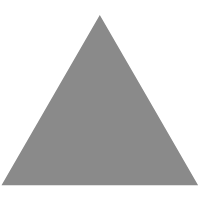
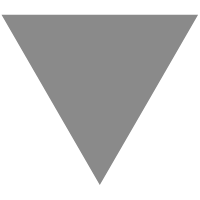
Why do I keep saying that you need to practise?
source link: https://towardsdatascience.com/why-do-i-keep-saying-that-you-need-to-practise-6ee6b74ec5a0
Go to the source link to view the article. You can view the picture content, updated content and better typesetting reading experience. If the link is broken, please click the button below to view the snapshot at that time.
Why do I keep saying that you need to practice?
Or why practising makes you better

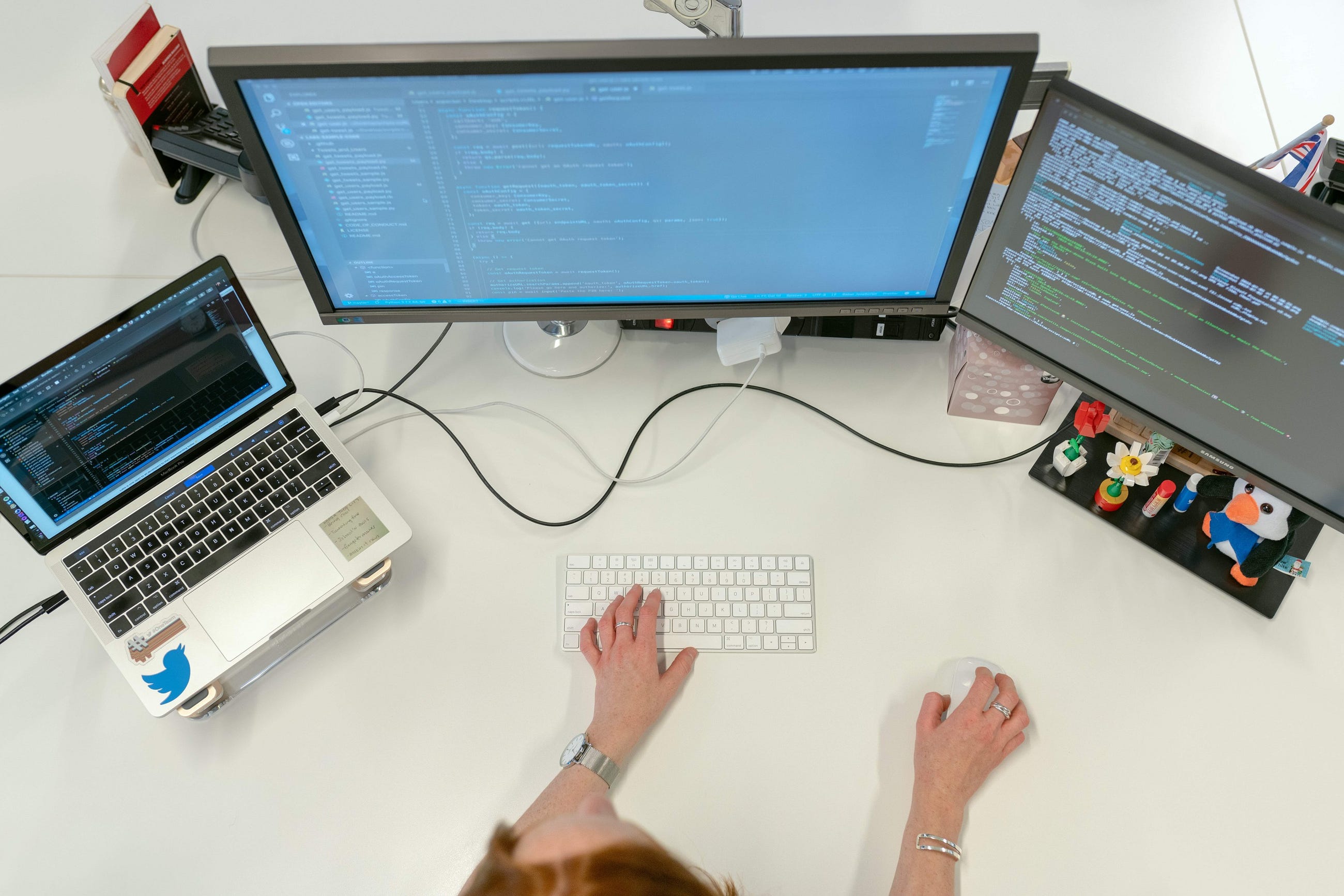
There are tons of courses out there to learn data science. Unfortunately, most of them teach you data science skills only in theory. To become a fully formed data scientist you need to go further than theoretical online courses, you need practical knowledge.
