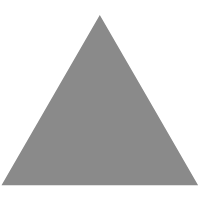
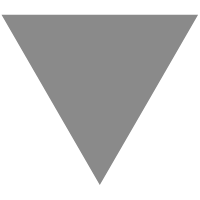
Giant leaps from small things - UK quantum firm sees reason
source link: https://diginomica.com/giant-leaps-small-things-uk-quantum-firm-sees-reason
Go to the source link to view the article. You can view the picture content, updated content and better typesetting reading experience. If the link is broken, please click the button below to view the snapshot at that time.
Giant leaps from small things - UK quantum firm sees reason
Summary:
Quantum computers are beginning to do what classical computers find hardest - infer meaning from absent data.

Quantum Computing aims to apply the science of very small things - the behaviour of subatomic particles - to solving very big problems. These are the questions that classical computers are either unable to answer or would take years to compute in the yes/no world of binary processors.
One of the biggest problem areas is reasoning. Namely, can computers reason in a way that is similar to human thought processes, which often veer into the complex, intuitive, and experiential?
For example, people are generally able to infer that something has happened from partial information. You or I could look out of a window and see a sopping wet lawn, a clear sky, dry pavements, and a sprinkler, and instantly conclude that someone has watered the grass. Computers find that tough.
But what if the sky is cloudy? To a human, dry pavements would still suggest a sprinkler as a more likely explanation than a sudden downpour. The point is that we would reach that conclusion swiftly, while considering a huge range of other factors in an instant: the smell of the air, the absence of raindrops on windows, people's attire and appearance, and so on.
Humans' mix of intuition, experience, and DNA makes this type of process both swift and easy. But it's much harder to model in a computer, which might find itself cycling through a loop with no obvious conclusion - like a Bayesian network with no arrow pointing to the right answer. Insufficient data. This is why the so-called ‘sprinkler problem' has become an archetype of the challenge facing quantum developers.
In layman's terms, we could describe what the human brain does in this instance as extracting probability from uncertainty, but the more accurate term is variational inference (VI) in complex systems. In other words, answering questions about things that we cannot see (unobserved variables) by looking at the things we can (observed variables). In this way, we can draw accurate conclusions from them.
Commercial applications
An announcement at the end of March from software tools company Cambridge Quantum Computing (CQC) suggested that quantum computers can now perform this type of computation much better and faster than classical machines.
In a paper published on Cornell University's arXiv platform, a team of researchers led by CQC's Marcello Benedetti say they have demonstrated "efficient variational inference with distributions beyond those that are efficiently representable on a classical computer". They have proved this both theoretically and experimentally via tests on an IBM quantum device. The paper explains:
We adopt a distinct approach that focuses on improving inference in classical probabilistic models using quantum resources. We use Born machines, which are quantum machine learning models that exhibit high expressivity. We show how to employ gradient-based methods and amortization in the training phase. These choices are inspired by recent advances in classical VI.
It adds:
Finding a quantum advantage in Machine Learning in any capacity is an exciting research goal.
That much is undeniable. Far from being the abstract academic achievement it might appear to be, being able to do this at speed in a computer could have far-reaching commercial applications. For example, in healthcare - including in drug discovery and faster medical diagnoses (CQC cites cancer as an example) - and in biology, genetics, forensics, finance, materials science, carbon sequestration, and fault diagnostics, among many others.
In the future, the use of quantum techniques in natural language processing is another fast-emerging area: conversational machines and computers that can understand vague or imprecise utterances, again inferring meaning from incomplete data.
Demonstrating these and other commercial advantages for quantum technologies was one of the founding purposes of CQC when it emerged in 2014 from Cambridge University's Accelerate Cambridge entrepreneurship programme, the aim of which was to commercialise deep science.
However, CEO Ilyas Khan accepts that any claim that quantum computers can ‘reason' is too bold to make, particularly as it is a trigger word for people who worry about sci-fi dystopias. It's all about inference, he says, rather than any implied notion of sentience.
It's not a guess
That said, there are few, if any, aspects of human life that will not be impacted "when fault-tolerant quantum computers - machines that no longer suffer from error and instability - become commonplace", he believes. That brings real ethical concerns, once clearer signals emerge from the noise of quantum processors, just as real-world applications emerge from hype.
That aside, Khan believes that quantum computers could serve humanity well in two ways: either by doing things that classical computers are unable to do, thanks to the limitations imposed by the laws of physics; or by carrying out calculations much, much faster. The latter could be via standalone devices or by simulating quantum processes within classical computers - or using quantum components to accelerate classical processing. He tells me:
There's a legitimate reason for trying to make quantum computers do things better and faster, but there's another thing: those instances where you simply can't do it on a classical computer. And one of the things in that category is the extraction from Mother Nature of raw entropy.
You've heard of the Bell Test [which tests Einstein's adherence to the concept of ‘local realism', when links appear to exist between quantum particles, even if they are at opposite ends of the universe]. If I said, ‘What is the perfect random sequence?' You could spend billions or trillions of dollars and come up with some fancy algorithm, with supercomputers, and pull it all together, but it would not match Mother Nature in giving you randomness.
A fundamental law of quantum mechanics allows you to extract from Mother Nature strings of randomness that will never be repeated, with no pattern. What we've done at Cambridge Quantum is find a way of extracting from the data, in a commercial and viable manner, randomness, if you like, rather than entropy, which is certifiably quantum and therefore maximally random. We can then package that - though these things are very hard to do, they're not trivial.
So, this is one area where we can do things which classical computers cannot do. This is not a computable exercise, it's not like data science or machine learning. It is using a quantum computer to do something which otherwise could not be done. The use cases are, as you can imagine, cryptography and cyber-security.
But back to the archetypal ‘sprinkler' problem and the challenge of computer reasoning. What about Cambridge's experiments in inference specifically, which appear to dramatically speed up the process of inferring conclusions from imperfect information, improving on the classical world? He says:
As far as we're aware - there might be something hidden that somebody hasn't published yet - this is the first novel implementation of a quantum computing algorithm for a useful real-world application.
That's great news, both for Quantum Computing as a discipline and for UK science, technology, and entrepreneurship. But isn't there a risk that such machines might infer things incorrectly? Toss a coin - or rather a qubit - and come out with completely the wrong answer? Khan explains that this is the wrong way of looking at the research:
This isn't about ‘guessing' things. Very often - in fact, we do it all the time - we know that humans infer something correctly. We know that, so this is in that part of computational complexity.
I'll give you another example. I've got kids, I'm married, I've got family and I've got colleagues, so, there is a group of perhaps 50 voices that are dear to me. When I hear one of those voices - sometimes very crackly - on the phone, even though I can't see them I know who it is. That's an inference. Yes, there may be an infinitesimally small chance that I'm wrong, but it's unlikely.
My take
Let's hope that Khan's sentiment applies to Cambridge Quantum Computing itself. The company is certainly doing a lot of things right. Its enabling technology, TKET, has access to "literally the biggest number of hardware companies in the world" in the quantum field, says Khan, via millions of dollars of targeted expenditure. And it has thousands of users worldwide.
He says: "We don't just theorise, we implement, and we experiment."
That's the ticket.
Recommend
About Joyk
Aggregate valuable and interesting links.
Joyk means Joy of geeK