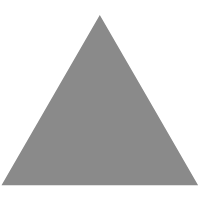
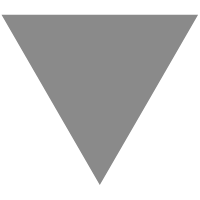
Logistic Modeling & Maximum Likelihood Estimation vs. Linear Regression &...
source link: http://econometricsense.blogspot.com/2010/09/logistic-modeling-maximum-likelihood.html
Go to the source link to view the article. You can view the picture content, updated content and better typesetting reading experience. If the link is broken, please click the button below to view the snapshot at that time.
Logistic Modeling & Maximum Likelihood Estimation vs. Linear Regression & Ordinary Least Squares
See also: Analysis of the Logistic Function
Ordinary Least Squares:
OLS: y = XB + e
Minimizes the sum of squared residuals e'e where e = (y –XB)
R2 = 1- SSE/SST
OLS With a Dichotomous Dependent Variable: y = (0 or 1)
Dichotomous Variables, Expected Value, & Probability:
Linear Regression E[y|X] = XB ‘conditional mean(y) given x‘
If y = { 0 or 1}
E[y|X] = Pi a probability interpretation
Expected Value: sum of products of each possible value a variable can take * the probability of that value occurring.
If P(y=1) = pi and P(y=0) = (1-pi) then E[y] = 1*pi +0* (1-pi) = pi → the probability that y=1
Problems with OLS:
1) Estimated probabilities outside (0,1)
2) e~binomial var(e) = n*p*(1-p) violates assumption of uniform variance → unreliable inferences
Logit Model:
Ln ((Di /(1 – Di)) = βX
Di = probability y = 1 = eXβ / ( 1 + eXβ )
Where : Di / (1 – Di) = ’odds’
E[y|X] = Prob(y = 1|X) = p = eXβ / (1 + eXβ )
Maximum Likelihood Estimation
L(θ) =∏f(y,θ) -the product of marginal densities
Take ln of both sides, choose θ to maximize, → θ* (MLE)
Choose θ’s to maximize the likelihood of the sample being observed.Maximizes the likelihood that data comes from a ‘real world’ characterized by one set of θ’s vs another.
Estimating a Logit Model Using Maximum Likelihood:
L(β) = ∏f(y, β) = ∏ eXβ / (1 + eXβ ) ∏ 1/(1 + eXβ)
choose β to maximize the ln(L(β)) to get the MLE estimator β*
To get p(y=1) apply the formula Prob( y = 1|X) = p = eXβ* / (1 + eXβ*) utilizing the MLE estimator β*to'score' the data X.
Deriving Odds Ratios:
Exponentiation ( eβ ) gives the odds ratio.
Variance:
When we undertake MLE we typically maximize the log of the likelihood function as follows:
Max Log(L(β)) or LL ‘log likelihood’ or solve:
∂Log(L(β))/∂β = 0 'score matrix' = u( β)
-∂u(β)/ ∂β 'information matrix' = I(β)
Assessing Model Fit and Predictive Ability:
Not minimizing sums of squares: R2 = 1 – SSE / SST or SSR/SST. With MLE no sums of squares are produced and no direct measure of R2 is possible. Other measures must be used to assess model performance:
Model χ2 : DN -DK For a good fitting model, model deviance will be smaller than null deviance, giving a larger χ2 and a higher level of significance.
Pseudo-r-square: DN -DK / DN Smaller (better fitting) DK gives a larger ratio. Not on (0,1)
Cox & Snell Pseudo R square: adjusts for parameters and sample size, not on (0,1)
Nagelkerke (Max-rescaled r-square) : transformation such that R --> (0,1)
Other:
Percentage of Correct Predictions
Area under the ROC curve:
Area = measure of model’s ability to correctly distinguish cases where (y=1) from those that do not based on explanatory variables.
y-axis: sensitivity or prediction that y = 1 when y = 1,
x-axis: 1-specificity or prediction that y = 1 when y = 0, false positive
References:
Menard, Applied Logistic Regression Analysis, 2nd Edition 2002
Recommend
About Joyk
Aggregate valuable and interesting links.
Joyk means Joy of geeK