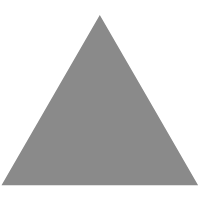
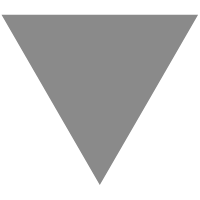
Pandas高级教程之:Dataframe的重排和旋转
source link: http://www.flydean.com/05-python-pandas-reshaping-pivot/
Go to the source link to view the article. You can view the picture content, updated content and better typesetting reading experience. If the link is broken, please click the button below to view the snapshot at that time.
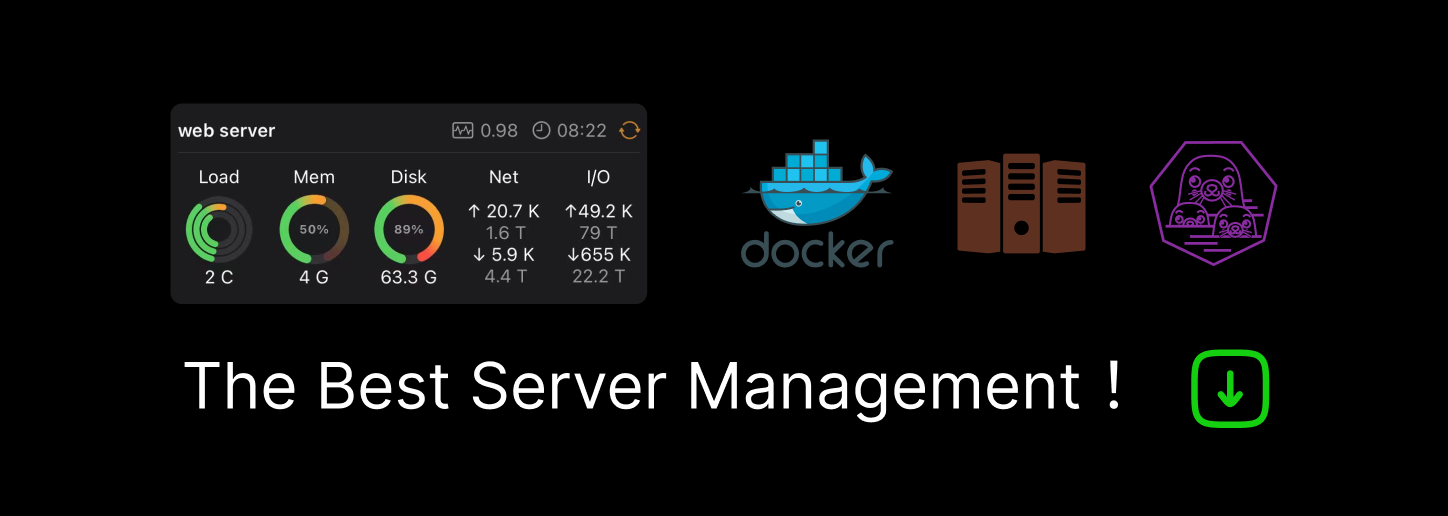
使用Pandas的pivot方法可以将DF进行旋转变换,本文将会详细讲解pivot的秘密。
使用Pivot
pivot用来重组DF,使用指定的index,columns和values来对现有的DF进行重构。
看一个Pivot的例子:
通过pivot变化,新的DF使用foo中的值作为index,使用bar的值作为columns,zoo作为对应的value。
再看一个时间变化的例子:
In [1]: df
Out[1]:
date variable value
0 2000-01-03 A 0.469112
1 2000-01-04 A -0.282863
2 2000-01-05 A -1.509059
3 2000-01-03 B -1.135632
4 2000-01-04 B 1.212112
5 2000-01-05 B -0.173215
6 2000-01-03 C 0.119209
7 2000-01-04 C -1.044236
8 2000-01-05 C -0.861849
9 2000-01-03 D -2.104569
10 2000-01-04 D -0.494929
11 2000-01-05 D 1.071804
In [3]: df.pivot(index='date', columns='variable', values='value')
Out[3]:
variable A B C D
date
2000-01-03 0.469112 -1.135632 0.119209 -2.104569
2000-01-04 -0.282863 1.212112 -1.044236 -0.494929
2000-01-05 -1.509059 -0.173215 -0.861849 1.071804
如果剩余的value,多于一列的话,每一列都会有相应的columns值:
In [4]: df['value2'] = df['value'] * 2
In [5]: pivoted = df.pivot(index='date', columns='variable')
In [6]: pivoted
Out[6]:
value value2
variable A B C D A B C D
date
2000-01-03 0.469112 -1.135632 0.119209 -2.104569 0.938225 -2.271265 0.238417 -4.209138
2000-01-04 -0.282863 1.212112 -1.044236 -0.494929 -0.565727 2.424224 -2.088472 -0.989859
2000-01-05 -1.509059 -0.173215 -0.861849 1.071804 -3.018117 -0.346429 -1.723698 2.143608
通过选择value2,可以得到相应的子集:
In [7]: pivoted['value2']
Out[7]:
variable A B C D
date
2000-01-03 0.938225 -2.271265 0.238417 -4.209138
2000-01-04 -0.565727 2.424224 -2.088472 -0.989859
2000-01-05 -3.018117 -0.346429 -1.723698 2.143608
使用Stack
Stack是对DF进行转换,将列转换为新的内部的index。
上面我们将列A,B转成了index。
unstack是stack的反向操作,是将最内层的index转换为对应的列。
举个具体的例子:
In [8]: tuples = list(zip(*[['bar', 'bar', 'baz', 'baz',
...: 'foo', 'foo', 'qux', 'qux'],
...: ['one', 'two', 'one', 'two',
...: 'one', 'two', 'one', 'two']]))
...:
In [9]: index = pd.MultiIndex.from_tuples(tuples, names=['first', 'second'])
In [10]: df = pd.DataFrame(np.random.randn(8, 2), index=index, columns=['A', 'B'])
In [11]: df2 = df[:4]
In [12]: df2
Out[12]:
A B
first second
bar one 0.721555 -0.706771
two -1.039575 0.271860
