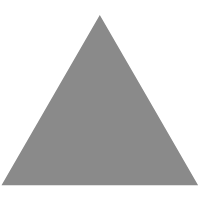
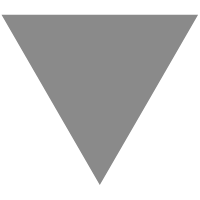
PaddlePaddle2.0 数据加载及处理
source link: https://my.oschina.net/u/4067628/blog/4839732
Go to the source link to view the article. You can view the picture content, updated content and better typesetting reading experience. If the link is broken, please click the button below to view the snapshot at that time.
PaddlePaddle2.0 数据加载及处理
PaddlePaddle2.0 数据加载及处理
大家好这里是小白三岁,三岁白话系列第7话来啦!
下载安装命令 ## CPU版本安装命令 pip install -f https://paddlepaddle.org.cn/pip/oschina/cpu paddlepaddle ## GPU版本安装命令 pip install -f https://paddlepaddle.org.cn/pip/oschina/gpu paddlepaddle-gpu
AIStudio项目地址:
https://aistudio.baidu.com/aistudio/projectdetail/1349615
参考文档:
Paddle官网:https://www.paddlepaddle.org.cn/documentation/docs/zh/2.0-rc/tutorial/quick_start/getting_started/getting_started.html#id3
paddle API查看地址:https://www.paddlepaddle.org.cn/documentation/docs/zh/2.0-rc1/api/index_cn.html
CSDN地址
三岁白话系列CSDN:https://blog.csdn.net/weixin_45623093/category_10616602.html
paddlepaddle社区号:https://blog.csdn.net/PaddlePaddle
# 导入paddle并查看版本
import paddle
print(paddle.__version__)
2.0.0-rc1
分为框架自带数据集和自定义(自己上传)的数据集
数据的处理
paddle对内置的数据集和非内置的提供了两种不用的模式
接下来让我们一起来看看叭!
框架自带数据集
paddle.vision.datasets
是cv(视觉领域)的有关数据集
paddle.text.datasets
是nlp(自然语言领域)的有关数据集
可以使用__all__
魔法方法进行查看
print('视觉相关数据集:', paddle.vision.datasets.__all__)
print('自然语言相关数据集:', paddle.text.datasets.__all__)
视觉相关数据集: ['DatasetFolder', 'ImageFolder', 'MNIST', 'FashionMNIST', 'Flowers', 'Cifar10', 'Cifar100', 'VOC2012']
自然语言相关数据集: ['Conll05st', 'Imdb', 'Imikolov', 'Movielens', 'UCIHousing', 'WMT14', 'WMT16']
ToTensor
ToTensor是位于paddle.vision.transforms
下的API
作用是将 PIL.Image
或 numpy.ndarray
转换成 paddle.Tensor
接下来看一下手写数字识别的数据集的导入吧
在第6话的时候我们就详解了数字识别,这里我们再导入看看
from paddle.vision.transforms import ToTensor # 导入ToTensor API
# 训练数据集 用ToTensor将数据格式转为Tensor
train_dataset = paddle.vision.datasets.MNIST(mode='train', transform=ToTensor()) # 通过mode选择训练集和测试集
# 验证数据集
val_dataset = paddle.vision.datasets.MNIST(mode='test', transform=ToTensor())
Cache file /home/aistudio/.cache/paddle/dataset/mnist/train-images-idx3-ubyte.gz not found, downloading https://dataset.bj.bcebos.com/mnist/train-images-idx3-ubyte.gz
Begin to download
Download finished
Cache file /home/aistudio/.cache/paddle/dataset/mnist/train-labels-idx1-ubyte.gz not found, downloading https://dataset.bj.bcebos.com/mnist/train-labels-idx1-ubyte.gz
Begin to download
........
Download finished
Cache file /home/aistudio/.cache/paddle/dataset/mnist/t10k-images-idx3-ubyte.gz not found, downloading https://dataset.bj.bcebos.com/mnist/t10k-images-idx3-ubyte.gz
Begin to download
Download finished
Cache file /home/aistudio/.cache/paddle/dataset/mnist/t10k-labels-idx1-ubyte.gz not found, downloading https://dataset.bj.bcebos.com/mnist/t10k-labels-idx1-ubyte.gz
Begin to download
..
Download finished
自带数据集的处理方案
paddle.vision.transforms
中就有有关的处理办法
使用__all__
魔法方法查看所有的处理方法
print('数据处理方法:', paddle.vision.transforms.__all__)
数据处理方法: ['BaseTransform', 'Compose', 'Resize', 'RandomResizedCrop', 'CenterCrop', 'RandomHorizontalFlip', 'RandomVerticalFlip', 'Transpose', 'Normalize', 'BrightnessTransform', 'SaturationTransform', 'ContrastTransform', 'HueTransform', 'ColorJitter', 'RandomCrop', 'Pad', 'RandomRotation', 'Grayscale', 'ToTensor', 'to_tensor', 'hflip', 'vflip', 'resize', 'pad', 'rotate', 'to_grayscale', 'crop', 'center_crop', 'adjust_brightness', 'adjust_contrast', 'adjust_hue', 'normalize']
Compose
将用于数据集预处理的接口以列表的方式进行组合。
Resize
将输入数据调整为指定大小。
ColorJitter
随机调整图像的亮度,对比度,饱和度和色调。
from paddle.vision.transforms import Compose, Resize, ColorJitter
# 定义想要使用那些数据增强方式,这里用到了随机调整亮度、对比度和饱和度(ColorJitter),改变图片大小(Resize)
transform = Compose([ColorJitter(), Resize(size=100)])
# 通过transform参数传递定义好的数据增项方法即可完成对自带数据集的应用
train_dataset = paddle.vision.datasets.MNIST(mode='train', transform=transform)
Cache file /home/aistudio/.cache/paddle/dataset/mnist/train-images-idx3-ubyte.gz not found, downloading https://dataset.bj.bcebos.com/mnist/train-images-idx3-ubyte.gz
Begin to download
Download finished
Cache file /home/aistudio/.cache/paddle/dataset/mnist/train-labels-idx1-ubyte.gz not found, downloading https://dataset.bj.bcebos.com/mnist/train-labels-idx1-ubyte.gz
Begin to download
........