This might sound familiar to you if you have taken my data science kick-starter course where I mention the three crucial levels of data science knowledge; theoretical knowledge, technical knowledge and practical knowledge.
Theory and technical knowledge are things you can simply pick up from online videos, courses and instruction. On top of these, you need to practice to acquire practical knowledge. I define practical knowledge as knowing how to bring together everything you’ve learned and being aware of the data science way of doing things.
In most sources, practical knowledge is portrayed as having a portfolio. Of course, if you practice, you will have a collection of things you’ve worked on and this will help you show off your skills in the form of a portfolio when you’re looking for jobs. But practising hands-on is much more than that. It is a way to become a better data scientist. After all, data science is a hands-on job.
Let me break down why I always recommend aspiring data scientists to focus on hands-on work.
Gaining experience
Of course! You get experience by practising. Sounds very obvious right? But what kind of experience? And what is experience anyway? What I mean by experience especially in the context of data science is; you learn what problems might occur.
Anticipating problems is very important in data science as the mistakes you make could be subtle. Likely there will not be an error screen popping up on your screen. You might not even realise you did something wrong until someone points it out.
When you practice on a personal project, you get to experience these mistakes. You make them, find them and understand why and how they occur. So that next time, you will be more sensitive to the possibility of making the same mistakes and you will take the necessary precautions. Even if that’s just a different way of looking at your data.
This is one of those things in data science that you cannot learn by passive learning (reading or watching videos). You just have to get your hands dirty.
Learning how to learn things
I keep saying this: you do not need to know or even feel like you know everything about data science to start doing projects. You just need fundamental knowledge and a can-do attitude. When you first start off there will be tons of things you don’t know. And that is great. This will be training for you to learn how to acquire this new knowledge.
After I finished my degree on computer science, my friends and I often joked that what we learned throughout our degree was how to Google things. That’s because it was a very hands-on degree and our professors kept pushing us to learn things that were sometimes not even covered in lectures.
When learning data science, you have to be your own professor and push yourself. If you run into something you don’t know, Google it and look through the answers until you get your explanation. This might take some time in the beginning but in time, you will get more efficient in finding answers. This will be great practice for when you become a professional data scientist.
Calibration
I’ve talked about the problem of endless learning in data science before. It is a vast subject with many branches that go as far deep as you could follow it. That’s another benefit of practising. When you have a project in mind, it will have a goal. And when you have a goal in mind, it will keep you focused and tethered to reality… The reality of what you need to know as a data scientist.
Yes, you can always learn more but what I’ve seen in my career so far is that being efficient and effective is 90% of the job.
Practice will help you understand how much and how deep of a knowledge you need. Focusing on finishing the project will stop you from digging deeper than you need to and confusing yourself, which mostly ends up in you being overwhelmed and giving up.
Internalising the data science way of thinking about things
Data science way of thinking is knowing what to do when. It is the instinct that helps you choose your next step. It is the knowledge of how much is possible, the potential problems that might occur on the way, what needs to be done to bring together a quick proof of concept. Very simply put, it is what makes you an expert in this area.
Practising will help you expose yourself to decision points and by trial and error, you will learn to make the right decisions and your instincts will take shape in time. An efficient way of doing this is also working with a more experienced data scientist and observing their work. That’s how I got most of my data scientist senses (sort of like spidey senses) when I was just starting out. And that’s why I designed my course Master the Data Science Method in the form of a project where I guide you on your practical work and you get to follow along with me as I go through the data science pipeline.
Finding out the best practices
Although you can learn about best practices during your theoretical training, you cannot possibly understand and internalise all of it through passive learning. It’s easy to think when A happens I need to do X. But when you’re working on a project will it be easy to spot that A happened? Will you need to do extra checks to see if A happened? If you do, then when should you do those checks exactly?
It is not easy to write down the answers to these questions individually. But when you practice, it will be much easier to understand the whys and hows behind the best practices.
You will mess up. Then you will Google it and read some online threads. And then you will see someone mention that best practice or rule of thumb you heard before. You will have a lightbulb moment. “That’s why it is a best practice!” you will think. And, likely, you will not forget this again.
Long story short, practising your skills can only do you good. Once you start working on your own projects, you will be surprised at how much you learn during the process. Before you do it, it’s sort of a “you don’t know what you don’t know” situation. Take a chance, start a side project and trust the process!
Recommend
About Joyk
Aggregate valuable and interesting links.
Joyk means Joy of geeK