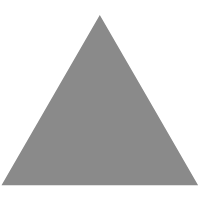
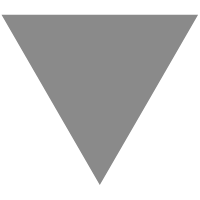
The future urban heat-wave challenge in Africa: Exploratory analysis
source link: https://www.sciencedirect.com/science/article/pii/S0959378020307731?via%3Dihub=
Go to the source link to view the article. You can view the picture content, updated content and better typesetting reading experience. If the link is broken, please click the button below to view the snapshot at that time.
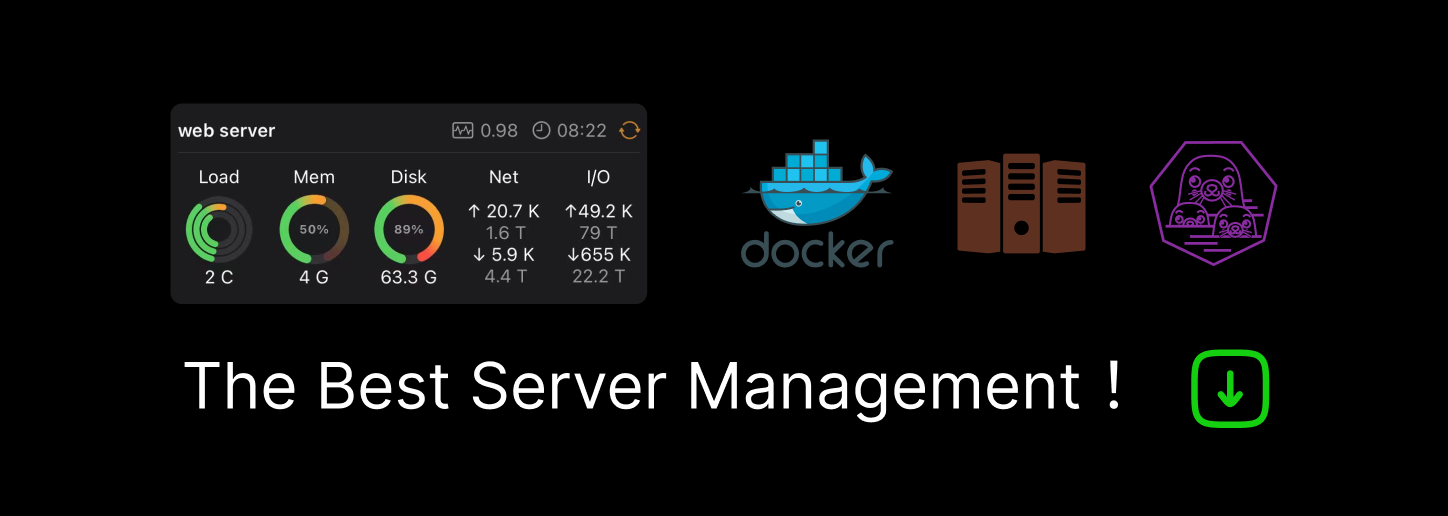
1. Introduction
During the first decades of the twenty-first century, urban scholars have increasingly focused attention on developing-world cities. This is understandable considering the enormous economic and population growth that these parts of the world have recently experienced and the future anticipation of billions of residents and further economic activity. Indeed, according to the UN (2018), after around 2025 all global population growth will be in the world’s cities and over 95% will be in the developing world.
Much interest has been on Asia, as the region has experienced the world’s largest and most intense changes. Towards the middle of this century, however, conditions are expected to shift, as Africa is projected to undergo enormous population growth and urbanization. While Africa has experienced the world’s highest regional population growth rate since the 1970s, total population has remained lower than that of Asia. By 2010, the population of Africa was about a quarter of that of Asia, as the region held approximately 10% of the world’s population. By the end of the twenty-first century, however, the region’s total population is projected to be over 90% of Asia’s (approximately 40% of the world’s total population), exceeding 4.4 billion (UN, 2017). Urbanization across the continent is also projected to increase dramatically (Güneralp et al., 2018, UN, 2018). Despite these population and urbanization forecasts, however, whether economic activity will increase remains uncertain. Urbanization in African nations has not always brought economic growth as experienced by other regions. Currently, more than half of the global extremely poor (those that live on less than US$1.90 a day) live in sub-Saharan Africa. The World Bank projects that if trends continue, by 2030, 9 out of 10 extremely poor will live in the region (World Bank, 2018).
Simultaneously, climate analysts project enhanced climate change, driven by anthropogenic influence. Even if countries meet the Paris Agreement Nationally Determined Contributions, the world will warm by>1.5 °C by 2050 (Obersteiner et al., 2018, Rogelj et al., 2016, Rogelj et al., 2015), bringing climate risks to urban centers around the world (Eakin & Lynd Luers, 2006, IPCC, 2018, Revi et al., 2014). Among a variety of threats, one particularly important climate risk for urban residents is high temperature events. Future projections suggest high-temperature events affecting large portions of Earth (Mora et al., 2017, Seneviratne et al., 2012, Meehl and Tebaldi, 2004, Russo et al., 2014). Generally, climate change-related induced heat events include increases in maximum extreme temperatures, heat waves of greater intensity and duration than currently experienced and increasingly warm summers (Dosio et al., 2018, Seneviratne et al., 2016, Fischer and Schär, 2009, King et al., 2018). Recent studies suggest the number of high temperature events have already increased (Christidis et al., 2015, Rahmstorf and Coumou, 2011, Sun et al., 2014), including in urban areas (Matthews et al., 2017, Mishra et al., 2015). In Africa, heat waves are increasing in intensity and frequency (Ceccherini et al., 2017) and with increasing climate change, this region is also projected to experience significant increases in future extreme heat events (Harrington et al., 2016, Nikulin et al., 2018, Russo et al., 2019, Russo et al., 2016).
While research has projected increases in frequency and intensity of heat waves, there remains much we do not know about the scale of the potential impacts, particularly for African cities. For example, there are limited studies that examine the extent of urban heat wave vulnerability in Africa (Carter, 2018). There also remain very basic unanswered questions. How many urban Africans might be exposed to these heat waves? Where might the largest exposed populations be located within the continent? Of those urban populations exposed, how many might be sensitive to heat events and how many might be living in countries with low incomes, with limited resources to provide relief? The objective of this research is to generate ranges of estimates to help answer these questions. We attempt this through an exploratory scenarios study that provides ranges of urban population and identification of the general location (to the sub-regional level) exposed to 15-day heat events of different magnitudes across three 30-year periods, from 2010 to 2100. Our study suggests a range of futures for urban heat exposure in Africa, although one common element across projections is the large and growing numbers of residents that may be exposed to very warm (42 °C and higher) 15-day heat events.
In the next section, we describe the background to this research. This includes sections on scenario frameworks, urban land cover simulations, heat waves and the urban heat island effect. The section ends with a discussion of the relationship of the project’s results to urban climate vulnerability research. The third section presents the research methods for identification of heat events, urban land cover growth modeling, identification of urban heat islands and how these data are combined in the scenarios. The fourth section provides the results of the analysis including ranges of population exposure to different intensity heat waves of 15-day duration and the share of heat-sensitive and low-income persons in these populations. In the fifth section we discuss the implications of the findings and conclude with a summary and an agenda for future work.
2. Background
2.1. Scenarios
Scholars argue that scenarios are good tools to analyze future trends while addressing uncertainties (Peterson et al., 2003, Schoemaker, 1991, van Vliet and Kok, 2015, Van’ T Klooster & Van Asselt 2011). Several different approaches to scenario development exist (Borjeson et al., 2006, van Notten et al., 2003). While there is no universal scenario typology, literature reviews often include three distinct types: predictive, exploratory, and backcasting (Borjeson et al., 2006). Predictive scenarios forecast how the future will unfold, based on preconceived development patterns. Exploratory scenarios sketch plausible futures, showing the implications of change in external drivers. Though not necessarily for prediction, they focus on what may happen, ultimately exploring uncertainty in outcomes and driving forces (Shearer, 2005, Van Der Heijden, 2000). Typically, exploratory projects include a set of scenarios constructed to span a wide scope of plausible developments over a very long-time span. The third scenario type includes normative, transformation studies. These scenarios start with the end state and work backwards, hence the name “backcasting” (Quist, 2007, Lovins 1977, Robinson, 1982).
