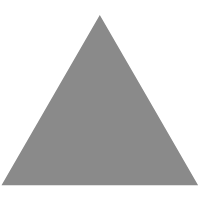
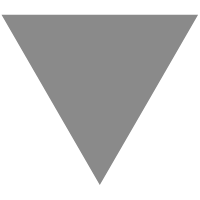
Inverse-gamma distribution
source link: https://en.wikipedia.org/wiki/Inverse-gamma_distribution
Go to the source link to view the article. You can view the picture content, updated content and better typesetting reading experience. If the link is broken, please click the button below to view the snapshot at that time.
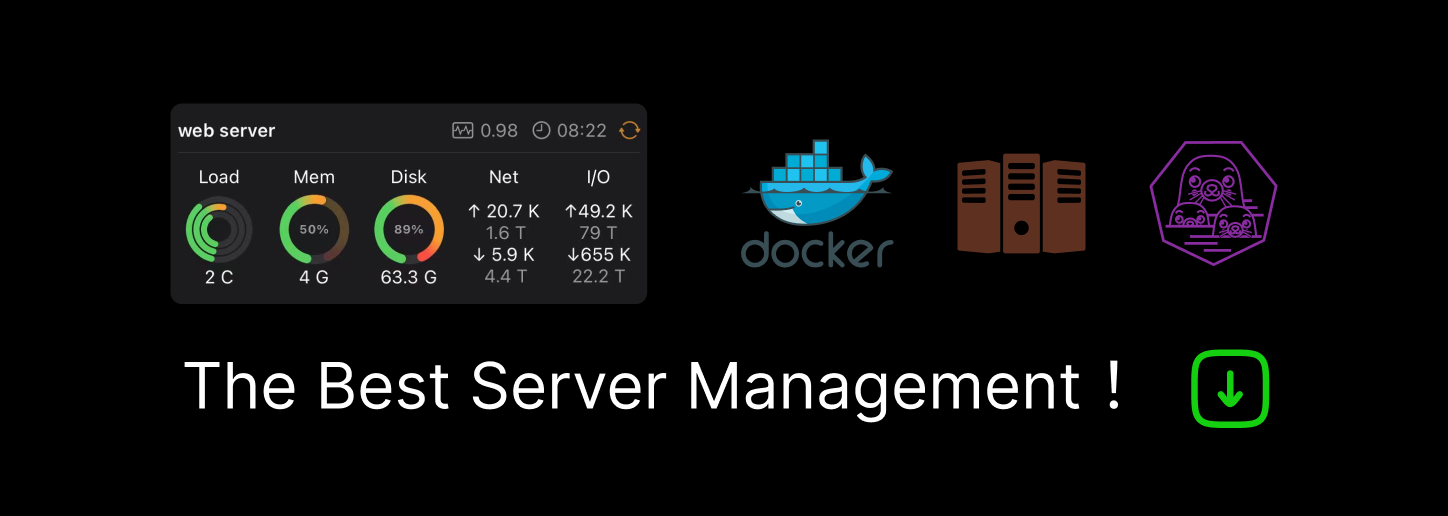
Inverse-gamma distribution
Jump to navigation Jump to search
Inverse-gamma
β>0{\displaystyle \beta >0}
α+ln(βΓ(α))−(1+α)ψ(α){\displaystyle \alpha \!+\!\ln(\beta \Gamma (\alpha ))\!-\!(1\!+\!\alpha )\psi (\alpha )}
In probability theory and statistics, the inverse gamma distribution is a two-parameter family of continuous probability distributions on the positive real line, which is the distribution of the reciprocal of a variable distributed according to the gamma distribution. Perhaps the chief use of the inverse gamma distribution is in Bayesian statistics, where the distribution arises as the marginal posterior distribution for the unknown variance of a normal distribution, if an uninformative prior is used, and as an analytically tractable conjugate prior, if an informative prior is required.
However, it is common among Bayesians to consider an alternative parametrization of the normal distribution in terms of the precision, defined as the reciprocal of the variance, which allows the gamma distribution to be used directly as a conjugate prior. Other Bayesians prefer to parametrize the inverse gamma distribution differently, as a scaled inverse chi-squared distribution.
