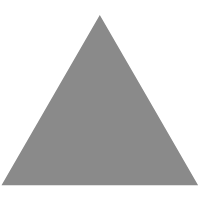
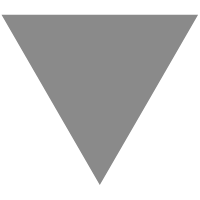
亚马逊提出:用于人群计数的尺度感知注意力网络
source link: https://bbs.cvmart.net/articles/278
Go to the source link to view the article. You can view the picture content, updated content and better typesetting reading experience. If the link is broken, please click the button below to view the snapshot at that time.
亚马逊提出:用于人群计数的尺度感知注意力网络
1年前 ⋅ 1965 ⋅ 1 ⋅ 0
Summary:亚马逊提出:用于人群计数的尺度感知注意力网络
Author:Amusi
Date:2019-02-11
微信公众号:CVer
原文链接:亚马逊提出:用于人群计数的尺度感知注意力网络
知乎:https://zhuanlan.zhihu.com/p/55787893
《Scale-Aware Attention Network for Crowd Counting》
arXiv:https://arxiv.org/abs/1901.06026
作者团队:Amazon
注:2019年01月21日刚出炉的paper
Abstract:In crowd counting datasets, people appear at different scales, depending on their distance to the camera. To address this issue, we propose a novel multi-branch scale-aware attention network that exploits the hierarchical structure of convolutional neural networks and generates, in a single forward pass, multi-scale density predictions from different layers of the architecture. To aggregate these maps into our final prediction, we present a new soft attention mechanism that learns a set of gating masks. Furthermore, we introduce a scale-aware loss function to regularize the training of different branches and guide them to specialize on a particular scale. As this new training requires ground-truth annotations for the size of each head, we also propose a simple, yet effective technique to estimate it automatically. Finally, we present an ablation study on each of these components and compare our approach against the literature on 4 crowd counting datasets: UCF-QNRF, ShanghaiTech A & B and UCF_CC_50. Without bells and whistles, our approach achieves state-of-the-art on all these datasets. We observe a remarkable improvement on the UCF-QNRF (25%) and a significant one on the others (around 10%).
摘要:在人群计数的数据集中,人们以不同的尺度(scales)出现,具体取决于他们与摄像头的距离。为了解决这个问题,我们提出了一种新的多分支尺度感知注意网络,它利用卷积神经网络的层次结构,并在单个前向传播中生成来自架构不同层的多尺度密度预测。为了将这些 maps 聚合到我们的最终预测中,我们提出了一种新的 soft 注意力机制,其可以学习一组 gating masks。此外,我们引入了规模感知损失函数来规范不同分支的训练并指导它们专门研究特定的尺度。由于这种新训练需要对每个头部的大小进行 ground-truth 标注,我们还提出了一种简单而有效的技术来自动估计它。最后,我们对每个部分进行ablation study ,并将我们的方法与4个人群计数数据集的文献进行比较:UCF-QNRF,ShanghaiTech A&B和UCF_CC_50。实验结果表明,我们的方法在这些数据集上取得最先进技术的水平(state-of-the-art,SOTA)。我们观察到UCF-QNRF显著提高(25%),其他显著提高(约10%)。
Our multi-branch architecture
创新点
- Baseline network for crowd counting
- Scale-aware soft attention masks
- Scale-aware loss regularization
- Estimating the size of each head
实验结果
版权声明:自由转载-非商用-非衍生-保持署名(创意共享3.0许可证)
Recommend
About Joyk
Aggregate valuable and interesting links.
Joyk means Joy of geeK