We use exploratory scenarios to address the questions of what may happen over the course of the century. There is already a developed framework for exploratory climate change and socio-economic development scenarios (Moss et al., 2008). This framework deploys, at least, two sets of data. The first set is defined by representative concentration pathways (RCPs), which embody climate changes through projecting different levels of greenhouse gas (GHG) concentrations in the atmosphere to 2100. The RCPs represent trajectories for emissions that subsequently affect the radiative forcing of the climate system (van Vuuren et al., 2014). This study uses RCPs 2.6, 4.5, 6.0 and 8.5. The RCP numbers describe energy intensity (watts per m2) above the 1750 level by the end of the current century and are meant to reflect different emission scenarios (Wayne, 2013). The corresponding greenhouse gas concentrations for the emissions scenarios vary for individual global circulation models (GCMs) as a function of their climate sensitivity. RCP 8.5 is the reference scenario and results in the highest GHG concentrations and temperatures among all RCPs by the end of the century. Any deviation from this pathway (including the other RCPs) is arguably because of actions to reduce emissions (i.e., mitigation efforts).
The second set of data in the framework is the shared socioeconomic pathways (SSPs), which describe development trends and conditions. The SSPs offer plausible alternative tendencies in the evolution of society and natural systems and include narrative descriptions and quantifications of selected socioeconomic variables at the national, regional and global scales. SSP categorization is through the individual pathway’s global challenges to mitigation and to adaptation (Riahia et al., 2017). That is, in each SSP, the level of energy usage, the increases in GDP, trade, population and urbanization growth and the scale of international coordination, among other aspects, provide for either benefits or challenges to climate mitigation or adaptation (O’Neill et al., 2017). This SSP is the most sustainable development pathway with low mitigation and adaptation challenges. SSP 2 results in both slightly higher mitigation and adaptation challenges than SSP 1. SSP 3 defines a pathway where the world faces the highest mitigation and adaptation challenges among all SSPs. SSP 4 describes a world with increasing inequality where mitigation challenges are low, but adaptation challenges are high. Finally, SSP 5 – the high fossil fuel use pathway – includes development patterns such that adaptation challenges are low, but mitigation challenges are high. All SSPs are “reference” pathways and assume no climate change or climate impacts, and no new climate policies (Kriegler et al., 2014).
Together the set of RCPs and SSPs provide tools to explore a wide range of outcomes given socio-economic development and GHG emission concentrations. Mapping an SSP across different RCPs can reveal the relationship between changing climate policies and climate impacts for a particular socio-economic development pathway. Conversely, mapping an RCP across different SSPs can reveal how a specific climate change trend impacts different socio-economic development pathways. There are constraints to combining RCPs and SSPs, however. For example, given the socio-economic conditions in SSP 3, it is not possible to achieve RCP 2.6 (the lowest GHG emissions levels trajectory in our study). Likewise, the GHG levels of RCP 8.5 can only be reached with SSP 5.
2.2. Urban land cover growth simulations
Cities have taken on a multitude of urban forms since their emergence (Kostof, 1991, Morris, 1994). Over the past 200 years, urban growth patterns have differed across cultural regions (Brunn et al., 2016) and across time with technological development, particularly mobility (Newman and Kenworthy, 1999). Some argue that during the era of globalization urban growth patterns in developed and rapidly developing world cities are converging in urban form (Dick and Rimmer, 1998) although others debate this claim (Marcotullio, 2003).
Understanding the drivers and patterns of urban land cover growth is critical to projecting future spatial urbanization patterns. As mentioned, population growth estimates suggest that the world will be increasingly urban. After around 2030, almost all population growth will occur in the world’s cities, as the global rural population is anticipated to decline. While there are population projections for national urban population shares, however, there are a limited number of spatially disaggregated projections. An analysis that examines the exposure of urban populations to heat waves, necessitates spatially disaggregating these national population estimates to local areas (cities, towns, villages).
The few models that exist attempt to use drivers of urbanization to project urban growth. For example, Seto et al., 2012, Güneralp et al., 2017 use an analysis of satellite imagery combined with an urban growth modeling to develop probabilities of future urban land cover growth to 2030 and urban population densities to 2050. Angel et al. (2005) also use satellite imagery to define urban extents and then bases growth on projected future changes in urban densities. This group projects urban land cover change to 2050. Chen et al (2020) provide a set of global urban land use growth estimates to 2100 for all SSPs to examine the impact of urban growth on other land uses. Jones and O’Neill (2016) use a gravity model to spatially project urban and rural populations, to identify the urban demographic change. All of these simulations project urbanization based upon an understanding of previous patterns, drivers, or urban forms. There is, however, no one best simulation, as all face similar challenges including the lack of historical spatial data on which to base future urban growth, the uncertainty of future technologies, governance and cultural factors that affect urban growth, and difficulties of modeling the details of urban land cover growth at regional and global scales.
An alternative technique to project urban land cover growth is to address uncertainty using multiple models that provide a range in growth outcomes. That is, rather than attempting to define urban land growth as a specific pattern, using one model, it is feasible to use different models to develop a range that defines extremes in future outcomes. Arguably, the true future outcome would fall between these extremes. This study uses this assumption to present differences between so-called sprawled and compacted urban development as distinct urban land cover growth patterns. While these ideal categories are notional, scholars suggest that a sprawled development pattern is evident in the US and Australia and that compacted urban development can be found in parts of Europe and Asia (Newman and Kenworthy, 1999). These terms also carry more than just an indication of difference in urban area. Compact urban development for example, can include integrated land cover zoning, transit-oriented development, walkable neighborhoods, traffic calming, eco-city orientation and a host of other elements (Kenworthy, 2006). Sprawled development, alternatively, is often associated with rising incomes, supporting government land use and tax policies or lack of land use regulations and increase use of personal vehicles, among other elements (Nechyba and Walsh, 2004, Sudhira et al., 2004). There are also variants within each type of land cover pattern, as there are different urban forms that provide similar types of densities (Seto et al., 2014). We do not include these differences in policies, governance and social conditions in our definitions. And we do not promote one form as normatively preferred over the other. Furthermore, we do not attempt to assess the plausible responses to heatwaves under different growth pattern (i.e., are heat waves hotter in compact versus sprawled settings), as we do not have the detailed data at the urban scale with regional coverage to perform such an analysis. Rather we use these terms to describe urban land cover patterns that signify the difference in both population densities and urban area at the extremes. The goal is to define a range of the extent of urban land cover with increasing population that might affect exposure to heat events.
2.3. Heat waves
There is no standardized definition or measure of a heat wave (Horton et al., 2016, Perkins, 2015, Dosio et al., 2018). Heat wave characteristics (e.g., intensity and duration) vary over different regions (Perkins et al., 2012) making a universal definition for these phenomena difficult. The most agreed upon definition is that heat waves are observed high temperature extremes over consecutive days at the global, regional or local scales (Alexander et al., 2006, Della-Marta et al., 2007). Some researchers have attempted to define heat waves empirically. One example is an event of at least three days in duration during which the daily maximum temperature starts above the 97.5th percentile of temperature distribution and remains above the 81st percentile of temperature thereafter (Meehl and Tebaldi, 2004).