Characterization[edit]
Probability density function[edit]
The inverse gamma distribution's probability density function is defined over the support x>0{\displaystyle x>0}
with shape parameter α{\displaystyle \alpha } and scale parameter β{\displaystyle \beta }
.[1] Here Γ(⋅){\displaystyle \Gamma (\cdot )}
denotes the gamma function.
Unlike the Gamma distribution, which contains a somewhat similar exponential term, β{\displaystyle \beta } is a scale parameter as the distribution function satisfies:
Cumulative distribution function[edit]
The cumulative distribution function is the regularized gamma function
F(x;α,β)=Γ(α,βx)Γ(α)=Q(α,βx){\displaystyle F(x;\alpha ,\beta )={\frac {\Gamma \left(\alpha ,{\frac {\beta }{x}}\right)}{\Gamma (\alpha )}}=Q\left(\alpha ,{\frac {\beta }{x}}\right)\!}where the numerator is the upper incomplete gamma function and the denominator is the gamma function. Many math packages allow direct computation of Q{\displaystyle Q}, the regularized gamma function.
Moments[edit]
The n-th moment of the inverse gamma distribution is given by[2]
E[Xn]=βn(α−1)⋯(α−n).{\displaystyle \mathrm {E} [X^{n}]={\frac {\beta ^{n}}{(\alpha -1)\cdots (\alpha -n)}}.}Characteristic function[edit]
Kα(⋅){\displaystyle K_{\alpha }(\cdot )} in the expression of the characteristic function is the modified Bessel function of the 2nd kind.
Properties[edit]
For α>0{\displaystyle \alpha >0} and β>0{\displaystyle \beta >0}
,
The information entropy is
H(X)=E[−ln(p(X))]=E[−αln(β)+ln(Γ(α))+(α+1)ln(X)+βX]=−αln(β)+ln(Γ(α))+(α+1)ln(β)−(α+1)ψ(α)+α=α+ln(βΓ(α))−(α+1)ψ(α).{\displaystyle {\begin{aligned}\operatorname {H} (X)&=\operatorname {E} [-\ln(p(X))]\\&=\operatorname {E} \left[-\alpha \ln(\beta )+\ln(\Gamma (\alpha ))+(\alpha +1)\ln(X)+{\frac {\beta }{X}}\right]\\&=-\alpha \ln(\beta )+\ln(\Gamma (\alpha ))+(\alpha +1)\ln(\beta )-(\alpha +1)\psi (\alpha )+\alpha \\&=\alpha +\ln(\beta \Gamma (\alpha ))-(\alpha +1)\psi (\alpha ).\end{aligned}}}where ψ(α){\displaystyle \psi (\alpha )} is the digamma function.
The Kullback-Leibler divergence of Inverse-Gamma(αp, βp) from Inverse-Gamma(αq, βq) is the same as the KL-divergence of Gamma(αp, βp) from Gamma(αq, βq):
DKL(αp,βp;αq,βq)=E[logρ(X)π(X)]=E[logρ(1/Y)π(1/Y)]=E[logρG(Y)πG(Y)],{\displaystyle D_{\mathrm {KL} }(\alpha _{p},\beta _{p};\alpha _{q},\beta _{q})=\mathbb {E} \left[\log {\frac {\rho (X)}{\pi (X)}}\right]=\mathbb {E} \left[\log {\frac {\rho (1/Y)}{\pi (1/Y)}}\right]=\mathbb {E} \left[\log {\frac {\rho _{G}(Y)}{\pi _{G}(Y)}}\right],}
where ρ,π{\displaystyle \rho ,\pi } are the pdfs of the Inverse-Gamma distributions and ρG,πG{\displaystyle \rho _{G},\pi _{G}}
are the pdfs of the Gamma distributions, Y{\displaystyle Y}
is Gamma(αp, βp) distributed.
