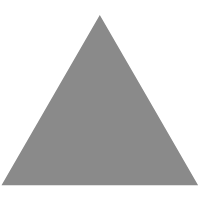
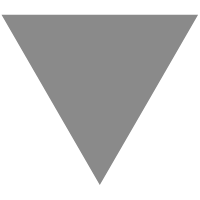
The Dark Past of Algorithms That Associate Appearance and Criminality
source link: https://www.americanscientist.org/article/the-dark-past-of-algorithms-that-associate-appearance-and-criminality
Go to the source link to view the article. You can view the picture content, updated content and better typesetting reading experience. If the link is broken, please click the button below to view the snapshot at that time.
The Dark Past of Algorithms That Associate Appearance and Criminality
By Catherine Stinson
Machine learning that links personality and physical traits warrants critical review.
Computer Technology
"Phrenology" has an old-fashioned ring to it. It sounds like it belongs in a history book, filed somewhere between bloodletting and velocipedes. We’d like to think that judging people’s worth based on the size and shape of their skulls is a practice that’s well behind us. However, phrenology is once again rearing its lumpy head, this time under the guise of technology.
In recent years, machine-learning algorithms have seen an explosion of uses, legitimate and shady. Several recent applications promise governments and private companies the power to glean all sorts of information from people’s appearances. Researchers from Stanford University built a “gaydar” algorithm that they say can tell straight and gay faces apart more accurately than people can. The researchers indicated that their motivation was to expose a potential privacy threat, but they also declared their results as consistent with the “prenatal hormone theory” that hypothesizes that fetal exposure to androgens helps determine sexual orientation; the researchers cite the much-contested claim that these hormone exposures would also result in gender-atypical faces.
- An increasing number of artificial intelligence (AI) technologies claim to be able to find associations between appearance and character traits better than humans can.
- However, the generalizations that these algorithms make can often be explained by factors that do not relate to genetics, such as social presentation.
- Rather than producing objective insights, researchers are cautioning that AI programs are often reinforcing human biases and could harm already marginalized populations.
Several startups claim to be able to use artificial intelligence (AI) to help employers detect the personality traits of job candidates based on their facial expressions. In China, the government has pioneered the use of surveillance cameras that identify and track ethnic minorities. Meanwhile, reports have emerged of schools installing camera systems that automatically sanction children for not paying attention, based on facial movements and microexpressions such as eyebrow twitches. University students taking online exams monitored by proctoring algorithms not only have to answer the questions, but also maintain the appearance of a student who is not cheating. These algorithms reportedly make false accusations against students with disabilities who move their faces and hands in atypical ways, and Black students have indicated that they have been required to shine bright lights in their faces so as to have their features detected at all.
Courtesy of Rachael E. Jack; from Jack et al., 2016.
Perhaps the most notorious recent misuse of facial recognition is the case of AI researchers Xiaolin Wu and Xi Zhang of Shanghai Jiao Tong University, who claimed to have trained an algorithm to identify criminals based on the shape of their faces, with an accuracy of 89.5 percent. The 2016 work has appeared only as a preprint, not in a peer-reviewed journal. The researchers didn’t go quite so far as to endorse the ideas about physiognomy and character that circulated in the 19th century, notably from the work of the Italian criminologist Cesare Lombroso: that criminals are under-evolved, subhuman beasts, recognizable from their sloping foreheads and hawklike noses. However, the study’s seemingly high-tech attempt to pick out facial features associated with criminality borrows directly from the “photographic composite method” developed by Victorian jack-of-all-trades Francis Galton—which involved overlaying the faces of multiple people to find the features indicative of qualities such as health, disease, beauty, or criminality.
Technology commentators have panned these facial-recognition technologies as “literal phrenology”; they’ve also linked some applications to eugenics, phrenology’s parent pseudoscience that aims to “improve” the human race by encouraging people deemed the fittest to reproduce, and discouraging childbearing in those deemed unfit. Galton himself coined the term eugenics, describing it in 1883 as “all influences that tend in however remote a degree to give to the more suitable races or strains of blood a better chance of prevailing speedily over the less suitable than they otherwise would have had.” China’s surveillance of ethnic minorities has the explicit goal of denying opportunities to those deemed unfit. Technologies that attempt to detect the faces of criminals or exam cheaters may have more noble goals, but tend to lead to the same predictable result: a lot of false positives for already marginalized people, leading to the denial of rights and opportunities.
Yet when algorithms are dismissed with labels such as ”phrenology” or “pseudoscience,” what exactly is the problem being pointed out? Is phrenology scientifically flawed? Or is it morally wrong to use it, even if it could work?