High temperatures alone, however, make up only a part of what might be considered heat waves. Another component is humidity. Together these weather aspects define how ambient conditions “feel” and arguably provide a better indicator of human comfort than either one alone (Epstein and Moran, 2006). Combing these two factors creates a heat index. The most common technique used in heat index research today is a variant originally proposed by Steadman, 1979a, Steadman 1979b and has subsequently been refined (Anderson et al., 2013). National weather bureaus often define heat waves with a heat index. For example, the US National Oceanographic and Atmospheric Administration (NOAA) National Weather Service’s heat index is called the “Likelihood of Heat Disorder with Prolonged Exposure or Strenuous Activity” index1 and includes categories with accompanying thresholds; Caution~>30 °C, Extreme Caution ~ >35 °C, Danger ~ >42 °C and Extreme Danger ~ >50 °C.
Observers argue that heat waves can be experienced differently by cultures and societies across climate zones (Patz et al., 2005). In a recent global review of heat mortality, however, researchers have defined a threshold level for temperature and humidity at which excess in mortality is experienced across all cultures and climates (Mora et al., 2017). This is not surprising, as there are upper physiological limits for all humans’ and mammals’ exposure to heat (Sherwood et al., 2010).
2.4. Urban heat island effect
For cities, climate related heat concern is exacerbated by the higher temperatures in the urban core as compared to surrounding areas. This is called the urban heat island (UHI) and it provides a clear example of anthropogenic impacts on climate. UHI has been known for some time, as it was identified 200 years ago by Howard (1818) and has subsequently been found in cities around the world (Kataoka et al., 2009). Analysts consider UHI one of the major environmental problems of the twenty-first century (Mckendry, 2003, Rizwan et al., 2008, Arnfield, 2003)
In recent years, four different types of UHI have been defined, including subsurface UHI, surface UHI, canopy layer UHI and boundary layer UHI. All these different UHIs relate to differences between the urban and rural temperatures, but each is measured at different altitudes and with different techniques. The canopy UHI is measured between the surface and the tree canopy or below the average building height of the city with stationary sensors or those mounted on vehicles. For this study we are interested in the canopy UHI, because compared to the other types it has the best correspondence with climate model air temperatures, it is taken at the most appropriate geographic scale and is the most relevant temperature experienced by people. Henceforth, referenced UHI in this research refers to canopy UHI.
In general, UHIs are typically around 3–4 °C (Oke, 1997, Voogt, 2002), but can vary between 0.4 and 12 °C (Santamouris, 2015) and reach 17 °C in inner-city hot spots (Makrogiannis et al., 1998). In many cases, the highest UHI occurs during the summer or warmer seasons (Erell and Williamson, 2007, Makrogiannis et al., 1998, Wang and Hu, 2006), although there are studies that find it is highest during the winter or cooler months (Hinkel et al., 2003, Salvati et al., 2017). Maximum UHI intensities are typically experienced during the evenings (Arnfield, 2003), but in some cases, the maximum UHI occurs during the afternoons (Oguntoyinbo, 1984).
The set of driving factors for UHI include local climatology, street geometry, building fabric and anthropogenic activities. Given the importance of urban form and human activities, UHI intensities for individual cities change as they grow. In the UHI literature, one important indicator for the level of UHI is city size, measured by population, city area or diameter (Oke et al., 2017). Several studies have identified the positive relationship between the urban population and UHI, although the slope of the increase and intercept vary by geographical region (Oke, 1973, Roth, 2007, Jauregui, 1997, Santamouris, 2015).
Exactly how global climate change will affect UHI is a current topic of research, but much remains unknown (Huebler et al., 2007, Roy et al., 2011). A recent study suggests that UHI will increase across all SSPs for RCP 4.5 due to urban expansion (Huang et al, 2019). Others find that both climate change and future urban population size and other factors will determine UHI (Shastri et al., 2017, Tran et al., 2006, US EPA, 2018, Manoli et al., 2019). While difficult to project, a study of future urbanization and heat waves is incomplete without the inclusion of UHI. Rather than forecasting a particular UHI value for a city, this study provides a range of UHI values for cities of different sizes.
2.5. Vulnerability, heat wave sensitivity and the low-income status of those exposed
The evaluation of the full impact of heat waves is a complex task and is approached with different framings and methods (O’Brien, et al, 2009). Scientific framings define vulnerability as a function of the intensity of the shock, the exposure of the population or infrastructure, the sensitivity of the population or infrastructure to that shock and the adaptive capacity of the system to avoid or ameliorate the shock (IPCC, 2014). Alternatively, contextual framings of vulnerability are based on multidimensional views of climate-society interactions. These studies focus on the political, institutional, economic and social structures, their interactions and how they condition the context for exposure, sensitivity and capacity to address climate events (O’Brien et al., 2009, Kelly and Adger, 2000). These different approaches prioritize different types of knowledge, can lead to different types of responses and therefore require explicit recognition.
This study uses a scientific framing of vulnerability. However, it is further limited by data availability. Scientific studies of vulnerability often estimate the potential monetary cost, morbidity or mortality associated with heat waves, and assess the potential ability of societies to cope with or adapt to these events. Therefore, they require detailed local knowledge and information, which is not available for global or even regional projections. Rather than focus on vulnerability, this provides more basic, components of vulnerability. We focus on total exposure and the heat sensitive and low-income population shares of those exposed.
Data available to identify this scientific framing of sensitivity is available through projected population structures (Samir and Lutz, 2017) for the shares of the population younger than 5 or older than 64 to define the heat wave sensitive population (Kovats and Hajat, 2008, Sheridan and Allen, 2018), as a partial indicator of heat wave sensitivity. We also use national income per capita, as incomplete measure of adaptive capacity. Of the exposed population, we identify those living in nations with GDP per capita levels lower than $4,000 across all decades. For the GDP data we use values at purchasing power parity (PPP) at constant US$2005. We use the $4,000 threshold, based upon World Bank definition of the boundary between middle class and above and lower-income and below countries.2 Certainly, adaptation will necessitate financial resources to mobilize public efforts (cool and green roofs, increased open space and vegetation, increased outdoor watering in parks, provision of cooling stations, etc.) (Gill et al., 2007, Harlan and Ruddell, 2011, Hewitt et al., 2014), as well as private efforts (increased air conditioning, social networking during heat events, etc.). Vulnerability assessments typically aggregate indices and almost all include economic indicators (Brooks et al., 2005, Cinner et al., 2018), but adaptive capacity also includes a host of other conditions such as the level of management (particularly governance and strength of institutional responses), access to resources and demonstrated successful historical coping experiences (Smit et al., 2001, Yohe and Tol, 2002). Furthermore, low-income status only partially estimates the impact of low per capita income, as it does not identify the distribution of incomes within nations. There is evidence that, even within wealthy nations, there are significant disparities of urban household incomes (Gornig and Goebel, 2016, Timberlake et al., 2012). By this proxy, therefore we can obtain a sense of the numbers living in conditions with tight governmental and private resources, but we do not claim to identify the population’s adaptive capacity to urban heat wave events. Given the coarse indicators used, the project makes no claim to identify urban vulnerability to heat waves.
3. Methods
3.1. Generation of spatialized heat waves data
We used an established technique to create a heat index (Rothfusz, 1990) by combining mean daily temperature and relative humidity (Anderson et al., 2013) from the outputs of climate models.3 We group the strength of the heat waves into the following categories, <30 °C, 30–36 °C, 36–42 °C, 42–46 °C, 46–50 °C, >50 °C, based upon NOAA’s National Weather Service Heat Index Likelihood of Heat Disorder with Prolonged Exposure or Strenuous Activity and the results of a recent global study on mortality under heat waves (Mora et al., 2017). The threshold of approximately 30 °C, signals conditions that above which result in excess mortality due to heat in some cities. The threshold, at > 42 °C, signals where there will be excess mortality due to heat in all cities. These thresholds bookend approximations of conditions from a recent study that reviewed over 780 cases of excess mortality associated with heat from 164 cities in 36 countries (Mora et al., 2017). In the Mora et al (2017) study, a moving index defined by temperature and humidity defined a “deadly threshold” under which excess mortality during heat waves emerged. From these data, we estimated that the lowest heat index conditions under which excess heat-related mortality emerged was approximately 30 °C. The highest heat index was approximately 42 °C. We use the upper threshold as an indicator of when all societies will experience some excess heat-related mortality during this level heat wave. Finally, rather than defining the duration of a heat event differently depending upon climate, we set a 15-day period to demonstrate the potential for prolonged intense high temperature events.