Related distributions[edit]
- If X∼Inv-Gamma(α,β){\displaystyle X\sim {\mbox{Inv-Gamma}}(\alpha ,\beta )}
then kX∼Inv-Gamma(α,kβ){\displaystyle kX\sim {\mbox{Inv-Gamma}}(\alpha ,k\beta )\,}
- If X∼Inv-Gamma(α,12){\displaystyle X\sim {\mbox{Inv-Gamma}}(\alpha ,{\tfrac {1}{2}})}
then X∼Inv-χ2(2α){\displaystyle X\sim {\mbox{Inv-}}\chi ^{2}(2\alpha )\,}
(inverse-chi-squared distribution)
- If X∼Inv-Gamma(α2,12){\displaystyle X\sim {\mbox{Inv-Gamma}}({\tfrac {\alpha }{2}},{\tfrac {1}{2}})}
then X∼Scaled Inv-χ2(α,1α){\displaystyle X\sim {\mbox{Scaled Inv-}}\chi ^{2}(\alpha ,{\tfrac {1}{\alpha }})\,}
(scaled-inverse-chi-squared distribution)
- If X∼Inv-Gamma(12,c2){\displaystyle X\sim {\textrm {Inv-Gamma}}({\tfrac {1}{2}},{\tfrac {c}{2}})}
then X∼Levy(0,c){\displaystyle X\sim {\textrm {Levy}}(0,c)\,}
(Lévy distribution)
- If X∼Inv-Gamma(1,c){\displaystyle X\sim {\textrm {Inv-Gamma}}(1,c)}
then 1X∼Exp(c){\displaystyle {\tfrac {1}{X}}\sim {\textrm {Exp}}(c)\,}
(Exponential distribution)
- If X∼Gamma(α,β){\displaystyle X\sim {\mbox{Gamma}}(\alpha ,\beta )\,}
(Gamma distribution with rate parameter β{\displaystyle \beta }
) then 1X∼Inv-Gamma(α,β){\displaystyle {\tfrac {1}{X}}\sim {\mbox{Inv-Gamma}}(\alpha ,\beta )\,}
(see derivation in the next paragraph for details)
- Note that If X ~ Gamma(k, θ) (Gamma distribution with scale parameter θ ) then 1/X ~ Inv-Gamma(k, θ−1)
- Inverse gamma distribution is a special case of type 5 Pearson distribution
- A multivariate generalization of the inverse-gamma distribution is the inverse-Wishart distribution.
- For the distribution of a sum of independent inverted Gamma variables see Witkovsky (2001)
Derivation from Gamma distribution[edit]
Let X∼Gamma(α,β){\displaystyle X\sim {\mbox{Gamma}}(\alpha ,\beta )}, and recall that the pdf of the gamma distribution is
Note that β{\displaystyle \beta } is the rate parameter from the perspective of the gamma distribution.
Define the transformation Y=g(X)=1X{\displaystyle Y=g(X)={\tfrac {1}{X}}}. Then, the pdf of Y{\displaystyle Y}
is
Note that β{\displaystyle \beta } is the scale parameter from the perspective of the inverse gamma distribution.
Occurrence[edit]
See also[edit]
References[edit]
- ^ "InverseGammaDistribution—Wolfram Language Documentation". reference.wolfram.com. Retrieved 9 April 2018.
- ^ John D. Cook (Oct 3, 2008). "InverseGammaDistribution" (PDF). Retrieved 3 Dec 2018.
- Hoff, P. (2009). "A first course in bayesian statistical methods". Springer.
- Witkovsky, V. (2001). "Computing the Distribution of a Linear Combination of Inverted Gamma Variables". Kybernetika. 37 (1): 79–90. MR 1825758. Zbl 1263.62022.
with finite supportDiscrete univariate
with infinite supportContinuous univariate
supported on a bounded intervalContinuous univariate
supported on a semi-infinite intervalContinuous univariate
supported on the whole real lineContinuous univariate
with support whose type variesMixed continuous-discrete univariateMultivariate (joint)DirectionalDegenerate and singularFamilies
Recommend
About Joyk
Aggregate valuable and interesting links.
Joyk means Joy of geeK