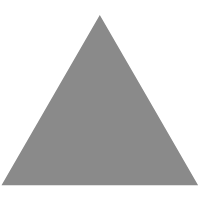
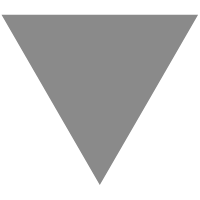
AI datasets are filled with errors. It's warping what we know about AI | MIT Tec...
source link: https://www.technologyreview.com/2021/04/01/1021619/ai-data-errors-warp-machine-learning-progress
Go to the source link to view the article. You can view the picture content, updated content and better typesetting reading experience. If the link is broken, please click the button below to view the snapshot at that time.
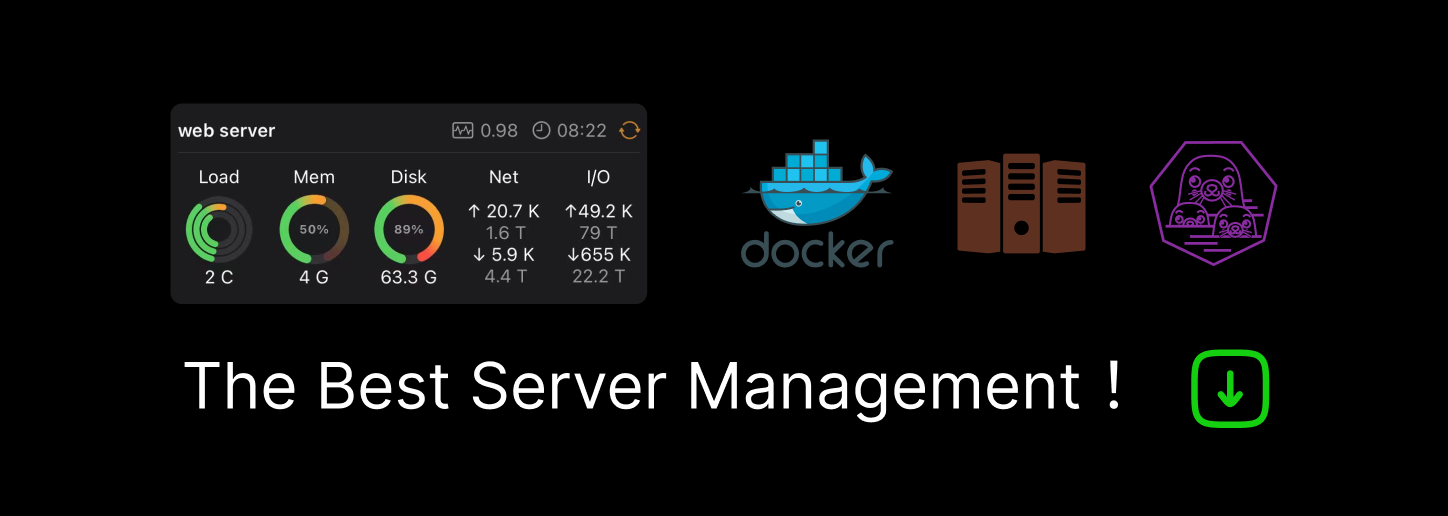
Error-riddled data sets are warping our sense of how good AI really is
Our understanding of progress in machine learning has been colored by flawed testing data.
The10 most cited AI data sets are riddled with label errors, according to a new study out of MIT, and it’s distorting our understanding of the field’s progress.
Data backbone: Data sets are the backbone of AI research, but some are more critical than others. There are a core set of them that researchers use to evaluate machine-learning models as a way to track how AI capabilities are advancing over time. One of the best-known is the canonical image-recognition data set ImageNet, which kicked off the modern AI revolution. There’s also MNIST, which compiles images of handwritten numbers between 0 and 9. Other data sets test models trained to recognize audio, text, and hand drawings.
Sign up for The Download
- Your daily dose of what's up in emerging technologyStay updated on MIT Technology Review initiatives and events?
Yes, but: In recent years, studies have found that these data sets can contain serious flaws. ImageNet, for example, contains racist and sexist labels as well as photos of people’s faces obtained without consent. The latest study now looks at another problem: many of the labels are just flat-out wrong. A mushroom is labeled a spoon, a frog is labeled a cat, and a high note from Ariana Grande is labeled a whistle. The ImageNet test set has an estimated label error rate of 5.8%. Meanwhile, the test set for QuickDraw, a compilation of hand drawings, has an estimated error rate of 10.1%.
How was it measured? Each of the 10 data sets used for evaluating models has a corresponding data set used for training them. The researchers, MIT graduate students Curtis G. Northcutt and Anish Athalye and alum Jonas Mueller, used the training data sets to develop a machine-learning model and then used it to predict the labels in the testing data. If the model disagreed with the original label, the data point was flagged up for manual review. Five human reviewers on Amazon Mechanical Turk were asked to vote on which label—the model’s or the original—they thought was correct. If the majority of the human reviewers agreed with the model, the original label was tallied as an error and then corrected.
Does this matter? Yes. The researchers looked at 34 models whose performance had previously been measured against the ImageNet test set. Then they remeasured each model against the roughly 1,500 examples where the data labels were found to be wrong. They found that the models that didn’t perform so well on the original incorrect labels were some of the best performers after the labels were corrected. In particular, the simpler models seemed to fare better on the corrected data than the more complicated models that are used by tech giants like Google for image recognition and assumed to be the best in the field. In other words, we may have an inflated sense of how great these complicated models are because of flawed testing data.
Now what? Northcutt encourages the AI field to create cleaner data sets for evaluating models and tracking the field’s progress. He also recommends that researchers improve their data hygiene when working with their own data. Otherwise, he says, “if you have a noisy data set and a bunch of models you’re trying out, and you’re going to deploy them in the real world,” you could end up selecting the wrong model. To this end, he open-sourced the code he used in his study for correcting label errors, which he says is already in use at a few major tech companies.
Recommend
-
64
PyChubby — Automated Face Warping
-
49
PyChubby Tool for automated face warping
-
35
Let us consider the following task: we have a bunch of evenly distributed time series of different lengths. The goal is to cluster time series by defining general patterns that are presented in the data. Here I...
-
6
Visualizing Warping Strategies for Sampling Environment Map Lights Jun 5, 2019 (I know, there’s supposedly another post on sampling triangular light sources coming. Soon, maybe!...
-
12
Rust and Dynamic Time Warping Read Time: 7 minutes I’m back to a familar topic, dynamic time warping. This time it’s my excuse to play with Rust. As a bit o...
-
11
Scientists Make Breakthrough in Warping Time at Smallest Scale EverScientists were able to measure time dilation at a distance of just a millimeter, about the width of a pencil tip.February 16,...
-
5
Gooey Warping SVG NumbersFeaturing Fabio OttavianiWritten by Alex TrostThis morphing number CodePen from Fabio Ottaviani is a clever use of two great techniques.Both techniques make use of SVG’s powerf...
-
8
Reducing Warping In Metal 3D Prints We are used to dealing with warping when printing with thermoplastics like ABS, but metal printers suffer from this problem, too. The University of Michigan has a new technology,...
-
10
Automatically Find Errors in ML Datasetscleanlab 2.0: Automatically Find Errors in ML Datasets04/21/2022
-
11
How to Prevent Warping in 3D Prints: The Ultimate Guide By Sammy Ekaran Published 6 hours ago If your 3D print...
About Joyk
Aggregate valuable and interesting links.
Joyk means Joy of geeK