We based our temperature and humidity values for the heat index calculations on Coupled Model Intercomparison Project Phase 5 (CMIP5) historical and climate projections from the Inter-Sectorial Impact Model Intercomparison Project (ISIMIP) comprehensive compilation of five Global Circulation Model (GCM) outputs from Earth System models (Warszawski et al., 2014). The ISIMIP five cover 1950-2005 (historical period) and future projections up to 2099. The outputs included daily time series for all meteorological variables consistently bias corrected (Hempel et al., 2013) so the spatialized model results from different contributing groups yielded comparable annual baselines for the contemporary period and only deviated in their future projections. We used mean daily temperatures and relative humidity for the calculations. For the heat indices, we computed 15-day running means and tested when the running mean was above our thresholds. The running mean acts as a low-pass filter that attenuated the temperature signal, captured the low frequency variations and therefore expressed the prolonged conditions over the 15-day period of time. From the running means, we identified the warmest 15-day heat indices for each cell for 30-year periods from 1950 to 2009 (current climate), 2010–2039 (near future), 2040–2069 (mid-future) and 2070–2099 (far future).
Using the mean temperatures and not the maximum daily temperatures provides conservative estimates of heat waves. We also believed that these values were more meaningful when adding urban heat island intensities. According to UHI research, urban and rural temperatures were approximately equal during mid-day to early afternoon, which was approximately when urban ambient temperatures were highest. That means that UHI values should not affect maximum temperatures. UHI intensities, however, affected minimum and mean daily temperature and arguably, the UHI intensities can be added to these means to achieve a heat index that roughly approximates the UHI impact. Given that the identified temperature values for the chosen 15-days are the highest temperatures over 30 years, we call them heat waves. Henceforth, we refer to heat waves that exceed the 42 °C threshold as “very warm.”
3.2. Generation of urban land cover simulations
Our urban growth simulations were based on urban population increases and urban densities. The urban populations were taken from the SSPs at the country level. We adopted an approach to urban simulations that considered concentrated (compacted) and dispersed (sprawled) urban growth. The simulation for the sprawled scenario started with the urban extents layer from the Global Rural-Urban Mapping Project (GRUMP) datasets (Balk, 2009), which was a gridded binary classification between urban and rural areas with 30 arc-second resolution (~1 km at the equator). GRUMP defined urban extents as derived from population counts, settlement points and nighttime lights. We used the European Space Agency’s Global Land Cover Map (GlobCover) (Bontemps et al., 2011) for the start of the compacted urban growth simulation. GlobCover, based on data from the MERIS sensor on board the ENVISAT satellite mission, was then classified into 24 land cover classes. From those, we used cells classified as artificial surfaces and associated urban areas. In addition to these two starting layers, we used a national grid that identified the cells in each country using ISO/UN numeric identifier codes, as well as a layer storing the area for each grid cell. This last layer is used to calculate population densities, as cells decreased in size with increasing latitude. All layers were scaled to 30 arc-second resolution. GlobCover was initially of higher resolution, but subsequently scaled to the same resolution as GRUMP. We used these two different starting layers because they were at opposing extremes of urban land cover estimations, with urban land cover area in GRUMP approximately 10 times larger than that of GlobCover (Schneider et al., 2009). These two extremes were chosen to identify the range within urban growth is most likely to stay until the end of the century.
The SSPs included total population and urban share by decade to 2100 for 167 nations (Samir and Lutz, 2017, Jiang and O’Neill, 2017). Each urban growth simulation was performed independently in 10-year steps for each of the 167 nations, starting with the current state in 2010, and running until 2100. National total population changes were calculated by urban and rural distinctions and the results were distributed or removed from cells within each country for each step. For example, for the change in urban population, as each simulation moved 10 years forward (e.g., from 2040 to 2050), the urban extents layer and population layer of the previous step (2040 in this example) were compared to the numbers projected for the following year (2050) in terms of total urban population per country. The numbers in the layers were then adjusted to match the projections from the SSP under consideration, such that population is added to the urban cells (or added or removed from non-urban cells) of each country. Population was added randomly in the urban cells (and added or removed randomly from rural cells), such that the urban and non-urban totals matched the following SSP year (2050) projections at the national level. For both urban and non-urban cells, however, the current population in the cell was used as a weight for the random assignment of new population, so that densely populated cells are more likely to attract additional population than less dense (or completely uninhabited) cells. The random factor was still used as a way to account for potential future development within cells which cannot be accounted for decades in advance, at continental scale.
During the process, a control mechanism was implemented to make sure that population density in a cell did not exceed a realistic limit. Without this control, populations could exceed millions per cell (i.e., millions per square kilometer at the equator). To identify a realistic limit, we used the current urban population densities as defined by the initial maps (GRUMP and Globcover) and set a limit based upon the mean of the 50 highest-density urban cells in the considered country. We argued that these 50 cells are enough to represent the high-end of the density distribution for each country. We then set the limit of 95% of this density for the next step. Thus, the threshold and the decreasing trend depended upon the urban density distribution within each nation. We lowered densities in conformance to research findings of this worldwide trend (Jiang and O'Neill, 2009, Angel et al., 2010, Güneralp et al., 2020).
A rural cell was urbanized if it ended the step with a population density equal to or greater than the national mean for urban cells. Inside urban areas, we re-examined urban cells to determine if they have a density higher than maximum threshold identified in the previous step. If so, population in the cell was automatically set to the threshold value, and the excess population is pushed to neighboring cells. With these steps, urban expansion was simulated by urbanizing cells, i.e., turning them from rural to urban in the urban extents layer. This process was iteratively repeated until no cells exceed the threshold. There were constraints to placing population in cells including no population in water bodies and pre-identified desert and mountain areas.
Fig. 1 illustrates one step in the simulation and analysis process for a given combination of SSP, RCP and urbanization scenario. The population grid and urban/rural grid for the current time step (1) was compared to the urban and rural population from the given SSP at the following time step, moving 10 years forward. The population grid was then adjusted to match the SSP number by adding or removing population from the urban and rural areas (2). For unrealistically densely populated cells, population was then moved to neighboring cells (3), followed by an urbanization step where densely populated rural cells were turned urban in the urban/rural grid (4). This population and urban/rural grid at time step N + 10 were then overlaid with the temperature grid indicating the warmest 15-day heat waves for the given year, extracted from the current RCP (5), allowing us to calculate the exposed urban and total population (6). Fig. 2 presents an example of the results of our two urban land use simulations.
Fig. 1. Overview of one step in the simulation and calculation of exposed population for a given combination of SSP, RCP, and urbanization scenario. The output population and urban/rural grids will be the starting point for the following simulation step going to N + 10.
Fig. 2. Example of the difference between in results between the sprawled urban land cover model outcomes and the compact urban land cover model outcomes for parts of West Africa.
3.3. Urban heat island analysis
We obtained UHI intensities from a review of 131 studies for 135 cities around the world. We identified these studies through a 3-step process. First, we identified UHI research reviews from 1979 to 2018 through a search of ISI Web of Science using the phrase “urban heat island”. From the approximately 178 review results we examined 30 reviews that had some part related to canopy UHI measurements. From these reviews, we identified highly regarded case studies (those included in two or more different reviews) with UHI results. In the second step we examined these highly regarded studies for results. Finally, we used the snowball method, in the third step, to identify references used in the highly regarded studies for further UHI data. In total, over 200 studies were examined. The results for several studies were incomplete and therefore not included in the analysis.
