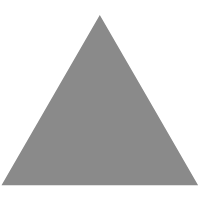
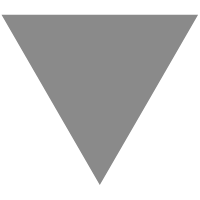
Predicting structures and stabilities for H-type pseudoknots with interhelix loo...
source link: https://www.ncbi.nlm.nih.gov/pmc/articles/PMC2661829/
Go to the source link to view the article. You can view the picture content, updated content and better typesetting reading experience. If the link is broken, please click the button below to view the snapshot at that time.
Predicting structures and stabilities for H-type pseudoknots with interhelix loops
Abstract
RNA pseudoknots play a critical role in RNA-related biology from the assembly of ribosome to the regulation of viral gene expression. A predictive model for pseudoknot structure and stability is essential for understanding and designing RNA structure and function. A previous statistical mechanical theory allows us to treat canonical H-type RNA pseudoknots that contain no intervening loop between the helices (see S. Cao and S.J. Chen [2006] in Nucleic Acids Research, Vol. 34; pp. 2634–2652). Biologically significant RNA pseudoknots often contain interhelix loops. Predicting the structure and stability for such more-general pseudoknots remains an unsolved problem. In the present study, we develop a predictive model for pseudoknots with interhelix loops. The model gives conformational entropy, stability, and the free-energy landscape from RNA sequences. The main features of this new model are the computation of the conformational entropy and folding free-energy base on the complete conformational ensemble and rigorous treatment for the excluded volume effects. Extensive tests for the structural predictions show overall good accuracy with average sensitivity and specificity equal to 0.91 and 0.91, respectively. The theory developed here may be a solid starting point for first-principles modeling of more complex, larger RNAs.
INTRODUCTION
An RNA pseudoknot is formed when nucleotides in a loop base-pair with complementary nucleotides outside the loop. An H-type pseudoknot is formed by base-pairing between a hairpin loop and the single-stranded region of the hairpin. The structure consists of two helix stems (Fig. 1A, S1, S2) and two loops (Fig. 1A, L1, L2) as well as a possible third loop/junction (Fig. 1A, L3) that connects the two helix stems. In most naturally occurring RNA pseudoknots, interhelix loop L3 contains no more than 1 nucleotide (nt). For these canonical pseudoknot structures, helix stems S1 and S2 tend to stack coaxially (or partially coaxially) to form a quasicontinuous RNA helix in the three-dimensional space (3D) (Walter and Turner 1994; Chen et al. 1996; Cornish et al. 2005; Theimer et al. 2005). The coaxial stacking interaction can provide an essential stabilizing force for the structure.
(A) An RNA pseudoknot with an interhelix loop L3. (B) Traditional two-vector virtual bond model for RNA nucleotides involves two bonds, P–C4–P. To describe the base orientation, we introduce a third virtual bond model, C4–N1 (pyrimidine) or C4–N9 (purine). (C) A virtual bond representation for a pseudoknot motif with S1 = 8 bp, S2 = 6 bp, L1 = 4 nt, L2 = 4 nt, and L3 = 2 nt.
The pseudoknot is a widespread motif in RNA structures (van Belkum et al. 1985; Perrotta and Been 1991; Tanner et al. 1994; Deiman et al. 1997; Ferré-D'Amaré et al. 1998; Su et al. 1999; Schultes and Bartel 2000) and plays a variety of structural and functional roles in RNAs. For instance, pseudoknots form the core structural motif in the central catalytic domain of human telomerase RNA (Chen et al. 2000; Comolli et al. 2002; Theimer et al. 2005). As another example, for many viruses, pseudoknots play indispensable roles in promoting ribosomal frameshifting, a mechanism used by a retrovirus to regulate retroviral genome expression (Brierley et al. 1989, 2007; Somogyi et al. 1993; Giedroc et al. 2000; Plant et al. 2003; Staple and Butcher 2005; Namy et al. 2006; Hansen et al. 2007; Cao and Chen 2008; Pennell et al. 2008). Mutations that strengthen or weaken pseudoknot (thermal or mechanical) stability can cause changes in ribosomal frameshifting efficiency (Cornish et al. 2005; Theimer et al. 2005). For these, and a vast number of other RNA-related problems, quantitative prediction of pseudoknot structure and its stability is essential in order to unveil the mechanisms of RNA functions and in order to design therapeutic strategies for the diseases. In the present study, we develop a rigorous statistical mechanical model to predict the structure and folding stability for general RNA pseudoknots.