baz one -0.424972 0.567020
two 0.276232 -1.087401
In [13]: stacked = df2.stack()
In [14]: stacked
Out[14]:
first second
bar one A 0.721555
B -0.706771
two A -1.039575
B 0.271860
baz one A -0.424972
B 0.567020
two A 0.276232
B -1.087401
dtype: float64
默认情况下unstack是unstack最后一个index,我们还可以指定特定的index值:
In [15]: stacked.unstack()
Out[15]:
A B
first second
bar one 0.721555 -0.706771
two -1.039575 0.271860
baz one -0.424972 0.567020
two 0.276232 -1.087401
In [16]: stacked.unstack(1)
Out[16]:
second one two
first
bar A 0.721555 -1.039575
B -0.706771 0.271860
baz A -0.424972 0.276232
B 0.567020 -1.087401
In [17]: stacked.unstack(0)
Out[17]:
first bar baz
second
one A 0.721555 -0.424972
B -0.706771 0.567020
two A -1.039575 0.276232
B 0.271860 -1.087401
默认情况下stack只会stack一个level,还可以传入多个level:
In [23]: columns = pd.MultiIndex.from_tuples([
....: ('A', 'cat', 'long'), ('B', 'cat', 'long'),
....: ('A', 'dog', 'short'), ('B', 'dog', 'short')],
....: names=['exp', 'animal', 'hair_length']
....: )
....:
In [24]: df = pd.DataFrame(np.random.randn(4, 4), columns=columns)
In [25]: df
Out[25]:
exp A B A B
animal cat cat dog dog
hair_length long long short short
0 1.075770 -0.109050 1.643563 -1.469388
1 0.357021 -0.674600 -1.776904 -0.968914
2 -1.294524 0.413738 0.276662 -0.472035
3 -0.013960 -0.362543 -0.006154 -0.923061
In [26]: df.stack(level=['animal', 'hair_length'])
Out[26]:
exp A B
animal hair_length
0 cat long 1.075770 -0.109050
dog short 1.643563 -1.469388
1 cat long 0.357021 -0.674600
dog short -1.776904 -0.968914
2 cat long -1.294524 0.413738
dog short 0.276662 -0.472035
3 cat long -0.013960 -0.362543
dog short -0.006154 -0.923061
上面等价于:
In [27]: df.stack(level=[1, 2])
使用melt
melt指定特定的列作为标志变量,其他的列被转换为行的数据。并放置在新的两个列:variable和value中。
上面例子中我们指定了两列first和last,这两列是不变的,height和weight被变换成为行数据。
举个例子:
In [41]: cheese = pd.DataFrame({'first': ['John', 'Mary'],
....: 'last': ['Doe', 'Bo'],
....: 'height': [5.5, 6.0],
....: 'weight': [130, 150]})
....:
In [42]: cheese
Out[42]:
first last height weight
0 John Doe 5.5 130
1 Mary Bo 6.0 150
In [43]: cheese.melt(id_vars=['first', 'last'])
Out[43]:
first last variable value
0 John Doe height 5.5
1 Mary Bo height 6.0
2 John Doe weight 130.0
3 Mary Bo weight 150.0
In [44]: cheese.melt(id_vars=['first', 'last'], var_name='quantity')
Out[44]:
first last quantity value
0 John Doe height 5.5
1 Mary Bo height 6.0
2 John Doe weight 130.0
3 Mary Bo weight 150.0
使用Pivot tables