We used the UHI and city size data reported in the final 135 case studies and combined these data with Koppen-Geiger climate zone classification and population estimates for each city. We added the climate zone classifications because of the small number (eight) of published results identified for African cities. To get a representative sample of UHI intensities, therefore, we used results from cities located in similar climate zones found in Africa for our UHI analysis. This resulted in over 110 UHI values. We further divided the cities by size and obtained the mean and standard deviation UHI (Table 1) (see also Manoli et al., 2019). In our final results we presented both heat indices with and without the addition of UHI to get a range of outcomes.
Table 1. Urban heat island sample statistics.
3.4. Low-income national status and sensitive populations
Data for national GDP per capita was available by SSP from the results of the IIASA GDP model (PPP at US$2005).4 To identify the nations which might have resources for mitigation, we used the World Bank’s 2010 threshold for low-middle income of approximately US$4,000 GDP per capita.5 This cutoff, applied to the GDP per capita data from the SSPs for 2010, identified approximately the same share of countries as did the World Bank during that year (World Bank’s share was around 37% of 218 nations and ours was about 41% of 167 nations) (World Bank, 2019). We applied a similar allocation of low-income status share to urban and rural populations. We then used this threshold across time, suggesting that this low-income indicator did not change, although some studies suggest that poverty levels shift upward over time (Hoy, 2016).
Of the 52 African countries in the IIASA GDP database, 50 had data on GDP per capita. Of these, approximately 80% were below $4000 per capita in 2010 and these nations held over 810 million total and 290 million urban low-income populations (Table 2). The SSP 1 and 5 defined rapid decreases in the number of low-income nations and total and urban populations. SSP 2 outlined a similar trend, but in slower fashion. SSPs 3 and 4 defined increases in low-income total and urban populations over time. These trends were commensurate with the descriptions of the SSPs above. Using the (2005) $4,000 threshold, by 2100, low-income nations no longer exist in SSPs 1, 2 and 5. That is, in these development pathways all nations on the continent have GDP per capita levels above $4000 by 2100.
Table 2. Low income African countries, total and urban population.
Data are for number of countries and total and urban population in millions.
The sensitive population was identified as the share of population in each country of a certain age (<5 and > 64). Given that the population age structure was available for each SSP by gender for each 10-year step, we calculated the numbers of people in this category for each nation. We then applied the share of sensitive population uniformly across urban and non-urban cells. The sensitive population made up about 19% of the African population in 2010, which translated into 191 million total and 76 million urban persons. The shares of these populations changed with the different SSPs, increasing dramatically in SSPs 1 and 5 to 40% by 2100 and remaining fairly flat throughout the century in SSPs 3 and 4 (Table 3).
Table 3. African sensitive population by SSP.
3.5. Application of the framework
After spatially allocating urban population derived from the SSPs, we overlaid the heat indices derived from the 5 GCMs in ISIMIP for each RCP, to identify the location of urban areas and residents projected to be exposed to the heat waves of different intensities for the different urban simulations (compacted and sprawled). We compared results along RCPs for the different appropriate SSPs to show how climate change impacts played out under different socio-economic futures (Ebi et al., 2014). We presented the most likely estimates (means), and range high and low estimates from the different GCMs, for each analysis. Because SSP 1 is considered the sustainable pathway, we reported figures for this SSP across appropriate RCPs and compared them with other pathways.
3.6. Sources of uncertainty
The numerous data sources and assumptions made in the different analyses introduced uncertainties in our outcomes. The sources of these uncertainties are found in the four major tasks of the research including the: 1) quantitative socio-economic projections; 2) heat wave estimates; 3) urban land cover expansion and urban population projections; and 4) UHI estimates. Within each SSP, there is uncertainty in the quantitative projections from the interpretation of the SSP narratives, as well as from the models that have generated the quantitative projections (Riahia et al., 2017). Moreover, our choices of indicators for sensitive and low-income populations (only using age, for example) introduced uncertainty in the results.
The use of an ensemble of GCMs was an attempt to capture uncertainty. In this case, we attempted to quantify the resultant uncertainty by presenting the most likely estimate (mean) and the entire range found within the ensemble of model outputs (using the symbol “±” for heat indices and reporting the max and min values for exposure estimates). Our categorization of heat wave intensities (>42 °C) and choice of heat wave duration (15-days) inevitably lead to conservative numbers of exposed populations. For both the RCPs and SSPs the combination of all the different pathways attempted to account for a wide variety of possibilities. No one pathway was prioritized, and the entire framework helped to identify the range of possible futures.
Uncertainty was also introduced in the urban land use expansion models. These were particularly difficult to address, as we were unsure of how future technologies, policies and behaviors could change urban patterns. Furthermore, the identification of what was urban was challenging. We attempted to address this through the use of two different urban extent simulations (compact and sprawled), which provided a range of outcomes. Our modeling approach for both urban land cover growth depended on a binary distinction between urban and non-urban areas (instead of a graded measure of level of urbanization per unit) missing much of the detail of urbanism. We also assumed urban expansion follows urban population growth rates. There are several sources of uncertainty in this assumption, most notably the reduction of urbanization to population density, as well as the limited resolution of 30 arc-second grid cells, which demanded a significant simplification of the urban structure within each cell. Finally, we randomly distributed urban population rather than privileging specific cities. We applied a weighting system, where higher density cells have higher probability of receiving more population, but that was applied to all cells. Identifying where economic activity will blossom was inherently difficult. Ultimately, we presented and compared the highest and lowest heat indices in both the sprawled and compacted models to find a range of exposure values and averaged the results from the two models to get a most likely outcome.
In terms of our UHI estimates, while there is abundant research on UHI, the methods used in studies may not be similar (Stewart, 2011). As such, our grouping of canopy level estimates introduced uncertainty. At the same time, we followed UHI experts’ examples by categorizing cities by population to predict UHI intensities (Oke, 1973, Roth, 2007, Jauregui, 1997, Santamouris, 2015). To address uncertainties in UHI, we estimated heat indices both with and without UHI values.
4. Results
4.1. Urban land cover simulations for Africa
Empirically, the SSPs define three general population patterns for African urbanization through the twenty-first century (Table 4). The first pattern is presented in SSPs 1 and 5, where urban growth proceeds rapidly during the first half of the century, tripling the total urban population. Then, during the second half of the century, total urban population growth decreases dramatically, resulting in a regional urban population of approximately 1.7 billion (91% of total population) by 2100. The second pattern, exemplified by SSPs 3 and 4, includes rapid urban growth during the first part of the century, and continued growth but at slower but steady growth rates during the second half of the century, at which time urban populations reach 2.0 and 3.3, respectively. Due to high total population growth in SSP3, by 2100, the urban share is only 51% of total population. In SSP 4, by 2100, the urban share is similar to SSPs 1 and 5 at 91% of total population. The third pattern, demonstrated in SSP 2, is mid-way between these SSP 1 and SSP 4 and includes steady urban growth throughout the first half of the century. During the second half of the century the growth rate drops by more than half resulting in approximately 1.9 billion urban dwellers making up 73% of the total population in the region in 2100.
Table 4. Projections for African urban population and urbanization levels by SSP, 2010–2100.
Growth patterns for the two urban land cover simulations result in different numbers of cities, total urban area and mean densities (Table 5). Total urban area in the sprawled model is an order of magnitude larger than in the compact model, therefore the urban densities in the compact model are typically higher than those using the sprawled model. The number of urban areas grows more rapidly in the sprawled model than the compact model due to the algorithm specifications. Urban area growth also varies across SSPs, but not as starkly as population. This is due to the fact that in our simulations, while population can decrease, urban area does not.
Table 5. Simulated African urban area expansion (Km2), increase in number of urban extents and change in mean densities by urban land use model and SSP.
