with hyperscale CSPs to simplify and integrate core systems. Microsoft Azure, Alibaba Cloud, Amazon Web Services, Google Cloud Platform, IBM Cloud, and others now offer SAP-certified infrastructure-as-a-service (IaaS) and purpose-built capabilities for supporting optimized SAP HANA workloads.
Intel has developed a six-step process to help enterprises choose the best deployment model for their current state. Based on user experience, this systematic approach guides decision-makers through the selection of private, public, hybrid, virtual, or “bare-metal” cloud instances of SAP HANA and other technology anchors of the intelligent enterprise.
In January, SAP introduced “Business Transformation as a Service” or BTaaS. RISE with SAP helps enterprises with process redesign and technology migrations. It can extend and integrate with any SAP, partner, or third-party solution, both in the cloud and on-premise. RISE expands offerings by Accenture, Deloitte, and others providing SAP migration and digital transformation services.
Finally, more than a dozen top top OEMs including Dell and Cisco have signed on. Many have rolled out managed hybrid cloud as a service, with flexible, consumption-based billing models. Innovative new offerings like HPE GreenLake and Lenovo TruScale provide solution, implementation, and support for optimized SAP cloud applications and infrastructure. Software partners like Red Hat, VMware, and Suse deliver complementary, optimized solutions. All told, more than 1,300 appliances and tailored datacenter integrations (TDI’s) help protect buyers against vendor lock-in.
Bottom line: Worth doing right
The global disruptions of Covid-19 were a reminder and wake up call. Even when the pandemic subsides, no one predicts an end to volatility, rapid change, and data growth, forecast by IDC at 25% CAGR through 2024. Companies in every industry must deploy resilient and optimized mission-critical cloud systems to respond to dynamic new demands. SAP, Intel, and ecosystem partners continue to advance the performance, agility, continuity, security, and TCO needed to deliver benefits today and position enterprises for success tomorrow.
Go deeper:
Sponsored articles are content produced by a company that is either paying for the post or has a business relationship with VentureBeat, and they’re always clearly marked. Content produced by our editorial team is never influenced by advertisers or sponsors in any way. For more information, contact [email protected].
Recommend
About Joyk
Aggregate valuable and interesting links.
Joyk means Joy of geeK