虽然Pivot可以进行DF的轴转置,Pandas还提供了 pivot_table() 在转置的同时可以进行数值的统计。
pivot_table() 接收下面的参数:
data: 一个df对象
values:一列或者多列待聚合的数据。
Index: index的分组对象
Columns: 列的分组对象
Aggfunc: 聚合的方法。
先创建一个df:
In [59]: import datetime
In [60]: df = pd.DataFrame({'A': ['one', 'one', 'two', 'three'] * 6,
....: 'B': ['A', 'B', 'C'] * 8,
....: 'C': ['foo', 'foo', 'foo', 'bar', 'bar', 'bar'] * 4,
....: 'D': np.random.randn(24),
....: 'E': np.random.randn(24),
....: 'F': [datetime.datetime(2013, i, 1) for i in range(1, 13)]
....: + [datetime.datetime(2013, i, 15) for i in range(1, 13)]})
....:
In [61]: df
Out[61]:
A B C D E F
0 one A foo 0.341734 -0.317441 2013-01-01
1 one B foo 0.959726 -1.236269 2013-02-01
2 two C foo -1.110336 0.896171 2013-03-01
3 three A bar -0.619976 -0.487602 2013-04-01
4 one B bar 0.149748 -0.082240 2013-05-01
.. ... .. ... ... ... ...
19 three B foo 0.690579 -2.213588 2013-08-15
20 one C foo 0.995761 1.063327 2013-09-15
21 one A bar 2.396780 1.266143 2013-10-15
22 two B bar 0.014871 0.299368 2013-11-15
23 three C bar 3.357427 -0.863838 2013-12-15
[24 rows x 6 columns]
下面是几个聚合的例子:
In [62]: pd.pivot_table(df, values='D', index=['A', 'B'], columns=['C'])
Out[62]:
C bar foo
A B
one A 1.120915 -0.514058
B -0.338421 0.002759
C -0.538846 0.699535
three A -1.181568 NaN
B NaN 0.433512
C 0.588783 NaN
two A NaN 1.000985
B 0.158248 NaN
C NaN 0.176180
In [63]: pd.pivot_table(df, values='D', index=['B'], columns=['A', 'C'], aggfunc=np.sum)
Out[63]:
A one three two
C bar foo bar foo bar foo
B
A 2.241830 -1.028115 -2.363137 NaN NaN 2.001971
B -0.676843 0.005518 NaN 0.867024 0.316495 NaN
C -1.077692 1.399070 1.177566 NaN NaN 0.352360
In [64]: pd.pivot_table(df, values=['D', 'E'], index=['B'], columns=['A', 'C'],
....: aggfunc=np.sum)
....:
Out[64]:
D E
A one three two one three two
C bar foo bar foo bar foo bar foo bar foo bar foo
B
A 2.241830 -1.028115 -2.363137 NaN NaN 2.001971 2.786113 -0.043211 1.922577 NaN NaN 0.128491
B -0.676843 0.005518 NaN 0.867024 0.316495 NaN 1.368280 -1.103384 NaN -2.128743 -0.194294 NaN
C -1.077692 1.399070 1.177566 NaN NaN 0.352360 -1.976883 1.495717 -0.263660 NaN NaN 0.872482
添加margins=True会添加一个All列,表示对所有的列进行聚合:
In [69]: df.pivot_table(index=['A', 'B'], columns='C', margins=True, aggfunc=np.std)
Out[69]:
D E
C bar foo All bar foo All
A B
one A 1.804346 1.210272 1.569879 0.179483 0.418374 0.858005
B 0.690376 1.353355 0.898998 1.083825 0.968138 1.101401
C 0.273641 0.418926 0.771139 1.689271 0.446140 1.422136