The urban land cover simulations result in two patterns across SSPs. On the one hand, urban area growth continues throughout the century resulting in similar amounts of urban land cover for SSPs 1, 2, 3 and 5. In these cases, by 2100, urban land cover varies between 840 and 935 thousand km2 (sprawled) and 91 to 103 thousand km2 (compact). On the other hand, by 2100, in SSP 4 urban area growth is the greatest leading to over 1.4 million km2 in the sprawled model and 156 thousand km2 in the compact model. By the end of the century, the number of urban areas also is largest in SSP 4 for the sprawled model (over 63 thousand) and is similar across SSPs 1,2 and 5 (around 31–32 thousand). SSP 3 had the intermediate number of urban areas (approximately 45 thousand). The same relative pattern, with smaller numbers of urban areas was exhibited in the compact simulation.
As a result of the different size of urban areas, the urban densities vary between the different simulations. By 2100, in SSPs 1, 2 3 and 5, the compacted urban growth model the average densities are approximately 3.8–4.5 times that of the averaged sprawled model. In SSP 4, the compacted simulations result in average regional densities that are almost 6 times as dense as the sprawled simulations.
4.2. Future heat waves
Over the course of the century, the average regional 15-day urban heat wave index climbs for all RCPs, although for RCP 2.6 heat indices level off between 2030 and 2070 for the rest of the century (Fig. 3). The greatest increases are seen in the higher RCPs. There are also large differences in average heat indices depending upon whether UHI is included or not. For example, during the current period, the estimates suggest that the mean warmest 15-day heat wave is approximately 32.7 °C (±2.1 °C) without UHI and with UHI is approximately 36.9 °C (±2.2 °C). By the end of the century, in RCP 2.6 and the sustainable development pathway (SSP 1) the mean 15-day heat waves are estimated to increase to 34.6 °C (±2.4 °C) without UHI and 38.7 °C (±2.4 °C). At the high end, for the high fossil fuel use development pathway (SSP 5) and RCP 8.5, the mean 15-day heat wave is projected to increase to 42.5 °C (±3.9 °C) without UHI and 46.6 °C (±3.9 °C) when UHI is included. For SSPs 2–4 for RCPs 4.5 and 6.0, by the end of the century, the mean 15-day heat wave index is projected to rise to between intermediate and high levels. Estimates for without UHI suggest levels of between 36.8 °C and 42.2 °C, while with UHI projections reach between 40.6 °C and 43.1 °C (Table 6 Supplement). Across RCPs, the African sub-region with the warmest 15-day heat indices is Western Africa, where the heat index rises higher than projected for the Northern and Middle sub-regions (Fig. 4).
Fig. 3. Average heat index for 15-day heat waves in cities of Africa by SSP, RCP and with and without UHI values added.
Fig. 4. Mean heat indices for cities in Africa by sub-region, SSP, RCP and with UHI and without UHI values. The red colored curves are for Western Africa. (For interpretation of the references to colour in this figure legend, the reader is referred to the web version of this article.)
4.3. Future exposure
The findings suggest large differences in African population exposure levels to very warm heat waves between simulations with and without the additional UHI effect (Fig. 5). For example, projections without the UHI effect suggest that in SSP 1 and RCP 2.6, the numbers or urban residents that could experience very warm conditions will increase from approximately 18 million (range: 3.0–47 million) in 2010–313 million (range: 111–608 million) in 2100. With added UHI, the numbers exposed start and end much higher: from 136 million (range: 87–191 million) in 2010 to 947 million (range: 542–1367 million) in 2100 (Table 7 Supplement). The numbers exposed to these warm conditions increase with RCP. In RCP 4.5, for example, exposure exceeds 600 million for SSPs 1,2,3 & 5 by 2100, and exceeds 1 billion for SSP 4, even for heat indices without the UHI effect. With the UHI effect exposure levels reach or exceed 1 billion for all SSPs for all RCPs.
Fig. 5. African urban population exposed to various levels of 15-day heat intensity by SSP, RCP and with and without UHI values.
The figures of exposure to high index heat waves absorb a large share of the African urban population. In SSP 1 – RCP 2.6, the percent of the African urban population exposed to very warm heat waves rises from 4% in 2010 to over 18% of the total urban population when UHI is excluded from the analysis. Including UHI in the heat index, the share rises from 36% in 2010 to over 55% in 2100. In other scenarios that include UHI, the share of the urban African population that experiences very warm heat events typically exceed 50% of the population by 2100. Furthermore, much of the increase in numbers in this category is due to increases in exposure to the extremely warm heat waves (>50 °C). When UHI is included in the heat index, the share of those exposed to heat waves of >50 °C rises from very low levels (around <2%) in 2010 to, in some cases, over 40% of the urban population. For SSP 5 and RCP 8.5, this share exceeds 56% and includes over 490 million people (or more than the total urban African population in 2010).
In each projection, while the numbers exposed to the milder level heat waves (<30 °C) increases across the century, the shares of this population decrease. For example, in SSP 1, RCP 2.6 including UHI, the numbers exposed to <30 °C heat waves increase from 38.2 million in 2010 to approximately 133 million, but these values translate to approximately 9.3% of the total African urban population in 2010, and 7.8% of the total African urban population in 2100. These increases in populations and declines in share are projected across all SSPs and RCPs and suggest population growth is projected to occur in areas prone to warmer heat events.
Using UN sub-regional distinctions (Fig. 6) for a sub-regional level analysis suggest that those in Western Africa have the highest numbers of exposed populations to very warm heat waves. In this analysis we compare SSP 1 and SSP 4 results across heat wave categories, RCPs and with and without the addition of UHI. Fig. 7 demonstrates that in both pathways and across RCPs, Western African urban populations make up the lion’s share of those projected to experience the higher intensity heat waves compared to residents in other parts of the continent. Those in Eastern Africa, alternatively will have the largest populations exposed to heat waves of <30 °C and 30–36 °C. As can be seen by the charts, SSPs 4 present cases of highest exposure levels to extremely warm 15-day heat waves of >50 °C. For SSP 4 and RCPs 4.5 and 6.0, projections, which include UHI, results project that the total number of urban Africans exposed to these intensities by 2100 may exceed 1 billion and most of these populations may be located in Western Africa.
Fig. 6. Sub-region in Africa, as defined by the UN.
Fig. 7. Bar charts demonstrating the difference in exposure to very warm heat waves by sub-region in Africa for SSPs 1 and 4 by RCP. The translucent bars are for exposure without UHI and the opaque are for exposure with UHI.
4.4. Future heat sensitivity and low-income populations
The African share of the elderly and very young population exposed to very warm heat waves varies across SSPs and RCPs (Fig. 8). From 2010 to 2100, in RCP 2.6 and SSP 1, without accounting for UHI, the number of sensitive populations in African cities exposed to very warm heat waves increases>40-fold, from around 2 million to 87 million (Table 8 Supplement). If UHI is included in the heat indices, the figures are more dramatic, suggesting an increase from 27 million in 2010 to over 360 million (or approximately 54% of the regional sensitive population) by 2100. By 2100, the highest shares of sensitive populations exposed to very warm heat waves are projected for the SSP 5 pathway, across all RCPs. For RCP 8.5 and SSP 5, by 2100, the numbers of sensitive population exposed to very warm heat waves climbs to over 380 million (range: 241–471 million) for the simulations without UHI and to over 440 million (range; 331–560 million) when UHI is included in the projections.
Fig. 8. Sensitive population exposed population to very warm 15-day heat waves by heat wave intensity, SSP and RCP and with and without UHI.
The population living in the lower income nations changes during the twenty-first century, due to projected economic growth that increases economic production faster than population growth (Fig. 9). These conditions are seen in SSPs 1 and 5, where by the end of the century, there are low numbers of countries with GDP per capita at or below $4,000. Even in SSP 2, projections suggest that the number of low-income Africans decreases over time. At present, approximately 8 million low-income African urban residents are exposed to very warm heat waves, if UHI is not included in the analysis. If the UHI effect is included, the numbers of low-income residents exposed to very warm heat waves almost equals the total African urban population exposed to these events. That is, with UHI added, most of those exposed to very warm heat waves during the current period are living in low-income countries (Table 9 Supplement).
Fig. 9. Low-income population exposed population to very warm 15-day heat waves by heat wave intensity, SSP and RCP and with and without UHI.
