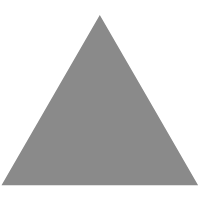
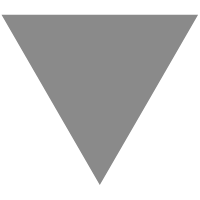
Not Getting the Most From Your Model Ops? Why Businesses Struggle With Data Scie...
source link: https://www.tibco.com/blog/2021/03/08/not-getting-the-most-from-your-model-ops-why-businesses-struggle-with-data-science-and-machine-learning/
Go to the source link to view the article. You can view the picture content, updated content and better typesetting reading experience. If the link is broken, please click the button below to view the snapshot at that time.
Not Getting the Most From Your Model Ops? Why Businesses Struggle With Data Science and Machine Learning
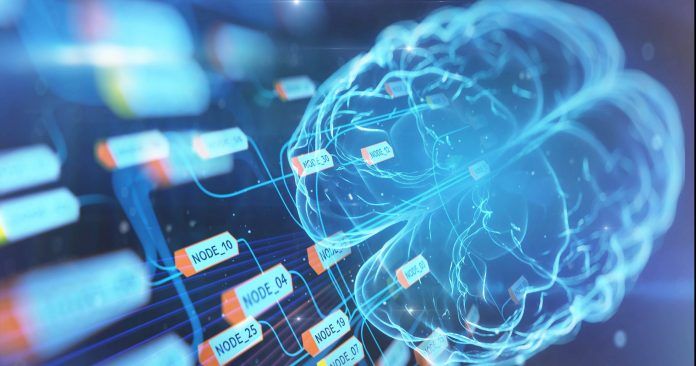
Companies have begun to recognize the value of integrating data science (DS) and machine learning (ML) across their organization to reap the benefits of the advanced analytics they can provide. As such, DS/ML has seen a surge in popularity and usage as businesses have invested heavily in this technology.
However, there’s a distinct difference between investing in DS/ML and managing to successfully gain tangible business value from that investment, and that’s where organizations are running into problems.
The Results Are in: Businesses Struggle With DS/ML Deployment Across the Board
We recently performed a global survey across 18 countries and 22 industries, including over a hundred business leaders and executives, more than half of which were in the C-Suite.
Of those respondents, just 14 percent reported that they are currently operationalizing DS/ML. Within that 14 percent, 24 percent can only use it in one functional area, far below the potential innovative capability of the technology.
Why are so few organizations able to follow through with model ops adoption? What are the barriers keeping businesses from operationalizing data science and machine learning?
The Devil’s in the Data
According to the survey results, while a lack of talented data scientists to build the models was listed in the top ten obstacles to DS/ML adoption, it was only cited by about 16 percent of respondents. On the other hand, seven of these ten, including the top four, were all data-related. Issues with data security, data privacy, data prep, and data access, in particular, were all cited by between 27 to 38 percent of respondents.
While there are many other issues to contend with, including lack of management and financial support and a clear integration strategy, security compliance and data privacy concerns are clearly a significant barrier when it comes to operationalizing DS/ML.
Why Overcoming These Problems are Critical for Innovation
Data scientists can develop as many models as they want for a business, but if they don’t get deployed, then they aren’t providing any value. For the modern digital business to have any hope of keeping up with the competition, model ops is a vital tool that can allow them to effectively operationalize DS/ML models, putting them into production and applying them to streaming, real-time data, edge applications, and more.
For a more in-depth breakdown of our survey results, you can check out our full ebook now. And if you’re ready to move past insights and into action, you can download our four-step guide to finding out what it takes to operationalize data science within your organization and get a leg-up on the competition.
Recommend
About Joyk
Aggregate valuable and interesting links.
Joyk means Joy of geeK