Download finished
非自带数据集的定义与加载
定义非自带数据集
paddle.io.Dataset
概述Dataset的方法和行为的抽象类。
映射式(map-style
)数据集需要继承这个基类,映射式数据集为可以通过一个键值索引并获取指定样本的数据集,所有映射式数据集须实现以下方法:
__getitem__
: 根据给定索引获取数据集中指定样本,在 paddle.io.DataLoader
中需要使用此函数通过下标获取样本。
__len__
: 返回数据集样本个数,paddle.io.BatchSampler
中需要样本个数生成下标序列。
from paddle.io import Dataset # 导入Datasrt库
class MyDataset(Dataset):
"""
步骤一:继承paddle.io.Dataset类
"""
def __init__(self, mode='train'):
"""
步骤二:实现构造函数,定义数据读取方式,划分训练和测试数据集
"""
super(MyDataset, self).__init__()
if mode == 'train':
self.data = [
['traindata1', 'label1'],
['traindata2', 'label2'],
['traindata3', 'label3'],
['traindata4', 'label4'],
]
else:
self.data = [
['testdata1', 'label1'],
['testdata2', 'label2'],
['testdata3', 'label3'],
['testdata4', 'label4'],
]
def __getitem__(self, index):
"""
步骤三:实现__getitem__方法,定义指定index时如何获取数据,并返回单条数据(训练数据,对应的标签)
"""
data = self.data[index][0]
label = self.data[index][1]
return data, label
def __len__(self):
"""
步骤四:实现__len__方法,返回数据集总数目
"""
return len(self.data)
# 测试定义的数据集
train_dataset2 = MyDataset(mode='train')
val_dataset2 = MyDataset(mode='test')
print('=============train dataset=============')
for data, label in train_dataset2:
print(data, label)
print('=============evaluation dataset=============')
for data, label in val_dataset2:
print(data, label)
=============train dataset=============
traindata1 label1
traindata2 label2
traindata3 label3
traindata4 label4
=============evaluation dataset=============
testdata1 label1
testdata2 label2
testdata3 label3
testdata4 label4
class paddle.io.DataLoader(dataset, feed_list=None, places=None, return_list=False, batch_sampler=None, batch_size=1, shuffle=False, drop_last=False, collate_fn=None, num_workers=0, use_buffer_reader=True, use_shared_memory=False, timeout=0, worker_init_fn=None)
DataLoader
返回一个迭代器,该迭代器根据 batch_sampler
给定的顺序迭代一次给定的 dataset
DataLoader
支持单进程和多进程的数据加载方式,当 num_workers
大于0时,将使用多进程方式异步加载数据。
# 此处暂时使用手写数字识别的数据进行演示
train_loader = paddle.io.DataLoader(train_dataset, batch_size=64, shuffle=True)
for batch_id, data in enumerate(train_loader()):
x_data = data[0]
y_data = data[1]
print(x_data.numpy().shape)
print(y_data.numpy().shape)
'''
定义了一个数据迭代器train_loader, 用于加载训练数据。
通过batch_size=64我们设置了数据集的批大小为64,
通过shuffle=True,我们在取数据前会打乱数据。
此外,我们还可以通过设置num_workers来开启多进程数据加载,提升加载速度。
'''
非自带数据集处理
方法一:一种是在数据集的构造函数中进行数据增强方法的定义,之后对__getitem__
中返回的数据进行应用
方法二:给自定义的数据集类暴漏一个构造参数,在实例化类的时候将数据增强方法传递进去
这里用方法一进行举例子:
from paddle.io import Dataset # 导入类库 Dataset
class MyDataset(Dataset): # 定义Dataset的子类MyDataset
def __init__(self, mode='train'):
super(MyDataset, self).__init__()
if mode == 'train':
self.data = [
['traindata1', 'label1'],
['traindata2', 'label2'],
['traindata3', 'label3'],
['traindata4', 'label4'],
]
else:
self.data = [
['testdata1', 'label1'],
['testdata2', 'label2'],
['testdata3', 'label3'],
['testdata4', 'label4'],
]
# 定义要使用的数据预处理方法,针对图片的操作
self.transform = Compose([ColorJitter(), Resize(size=100)]) # 和自带数据的处理类似
def __getitem__(self, index):
data = self.data[index][0]
# 在这里对训练数据进行应用
# 这里只是一个示例,测试时需要将数据集更换为图片数据进行测试
data = self.transform(data)
label = self.data[index][1]
return data, label
def __len__(self):
return len(self.data)
这个的内容就先到这里
感觉里面的东西又点多,看看再研究研究,能不能更细节一点,更加白话
那么下次见,给大家一个好的体验!
下载安装命令 ## CPU版本安装命令 pip install -f https://paddlepaddle.org.cn/pip/oschina/cpu paddlepaddle ## GPU版本安装命令 pip install -f https://paddlepaddle.org.cn/pip/oschina/gpu paddlepaddle-gpu
Paddle2.0-外部数据集导入详解
本文同步分享在 博客“三岁学编程”(CSDN)。
如有侵权,请联系 [email protected] 删除。
本文参与“OSC源创计划”,欢迎正在阅读的你也加入,一起分享。
Recommend
About Joyk
Aggregate valuable and interesting links.
Joyk means Joy of geeK