three A 0.794212 NaN 0.794212 2.049040 NaN 2.049040
B NaN 0.363548 0.363548 NaN 1.625237 1.625237
C 3.915454 NaN 3.915454 1.035215 NaN 1.035215
two A NaN 0.442998 0.442998 NaN 0.447104 0.447104
B 0.202765 NaN 0.202765 0.560757 NaN 0.560757
C NaN 1.819408 1.819408 NaN 0.650439 0.650439
All 1.556686 0.952552 1.246608 1.250924 0.899904 1.059389
使用crosstab
Crosstab 用来统计表格中元素的出现次数。
In [70]: foo, bar, dull, shiny, one, two = 'foo', 'bar', 'dull', 'shiny', 'one', 'two'
In [71]: a = np.array([foo, foo, bar, bar, foo, foo], dtype=object)
In [72]: b = np.array([one, one, two, one, two, one], dtype=object)
In [73]: c = np.array([dull, dull, shiny, dull, dull, shiny], dtype=object)
In [74]: pd.crosstab(a, [b, c], rownames=['a'], colnames=['b', 'c'])
Out[74]:
b one two
c dull shiny dull shiny
a
bar 1 0 0 1
foo 2 1 1 0
crosstab可以接收两个Series:
In [75]: df = pd.DataFrame({'A': [1, 2, 2, 2, 2], 'B': [3, 3, 4, 4, 4],
....: 'C': [1, 1, np.nan, 1, 1]})
....:
In [76]: df
Out[76]:
A B C
0 1 3 1.0
1 2 3 1.0
2 2 4 NaN
3 2 4 1.0
4 2 4 1.0
In [77]: pd.crosstab(df['A'], df['B'])
Out[77]:
B 3 4
A
1 1 0
2 1 3
还可以使用normalize来指定比例值:
In [82]: pd.crosstab(df['A'], df['B'], normalize=True)
Out[82]:
B 3 4
A
1 0.2 0.0
2 0.2 0.6
还可以normalize行或者列:
In [83]: pd.crosstab(df['A'], df['B'], normalize='columns')
Out[83]:
B 3 4
A
1 0.5 0.0
2 0.5 1.0
可以指定聚合方法:
In [84]: pd.crosstab(df['A'], df['B'], values=df['C'], aggfunc=np.sum)
Out[84]:
B 3 4
A
1 1.0 NaN
2 1.0 2.0
get_dummies
get_dummies可以将DF中的一列转换成为k列的0和1组合:
df = pd.DataFrame({'key': list('bbacab'), 'data1': range(6)})
df
Out[9]:
data1 key
0 0 b
1 1 b
2 2 a
3 3 c
4 4 a
5 5 b
pd.get_dummies(df['key'])
Out[10]:
a b c
0 0 1 0
1 0 1 0
2 1 0 0
3 0 0 1
4 1 0 0
5 0 1 0
get_dummies 和 cut 可以进行结合用来统计范围内的元素:
In [95]: values = np.random.randn(10)
In [96]: values
Out[96]:
array([ 0.4082, -1.0481, -0.0257, -0.9884, 0.0941, 1.2627, 1.29 ,
0.0824, -0.0558, 0.5366])
In [97]: bins = [0, 0.2, 0.4, 0.6, 0.8, 1]
In [98]: pd.get_dummies(pd.cut(values, bins))
Out[98]:
(0.0, 0.2] (0.2, 0.4] (0.4, 0.6] (0.6, 0.8] (0.8, 1.0]
0 0 0 1 0 0
1 0 0 0 0 0
2 0 0 0 0 0
3 0 0 0 0 0
4 1 0 0 0 0
5 0 0 0 0 0
6 0 0 0 0 0
7 1 0 0 0 0
8 0 0 0 0 0
9 0 0 1 0 0
get_dummies还可以接受一个DF参数:
In [99]: df = pd.DataFrame({'A': ['a', 'b', 'a'], 'B': ['c', 'c', 'b'],
....: 'C': [1, 2, 3]})
....:
In [100]: pd.get_dummies(df)
Out[100]:
C A_a A_b B_b B_c
0 1 1 0 0 1
1 2 0 1 0 1
2 3 1 0 1 0
本文已收录于 http://www.flydean.com/05-python-pandas-reshaping-pivot/
最通俗的解读,最深刻的干货,最简洁的教程,众多你不知道的小技巧等你来发现!