Flawed Data Sets
There is a long and tangled history to the way the word phrenology has been used as a withering insult. Moral and scientific criticisms of the endeavor have always been intertwined, although their entanglement has changed over time. In the 19th century, phrenology’s detractors objected to the fact that the practice attempted to pinpoint the location of different mental functions in different parts of the brain—a move that was seen as heretical, because it called into question Christian ideas about the unity of the soul. Interestingly, though, trying to discover a person’s character and intellect based on the size and shape of their head wasn’t perceived as a serious moral issue. Today, by contrast, the idea of localizing mental functions is fairly uncontroversial. Scientists might no longer think that destructiveness is seated above the right ear, for instance, but the notion that cognitive functions can be localized in particular brain circuits is a standard assumption in mainstream neuroscience.
Courtesy of the Wellcome Collection
Phrenology had its share of empirical criticism in the 19th century, too. Debates raged about which functions resided where, and whether skull measurements were a reliable way of determining what’s going on in the brain (they’re not). The most influential empirical criticism of old phrenology, though, came from French physician Jean Pierre Flourens’s studies based on damaging the brains of rabbits and pigeons—from which he concluded that mental functions are distributed, rather than localized. (This result was later re-interpreted.) The fact that phrenology was rejected for reasons that most contemporary observers would no longer accept makes it only more difficult to figure out what we’re targeting when we use phrenology as a slur today.
Some commentators argue that facial recognition should be regulated as tightly as plutonium, because it has so few non harmful uses.
Both “old” and “new” phrenology have been critiqued for their sloppy methods. In the recent AI study of criminality, the data were taken from two very different sources: ID photos provided by police forces for convicts, versus professional photos scraped from the internet for nonconvicts. Pictures that people willingly post on the internet tend to show them in rather different moods, clothing, and life circumstances than in ID photos. Those facts alone could account for the algorithm’s ability to detect a difference between the groups. Similarly, critics of the gaydar algorithm research point out that there is an obvious explanation for why it is not hard to tell apart the pictures of gay and straight people that the study took from dating sites: They tend to be dressed, made up, and posed differently. Even the angle from which the picture was taken can account for changes in face shapes.
In a new preface to the criminality preprint, the researchers also admitted that taking court convictions as synonymous with criminality was a “serious oversight.” Yet equating convictions with criminality seems to register with the authors mainly as an empirical flaw, in that using pictures of convicted criminals, but not of the ones who were cleared, introduces a statistical bias that skews the results. They said they were “deeply baffled” at the public outrage in reaction to a study that was intended “for pure academic discussions,” which also suggests an unawareness that their work’s flaws go beyond sloppy statistics.
Notably, the researchers don’t comment on the fact that being convicted of a crime itself depends on the impressions that police, judges, and juries form of the suspect—making a person’s “criminal” appearance a confounding variable. They also fail to mention how the intense policing of particular communities, and inequality of access to legal representation, skew the data set. It is utterly unsurprising to find differences in appearance between people who are arrested and convicted and those who are not. As Ice Cube famously argues, the Los Angeles Police Department thinks every Black man is a drug dealer. In their response to criticism, Wu and Zhang don’t back down on the assumption that “being a criminal requires a host of abnormal (outlier) personal traits.” Indeed, their framing suggests that criminality is an innate characteristic, rather than a response to social conditions such as poverty or abuse, or a label applied to exert social control. This assumption mirrors the gaydar study’s quick leap to the conclusion that it must be picking up on something biological. Part of what makes the data set questionable on empirical grounds is that who gets labeled “criminal” is hardly value-neutral.
From Wu and Zhang, 2016.
One of the strongest moral objections to using facial recognition to detect criminality is that it stigmatizes people who are already overpoliced. The authors of the criminality paper say that their tool should not be used in law enforcement, but cite only statistical arguments about why it ought not to be deployed. They note that the false-positive rate is very high (more than 95 percent of people it classifies as criminals have never been convicted of a crime), but take no notice of what that means in human terms. Those false positives would be individuals whose faces resemble people who have been convicted in the past. Given the racial and other biases that exist in the criminal justice system, such algorithms would end up overestimating criminality among marginalized communities.
Try, Try Again?
The most contentious question seems to be whether reinventing phrenology is fair game for the purposes of “pure academic discussion.” One could object on empirical grounds: Eugenicists of the past such as Galton and Lombroso ultimately failed to find facial features that predisposed a person to criminality. That lack of evidence is almost certainly because there are no such connections to be found. Likewise, psychologists who studied the heritability of intelligence, such as Cyril Burt and Philippe Rushton, had to play fast and loose with their data to make it look like they had found genuine connections between skull size, race, and IQ. If there were anything to discover, presumably the many people who have tried over the centuries wouldn’t have come up dry.