Alternatively, for SSP 3 and 4 economic growth is slower than population growth inducing an increase in low-income populations. As such, the low-income population climbs throughout the century in these pathways and with it the numbers of those exposed to very warm heat waves (Fig. 7). This suggests that changing development strategies will have an enormous effect on exposure and adaptation potential. While the numbers of low income exposed to very warm heat waves decline to 0 by the end of the century in SSP 1, 2 and 5, they increase in SSP 3 and 4.
5. Discussion
5.1. Projected African urban land use
The goal of using a sprawled and a compacted scenario for simulating urban land use growth was to delineate the range of possible futures for urban area expansion. There are three published urban land use projections for the globe that include Africa, which can test whether our models achieve the objective of providing the outside range of estimates. The first published future estimate for Africa starts with 41,450 km2 urban areas in 2000 and projects a 5.9-fold increase by 2030 to 244,000 km2 (Güneralp et al., 2017, Seto et al., 2012). This model starts with the global urban extent circa 2000 from National Aeronautics and Space Administration’s Moderate Resolution Imaging Spectroradiometer (Schneider, Friedl, & Potere, 2009). After modeling population densities, the research utilizes a spatial urban growth model, based upon the densities and surface slope, distance to roads and land cover as the primary drivers of land change. The second future urban land cover estimate for Africa starts with approximately 53,000 km2. This model has three scenarios based upon proposed decreases in density of over time (0%, 1% and 2% annually). By 2030, this second model projects between 96,000 (low) and 175,000 (high) km2 of urban areas and by 2050 projects between 154,000 (low) and 418,000 (high) km2 of urban area on the continent (Angel, 2012, Angel et al., 2010). In a recent set of urban land use projections that explicitly use the SSP framework Chen et al. (2020) present the scenario projections of global urban land expansion at a fine spatial resolution of 1 km. This research starts at 2015 with urban land estimates provided by the Global Human Settlement Layer (GHSL) dataset. Future urban land use growth to 2100 is estimated by a regression model with factors of population, urbanization rate (percentage of urban population to total population) and gross domestic product (GDP). The project provides data by 10-year steps for public analysis (Chen et al., 2019).
While the first two models span a considerable range of urban expansion scenarios depending on the corresponding model assumptions, the Chen et al., 2019, Chen et al., 2020 analysis produces a very tight range of possible urban expansion values for the African continent. Notably, the results are very close to, yet slightly above, our compacted scenarios. Fig. 10 shows how the different model outputs for Africa relate to each other and to our estimates. Given these comparisons, the intended results of the simulations, to capture plausible urban land use growth between sprawled and compacted simulations, given current estimations has been successful.
Fig. 10. Comparison of our compacted and sprawled scenario with the projections by Seto et al., 2012, Angel et al., 2010, and Chen et al. (2020). The ranges derive from the five SSPs (for our projections and Chen et al. 2020), quartiles of urbanization probabilities > 25% (Seto et al. 2012) and three different assumptions for the decrease in urban density 0%, 1% and 2% (Angel et al., 2010). The projections by Seto et al., 2012, Angel et al., 2010 are only available up to 2030 and 2050, respectively.
We further examine a fourth model by Jones and O’Neill (2016), which has become widely used in studies involving future urban populations. Their approach, however, focuses on urban and rural populations on a grid distributed by a gravity-based downscaling model. As such, their model does not rely on clearly delineated (and potentially expanding) urban extents but allows for urban and rural populations to co-exist in the same grid cells. There is no indication of the size of urban land use or boundaries of urban areas in this study. This makes comparison with our outputs difficult. Finally, a recent study of urban land use and heat exposure for Africa used a combination of the above scenarios Rohat, Flacke, Dosio, Dao, and van Maarseveen (2020) delineated future cities' boundaries based on declines in urban density were informed by historical trends (Angel et al., 2016), existing scenarios of urban densities in Africa (Angel et al., 2010, Güneralp et al., 2017) and assumptions of spatial patterns of urban development under the SSPs (Jiang and O’Neill, 2017, Jones and O’Neill, 2016). The land use change models, however differed by SSP in terms of rates of population density change. To get population sizes, these land use maps were then combined with spatially explicitly projections of urban population for the different SSPs (Jones & O’Neill, 2016).
5.2. Projected urban heat in Africa
It is more difficult to compare our future projections of heat waves with other models due to the different methods to compute heat waves and the techniques to present the data. General trends across studies, however, are consistent with our results. Research demonstrates that in a future warmer climate, African heat waves will not only become more frequent but also increase in duration and intensity (Ceccherini et al., 2017, IPCC, 2012, Nangombe et al., 2018). (Dosio, 2017) employing the HWMId index (Russo et al., 2014, Russo et al., 2015) presents projections of temperature and the frequency and intensity of extreme warm events for Africa based on the results of a large ensemble of the World Climate Research Programme COordinated Regional climate Downscaling Experiment (CORDEX) Regional Circulation Models. He finds that by between 2071 and 2100, under RCP8.5, warming for Africa can reach 5–6 °C and that the gulf of Guinea, the Horn of Africa, the Arabian peninsula, Angola and the Democratic Republic of Congo are expected to face, every 2 years, heat waves (defined by the model) of length between 60 and 120 days. This study also projects for these areas that by the end decades of the twenty-first century, the total length of heat spells projected to occur normally (i.e. once every 2 years) under RCP8.5 may be longer than those occurring once every 30 years under the lower emission scenarios.
Rohat et al. (2020) project that the annual number of days when the heat index (including temperature and humidity) exceeds 40.6 °C (what they term is “dangerous heat”), averaged across 150 African cities, increases under all RCPs until the 2060 s and then stabilizes for RCP 2.6 and RCP 4.5, but at different levels. The number of days in exceedance of this heat index continues to rise under RCP8.5 through to the end of the century. By the 2090 s, the number of days in exceedance of 40.6 °C could reach 59, 82, and 123 annually for RCPs 2.6, 4.5 and 8.5, respectively. These patterns in intensity and trends among different RCPs are similar to what is found in this study. The Rohat et al. (2020) study also find, similar to this study, that the cities of Western Africa are by far the most severely affected by, what they call, dangerous heat.
5.3. Projected urban heat exposure in Africa
There are fewer studies that have identified population exposure, in terms of total urban population to future heat. A recent global study identified the land area that will be above a ‘deadly threshold’, identified with temperature and humidity levels, at the global scale (Mora et al., 2017). Based upon the estimated land area affected, they report that while around 30% of the world’s population is currently exposed to their “deadly” threshold for at least 20 days a year, by 2100, this percentage may increase to ~ 48% under a RCP 2.6 scenario, and ~ 74% under a RCP 8.5 scenario. They also point out that that exposure to these levels of heat is concentrated in tropical areas, and that most of the land area in this climatic zone will be over the threshold every day of the year by 2100.
Rohat et al (2020) in their study of large African cities find exposure to “dangerous heat” (exceeding 40.6 °C) will increase by a multiple of 20–52-fold, depending upon the SSP. By the 2090, their results suggest a range of 86–217 billion person-days per year. They also demonstrate the concentration of exposure in cities of Western Africa. Importantly, however, this study did not include UHI in their final estimates and as the authors suggest, the final results are therefore conservative. Nevertheless, both of these studies point to the potential high exposure levels in Western Africa and the high potential population exposure levels for the continent.
5.4. Projected UHI in African cities
Recent research has simulated future urban growth to project UHI increases across arid, cold, tropical and temperature climate zones for RCP 4.5–2050 (Huang, Li, Liu, & Seto, 2019). In this study, the intensity of UHI for summer daytime and nighttime warming increased on average between 0.5 and 0.7 °C, but could reach ~ 3 °C in some locations. By 2050, UHI related warming is on average about half, and sometimes up to two times, as strong as that caused by greenhouse gas (GHG) emissions for the RCP. The extra urban expansion-induced warming increases extreme heat risks for about half of the future urban population, primarily in the tropical Global South. As S. Chapman, Watson, Salazar, Thatcher, and McAlpine (2017) explain, however, UHI responds differentially to cloud cover, wind speed, evapotranspiration and anthropogenic heat release and therefore can certainly increase with climate change, but may also decrease, as rural areas warm more than urban areas (see also, Oleson, 2012, Oleson et al., 2011).