欢迎关注我的公众号:「程序那些事」,懂技术,更懂你!
Recommend
-
11
Pandas之:Pandas高级教程以铁达尼号真实数据为例 2021-06-03 Pandas之:Pandas高级教程以铁达尼号真实数据为例 今天我们会讲解一下Pandas的高级教程,包括读写文件、...
-
8
Pandas高级教程之:Dataframe的合并 2021-06-08 Pandas提供了很多合并Series和Dataframe的强大的功能,通过这些功能可以方便的进行数据分析。本文将会详细讲解如何使用...
-
8
Pandas中有一种特殊的数据类型叫做category。它表示的是一个类别,一般用在统计分类中,比如性别,血型,分类,级别等等。有点像java中的enum。 今天给大家详细讲解一下category的用法。 创建category 使用Series创建 在创建Se...
-
7
在数据处理中,Pandas会将无法解析的数据或者缺失的数据使用NaN来表示。虽然所有的数据都有了相应的表示,但是NaN很明显是无法进行数学运算的。 本文将会讲解Pandas对于NaN数据的处理方法。 NaN的例子 上面讲到了缺失的数据会被表现为Na...
-
7
在1.0之前,只有一种形式来存储text数据,那就是object。在1.0之后,添加了一个新的数据类型叫做StringDtype 。今天将会给大家讲解Pandas中text中的那些事。 创建text的DF 先看下常见的使用text来构建DF的例子: In [1]: pd.Ser...
-
1
数据分析中经常会用到很多统计类的方法,本文将会介绍Pandas中使用到的统计方法。 变动百分百 Series和DF都有一个pct_change() 方法用来计算数据变动的百分比。这个方法在填充NaN值的时候特别有用。 ser = pd.Seri...
-
7
Pandas高级教程之:GroupBy用法 Pandas高级教程之:GroupBy用法 pandas中的DF数据类型可以像数据库表格一样进行groupby操作。通常来说groupby操作可以分为三部分:分割数据,应用变换和和合并数据。 本文将会详细讲解Pandas中的gr...
-
9
Pandas高级教程之:plot画图详解 python中matplotlib是非常重要并且方便的图形化工具,使用matplotlib可以可视化的进行数据分析,今天本文将会详细讲解Pandas中的matplotlib应用。 要想使用matplotlib,我们需要引用它: I...
-
5
如果数据中有很多NaN的值,存储起来就会浪费空间。为了解决这个问题,Pandas引入了一种叫做Sparse data的结构,来有效的存储这些NaN的值。 Spare data的例子 我们创建一个数组,然后将其大部分数据设置为NaN,接着使用这个数组来创建SparseArra...
-
10
Pandas高级教程之:自定义选项 pandas有一个option系统可以控制pandas的展示情况,一般来说我们不需要进行修改,但是不排除特殊情况下的修改需求。本文将会详细讲解pandas中的option设置。 pd.options.display 可以控制展示选项,比如设置...
About Joyk
Aggregate valuable and interesting links.
Joyk means Joy of geeK