Artificial intelligence algorithms seem to have even more power than math to bamboozle.
Complex personal traits such as a tendency to commit crimes are exceedingly unlikely to be genetically linked to appearance in such a way as to be readable from photographs. First, criminality would have to be determined to a significant extent by genes rather than environment. There may be some very weak genetic influences, but any that exist would be washed out by the much larger influence of environment. Second, the genetic markers relevant to criminality would need to be linked in a regular way to genes that determine appearance. This link could happen if genes relevant to criminality were clustered in one section of the genome that happens to be near genes relevant to face shape. For a complex social trait such as criminality, this clustering is extremely unlikely. A much more likely hypothesis is that any association that exists between appearance and criminality works in the opposite direction: A person’s appearance influences how other people treat them, and these social influences are what drives some people to commit crimes (or to be found guilty of them).
The argumentative strategy Wu and Zhang use—of claiming that we ought to be able to look at the evidence with a detached academic eye even when people’s lives hang in the balance—was pioneered by prominent eugenicist and statistician, Karl Pearson. In a recent article on this subject, mathematician Aubrey Clayton argues that statistical significance testing was designed to give a mathematical sheen to eugenic claims that only flawed methods could prop up: “By slathering it in a thick coating of statistics, Pearson gave eugenics an appearance of mathematical fact that would be hard to refute.” Using these (utterly common, but increasingly maligned) statistical methods, Wu and Zhang can offer results that are, technically speaking, statistically significant, but nevertheless highly misleading. AI algorithms seem to have even more power than math to bamboozle.
Do No Harm
The problem with reinventing eugenic methods such as phrenology cloaked in new technological guises is not merely that it has been tried without success many times before. Researchers who persist in looking for cold fusion after the scientific consensus has moved on also face criticism for chasing unicorns—but disapproval of cold fusion falls far short of opprobrium. At worst, cold fusion researchers are seen as wasting their time. The difference is that the potential harms of cold fusion research are much more limited. In contrast, some commentators argue that facial recognition should be regulated as tightly as plutonium, because it has so few nonharmful uses. When the dead-end project you want to resurrect was invented for the purpose of propping up colonial and class structures—and when the only thing it’s capable of measuring is the racism inherent in those structures—it’s hard to justify trying it one more time, just for curiosity’s sake.
Courtesy of Margaret Mitchell
However, calling facial-recognition research “phrenology” without explaining what is at stake isn’t the most effective strategy for communicating the force of the complaint. For scientists to take their moral responsibilities seriously, they need to be aware of the harms that might result from their research. Spelling out more clearly what’s wrong with the work labeled “phrenology” will hopefully have more of an impact than simply throwing the name around as an insult.
Bibliography
- Agüera y Arcas, B., M. Mitchell, and A. Todorov. 2017. Physiognomy’s new clothes. Medium, May 6.
- Agüera y Arcas, B., A. Todorov, and M. Mitchell. 2018. Do algorithms reveal sexual orientation or just expose our stereotypes? Medium, January 11.
- Clayton, A. 2020. How eugenics shaped statistics. Nautilus, October 28.
-
- Graves, J. L. 2002. What a tangled web he weaves: Race, reproductive strategies and Rushton’s life history theory. Anthropological Theory 2:131–154.
- Jack, R. E., et al. 2016. Four not six: Revealing culturally common facial expressions of emotion. Journal of Experimental Psychology: General 145:708–730.
- Kshetri, N. 2020. Remote education is rife with threats to student privacy. The Conversation, November 6.
- Stark, L. 2019. Facial recognition is the plutonium of AI. XRDS 25(3):50–55.
- Van Noorden, R. 2020. The ethical questions that haunt facial-recognition research. Nature 587:354–358.
- Wang, Y., and M. Kosinski. 2018. Deep neural networks are more accurate than humans at detecting sexual orientation from facial images. Journal of Personality and Social Psychology 114:246–257.
- Wu, X., and X. Zhang. 2016. Responses to critiques on machine learning of criminality perceptions. arXiv:1611.04135
- Wu, X., and X. Zhang. 2016. Automated inference on criminality using face images. arXiv:1611.04135v1
This article is adapted and expanded from one that appeared on Aeon, aeon.co.
American Scientist Comment Policy
Stay on topic. Be respectful. We reserve the right to remove comments.
Please read our Comment Policy before commenting.Recommend
About Joyk
Aggregate valuable and interesting links.
Joyk means Joy of geeK