In another recent analysis, UHI is associated with population size (a proxy for infrastructure) and mean annual precipitation (Manoli et al., 2019). This study analyzed surface land temperatures in 30,000 cities globally, in an attempt to identify a scaling law for UHI in cities. The model is based upon the argument that, as a city grows, its structure and function are predictably modified (Bettencourt, Lobo, Helbing, Kühnert, & West, 2007). As with Huang, et al (2019), the study suggests that as UHI will continue to increase as urban population increases. The Manoli et al. (2019) study further adds precipitation patterns into the equation.
Our methods also suggest that UHI increases with urban population size and hence urban area, but not with increasing temperatures or precipitation. Nevertheless, our study points to the significant addition of UHI effect for exposure to heat waves. For example, in SSP 1, RCP 2.6 without the UHI values, the projections suggest that by the end of the century approximately 18.4% of the total urban population in Africa will be exposed to very warm heat waves, but with UHI, the share increases to 66%. In terms of population number, the addition of UHI triples the number of urban residents exposed (from 310 to 947 million). The additional heat from urban heat islands is a significant factor in increasing the number of residents exposed to these intense heat waves. It is also interesting to note, however, that for larger changes in climate (RCPs 4.5, 6.0 and 8.5) the differences in share and total population exposed to very warm heat waves with and without UHI decreases. For example, by 2100 in SSP 5, RCP 8.5, the total numbers of African urban residents exposed to very warm heat waves reaches about 1 billion without adding the UHI factors, and approximately 1.17 billion with UHI and the percent share increases from 61% to 71%, respectively. This is probably due to high numbers of residents already in the very warm category even without UHI.
5.5. Projected urban heat sensitivity and the impact of lower resources
Urban Africa is highly vulnerable to climate change (Kareem et al., 2020). Recent reviews of the impact of climate change on heath in Africa find that heat waves will result in increased vulnerability (Asefi-Najafabady et al., 2018, Chersich et al., 2018, Pasquini et al., 2020), especially among children, elderly, patients taking anti‐cholinergic medications and patients with disorders of cornification (Uejio et al., 2011, Zhang et al., 2017). These climate change vulnerabilities for African populations are compounded by poverty (Simon, 2010).
Unfortunately, there is less quantitative research findings on the number of future climate sensitive populations in the region, particularly in cities (Simon & Leck, 2015). One global study that examined the difference in impact of 1.5° and 2.0 °C climate change highlighted the potential multiple impacts of climate change on vulnerable populations in the region (Byers et al., 2918). This study found that for populations vulnerable to poverty, climate exposure is an order of magnitude greater (8–32x) in the high poverty and inequality scenarios (SSP3) compared to sustainable socioeconomic development (SSP1). Their findings suggest that while 85%–95% of global exposure falls to Asian and African regions, populations in these continents have 91%–98% of the exposed and vulnerable population. In their study, higher warming scenarios, result in Africa’s growing share of the global exposed and vulnerable populations, ranging from 7% to 17% at 1.5 °C, doubling to 14–30% at 2 °C and again to 27–51% at 3 °C.
Here our estimates are crude, but comparable to these estimates. By 2100, at the low end, approximately 87 (range: 19–262 million) are projected to be sensitive to heat and none will be living in low income nations. At the high end, approximately 377 million (range: 247 – 460 million) of these residents are projected to be sensitive and 464 million (range: 326–634 million), 23% of the exposed population, may be living in low-income nations.
5.6. Summary and implications
Our most likely estimates for exposure to very warm heat waves by 2100, vary with the level of climate change, development pathway, the inclusion of UHI and the urban land cover growth patterns, suggesting a final range of between 310 million (range: 111 – 608 million) for SSP 1, RCP 2.6 with heat indices excluding UHI and 2.0 billion (range: 1277–2679 million) SSP 4, RCP 4.5 with heat indices including UHI. The large ranges suggest significant uncertainty, but there is no doubt that large populations will be exposed in the future. Even in the most sustainable development pathway and lowest climate change levels, both the numbers of urban residents exposed and the numbers of sensitive in the population are projected to climb compared to the current period. This result is probably due to both population growth and climate change. That is, the projections suggest African population growth in already very warm places.
In our study the differences in mean and range of total, sensitive and low-income exposed vary slightly between our sprawled and compacted urban models. This could be because of the resolution of the climate model outputs, which is not fine enough to identify the difference in urban land cover. Heat waves are typically events affecting larger scales than city boundaries, suggesting that the sprawled aspect of urbanization may not affect the total numbers of those exposed. Alternatively, there are studies that suggest that more compact urban landforms, without vegetation, may be warmer than less dense vegetated urban areas (Bechtel et al., 2019). The Bechtel, et al (2019) study used a dataset of 50 cities to examine differences in surface urban heat islands within different intra-urban local climate zones (LCZ). They compared urban signal across different type (from the rural to high density compact) to find higher land surface temperatures or the compact and commercial/industrial LCZ types than for the more rural and higher vegetated areas. This study is suggestive that more compact cities may be warmer than sprawled cities, but more research in this area is necessary to assess the impact of urban form on heat exposure.
The results of our study call attention not only to the critical importance of curbing climate change, but to the potential impact of varying socio-economics on Africa urban heat exposure. Changing SSP pathways makes a large difference in the numbers of those projected to be exposed to very warm heat waves, even within the same RCP. The difference in numbers exposed to very warm heat waves can more than triple between the SSP 1 pathway and the SSP 4 pathway by the end of the century.
Moreover, changing climate warming trends is important not only to reduce heat exposure, but also because of potential indirect and synergetic effects. High heat can reduce crop yields (Siebert & Ewert, 2014), potentially forcing people from rural areas into cities (Kanta et al., 2018). Increased heat can also affect the delivery of electricity, if air and water can no longer cool thermal power plants, potentially reducing energy provision for air conditioning-based cooling (Schaeffer et al., 2012). Studies also have identified high temperature impacts on biodiversity (marine heat waves) potentially reducing potential protein sources (Smale et al., 2019), wildfire risk (Parente, Pereira, Amraoui, & Fischer, 2018), electricity demand (Miller, Hayhoe, Jin, & Auffhammer, 2008), water supply (Zampieri et al., 2016), economic productivity (Dunne, Stouffer, & John, 2013) and urban infrastructure (Chapman et al., 2013). As such, the results of this study provide a critical, but partial, picture of climate-related heat impacts on cities.
6. Conclusions
This project finds that heat waves of increasing intensity will be part of the future for large numbers of African urban residents. The results from using different socioeconomic and climate pathways suggest, however, a variety of plausible futures. Some scenarios suggest growing numbers of exposed to very warm conditions, high numbers of sensitive urban residents exposed to such conditions and low resources to provide coping solutions. Other pathways suggest less dire overall conditions. The element that is common across all scenarios in the ensemble is the increase in numbers exposed to very warm heat waves. This will be the future for urban Africa unless the world embraces more dramatic changes to climate forcing activities than presented our scenarios.
These results, as per the title, are exploratory and while they present a vivid picture, they are only a partial glimpse of what can happen. There is a large number of other impacts from heat waves that are unexplored in this study. We also do not evaluate the full extent of vulnerability and potential adaptation options. We base our work upon a scientific framing of climate change vulnerability. To generate a more holistic understanding of heat vulnerability, it critical to engage in contextual framings that examine the socio-economic and political complexities of vulnerability in this region. A variety of different research projections, including different framings, are required to unpack the drivers and identify policies for remediation. This project suggests the demand for this work is urgent.
Recommend
About Joyk
Aggregate valuable and interesting links.
Joyk means Joy of geeK