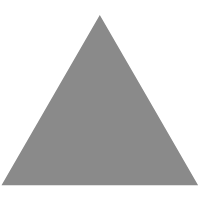
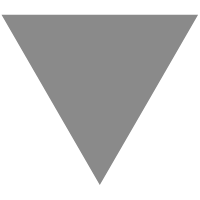
【资源】超分辨率相关资源大列表
source link: https://bbs.cvmart.net/articles/396
Go to the source link to view the article. You can view the picture content, updated content and better typesetting reading experience. If the link is broken, please click the button below to view the snapshot at that time.
作者:ChaofWang
来源:ChaofWang/Awesome-Super-Resolution
分享一个超分辨率相关资源列表,含图像和视频超分辨,内容包括相关论文、数据集、资料库等。
Awesome-Super-Resolution(in progress)
repositories
Awesome paper list:
Awesome repos:
Datasets
Note this table is referenced from here.
Name Usage Link Comments Set5 Test download jbhuang0604 SET14 Test download jbhuang0604 BSD100 Test download jbhuang0604 Urban100 Test download jbhuang0604 Manga109 Test website
SunHay80 Test download jbhuang0604 BSD300 Train/Val download
BSD500 Train/Val download
91-Image Train download Yang DIV2K2017 Train/Val website NTIRE2017 Real SR Train/Val website NTIRE2019 Waterloo Train website
VID4 Test download 4 videos MCL-V Train website 12 videos GOPRO Train/Val website 33 videos, deblur CelebA Train website Human faces Sintel Train/Val website Optical flow FlyingChairs Train website Optical flow Vimeo-90k Train/Test website 90k HQ videos
Dataset collections
Benckmark and DIV2K: Set5, Set14, B100, Urban100, Manga109, DIV2K2017 include bicubic downsamples with x2,3,4,8
SR_testing_datasets: Test: Set5, Set14, B100, Urban100, Manga109, Historical; Train: T91,General100, BSDS200
paper
Non-DL based approach
SCSR: TIP2010, Jianchao Yang et al.paper, code
ANR: ICCV2013, Radu Timofte et al. paper, code
A+: ACCV 2014, Radu Timofte et al. paper, code
IA: CVPR2016, Radu Timofte et al. paper
SelfExSR: CVPR2015, Jia-Bin Huang et al. paper, code
NBSRF: ICCV2015, Jordi Salvador et al. paper
RFL: ICCV2015, Samuel Schulter et al paper, code
DL based approach
Note this table is referenced from here
Model Published Code Keywords SRCNN ECCV14 Keras Kaiming RAISR arXiv - Google, Pixel 3 ESPCN CVPR16 Keras Real time/SISR/VideoSR VDSR CVPR16 Matlab Deep, Residual DRCN CVPR16 Matlab Recurrent DRRN CVPR17 Caffe, PyTorch Recurrent LapSRN CVPR17 Matlab Huber loss IRCNN CVPR17 Matlab
EDSR CVPR17 PyTorch NTIRE17 Champion BTSRN CVPR17 - NTIRE17 SelNet CVPR17 - NTIRE17 TLSR CVPR17 - NTIRE17 SRGAN CVPR17 Tensorflow 1st proposed GAN VESPCN CVPR17 - VideoSR MemNet ICCV17 Caffe
SRDenseNet ICCV17 -, PyTorch Dense SPMC ICCV17 Tensorflow VideoSR EnhanceNet ICCV17 TensorFlow Perceptual Loss PRSR ICCV17 TensorFlow an extension of PixelCNN AffGAN ICLR17 -
MS-LapSRN TPAMI18 Matlab Fast LapSRN DCSCN arXiv Tensorflow
IDN CVPR18 Caffe Fast DSRN CVPR18 TensorFlow Dual state,Recurrent RDN CVPR18 Torch Deep, BI-BD-DN SRMD CVPR18 Matlab Denoise/Deblur/SR DBPN CVPR18 PyTorch NTIRE18 Champion WDSR CVPR18 PyTorch,TensorFlow NTIRE18 Champion ProSRN CVPR18 PyTorch NTIRE18 ZSSR CVPR18 Tensorflow Zero-shot FRVSR CVPR18 PDF VideoSR DUF CVPR18 Tensorflow VideoSR TDAN arXiv - VideoSR,Deformable Align SFTGAN CVPR18 PyTorch
CARN ECCV18 PyTorch Lightweight RCAN ECCV18 PyTorch Deep, BI-BD-DN MSRN ECCV18 PyTorch
SRFeat ECCV18 Tensorflow GAN ESRGAN ECCV18 PyTorch PRIM18 region 3 Champion FEQE ECCV18 Tensorflow Fast NLRN NIPS18 Tensorflow Non-local, Recurrent SRCliqueNet NIPS18 - Wavelet CBDNet arXiv Matlab Blind-denoise TecoGAN arXiv Tensorflow VideoSR GAN RBPN CVPR19 PyTorch VideoSR SRFBN CVPR19 PyTorch Feedback MoreMNAS arXiv - Lightweight,NAS FALSR arXiv TensorFlow Lightweight,NAS Meta-SR arXiv
Arbitrary Magnification AWSRN arXiv PyTorch Lightweight OISR CVPR19 PyTorch ODE-inspired Network DPSR CVPR19 PyTorch
MAANet arXiv
Multi-view Aware Attention RNAN ICLR19 PyTorch Residual Non-local Attention FSTRN CVPR19 - VideoSR, fast spatio-temporal residual block MsDNN arXiv TensorFlow NTIRE19 real SR 21th place
Super Resolution survey:
[1] Wenming Yang, Xuechen Zhang, Yapeng Tian, Wei Wang, Jing-Hao Xue. Deep Learning for Single Image Super-Resolution: A Brief Review. arxiv, 2018. paper
[2]Saeed Anwar, Salman Khan, Nick Barnes. A Deep Journey into Super-resolution: A survey. arxiv, 2019.paper
微信公众号: 极市平台(ID: extrememart )
每天推送最新CV干货
Recommend
-
7
【资源】行为识别相关资源列表 1年前 ⋅...
-
9
【资源】Zero-Shot Learning 相关资源大列表 1年前 ⋅...
-
14
【资源】弱监督语义分割 state-or-art 资源列表 1年前 ⋅...
-
7
【资源】视频增强相关资源列表 1年前 ⋅...
-
2
之前逛爱可可老师微博看到的一个人脸识别资源,还是比较全面的,跟大家分享一下。 github链接:https://github.com/ChanChiChoi/awesome-Face_Recognition 作者:ChanChiCh...
-
6
作者:Rheelt 项目:Materials-Temporal-Action-Detection 本文汇总了时序行为检测相关的资源列表 更多Awsome Github资源请关注:【Awsome】G...
-
6
【资源】时尚 +AI 文献、资源大列表 1年前 ⋅...
-
5
【资源】全景分割相关资源大列表 1年前 ⋅...
-
9
V2EX › Windows Win10 资源管理器里面的快速访问列表,文件夹后面的别针按钮怎么去掉啊
-
4
【资源】图神经网络 (GNN) 相关资源大列表精选 技术讨论 Find me · 发表于 2019-05-21 10:33:16 文章来源: Awsome-Github 资源列表 最近,图神经网络 (GNN) 在各个领域越来越受到欢迎,包括计算机视觉、社交网络、知...
About Joyk
Aggregate valuable and interesting links.
Joyk means Joy of geeK