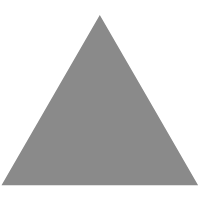
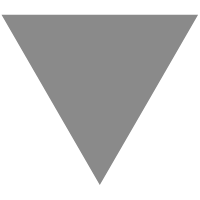
Response to Goldberg’s Blog on Deep Learning for NLG – Ehud Reiter's Blog
source link: https://ehudreiter.com/2017/06/19/response-to-goldberg/
Go to the source link to view the article. You can view the picture content, updated content and better typesetting reading experience. If the link is broken, please click the button below to view the snapshot at that time.
Response to Goldberg’s Blog on Deep Learning for NLG
I wrote a comment in response to Yoav Goldberg’s An Adversarial Review of “Adversarial Generation of Natural Language” on Medium, which essentially critiques some research using deep learning in NLG, focusing on papers published in less prestigious venues. Looks like quite a few people read this comment, so I am reposting my comment on this blog.
**********************************************************************************
I saw that Mike White mentioned my name, so I thought I would comment directly. A lot of the discussion is about papers published in second-tier venues, but from my perspective there are also major problems with DL NLG papers published in top venues. Perhaps less drastic, but its a question of degree.
This was brought home to me last year when I attended NAACL 2016 (in order to give an invited talk on NLG evaluation), which was the first time I had been to an ACL event in several years. I went to listen to a NAACL paper about using DL for NLG, and was absolutely horrified.
(1) The evaluation was weak, because the authors just used BLEU, which is a questionable way to evaluate NLG systems (https://ehudreiter.com/2017/05/03/metrics-nlg-evaluation/)
(2) One of the main training corpora used was the output of a rule-based NLG system (https://ehudreiter.com/2017/05/09/weathergov/). So were the authors trying to show that they could use DL to reverse engineer a rule-based system and steal the IP of someone who spent a lot of time carefully hand-crafting NLG rules?
(3) The presenting author was completely unaware of previous work in the NLG community on the problems he was solving (this was apparent in the Q&A session as well as in the paper). He claimed his system was better than state-of-the-art, but to me his output texts looked considerably worse than stuff we were producing 15 years ago.
I am willing to be convinced that DL is a good approach for NLG, but I need to see experiments and papers with solid evaluation, sensible and appropriate corpora, and good awareness of NLG state-of-the-art. Papers like the above NAACL one dont leave me with a good impression of DL for NLG.
I’d also like someone to explain to me how we can evaluate the worst-case (as well as the average case) performance of DL systems, because this is really important (https://ehudreiter.com/2016/12/12/nlg-and-ml/).
Finally, to echo some of the other opinions which people have expressed, there is a caricature of a DL (or indeed ML) NLP researcher as someone who just wants some corpora and a way to keep score, and has no interest whether the “score” means anything and also no interest in the provenance or suitability of the corpora. I realise this is a caricature, but I think it has some truth, and I dont think this is the right attitude for making progress in NLP.
Like this:
Recommend
About Joyk
Aggregate valuable and interesting links.
Joyk means Joy of geeK