There are two main approaches used to predict RNA structures: free-energy minimization and comparative sequence analysis. Existing free-energy-based algorithms are mainly for the prediction of the secondary structures. For instance, Nussinov and coworkers developed a dynamical programming algorithm for the prediction of the minimum free-energy secondary structure (Nussinov et al. 1978; Nussinov and Jacobson 1980). Later, Williams and Tinoco (1986) extended the dynamical programming algorithm to find multiple low free-energy structures. In 1989, Zuker (1989) developed an advanced algorithm to predict all suboptimal low free-energy structures, and the algorithm led to the widely used Mfold software. In 1999, Mathews et al. (1999) developed an algorithm based on the much improved thermodynamic parameters. Algorithms based on the statistical mechanical partition function provide an alternative approach to predicting the structure and structural distributions (McCaskill 1990; Chen and Dill 2000; Hofacker 2003).
For RNA pseudoknots, several lines of computational algorithms have also been developed (Gultyaev et al. 1995; Rivas and Eddy 1999; Dirks and Pierce 2003; Reeder and Giegerich 2004; Ruan et al. 2004; Ren et al. 2005; Huang and Ali 2007; Chen et al. 2008; Metzler and Nebel 2008; Sperschneider and Datta 2008). Heuristic approaches (Ren et al. 2005) are computationally efficient, but unlike dynamic algorithms, they cannot guarantee finding the global free-energy minimum. Critical to an accurate structure prediction are the energy and entropy parameters. Current pseudoknot structural prediction algorithms often ignore the contribution of loop entropies (Ren et al. 2005) or use simplified (nonphysical) approximations (Dirks and Pierce 2003) for the loops. Although these models are remarkable in their computational efficiency to treat large RNA pseudoknots with hundreds of nucleotides (Mathews and Turner 2006; Reeder et al. 2006; Schuster 2006; Jossinet et al. 2007; Shapiro et al. 2007; Bon et al. 2008), their accuracies are limited by the availability of reliable parameters for the entropies of pseudoknotted loops. A set of rigorous entropy parameters, such as the one derived in the present study, would be highly desirable for reliable structure prediction (Cao and Chen 2006b). Indeed, a current emphasis for RNA pseudoknot prediction is how to include the thermodynamic parameters, especially the loop entropy, in the dynamic algorithms (Zhang and Chen 2001; Ding 2006; Kopeikin and Chen 2006; Chen 2008; Chu and Herschlag 2008; Jabbari et al. 2008; Li et al. 2008; Zhang et al. 2008).
Using a virtual-bond-based RNA conformational model (termed the “Vfold” model) (Cao and Chen 2005, 2006a), we recently developed a physics-based theory to calculate the loop entropy and free energy for simple canonical H-type pseudoknots (Cao and Chen 2006b), namely, pseudoknots with no interhelix loop (Fig. 1A, L3). For such canonical H-type pseudoknots, the two helix stems often form a quasicontinuous coaxially stacked helix. Central to the loop entropy calculation is the influence of the excluded volume between loop and helix. The effect of volume exclusion is sensitive to the stem and loop lengths. Here, we develop a new Vfold model to treat more complex pseudoknots that contain an interhelix loop (Fig. 1A, L3). The development of such a more-general model is significant for two reasons. First, the general pseudoknots studied here form the structural basis for large RNA folds, which involve multiple loops between helices. Second, the interhelix loops considered here are biologically important. For example, it has been suggested that a large class of anti-HIV RNA aptamers form pseudoknots with interhelix loops (Burke et al. 1996) so that the aptamers can be flexible and prevent the rigid coaxial stacking between the helices.
This paper is organized as follows. We first present a new three-vector virtual-bond-based RNA conformational model. The development of the new virtual bond model is motivated by the need to explicitly include the base orientations in addition to the backbone configuration considered in the original Vfold model (Cao and Chen 2005). We then use the new conformational model to compute the loop entropies in different pseudoknot contexts. A key issue in the calculation is how to account for the excluded volume effects. The entropy parameters will then allow us to predict the lowest free-energy structure as well as the folding thermodynamics from the RNA sequence. Comparisons with other models for structural prediction show improved results from our new model. As an application of the theory, we also investigate the equilibrium unfolding pathway for an anti-HIV RNA pseudoknot aptamer (Burke et al. 1996), the Visna-Maedi virus (VMV) pseudoknot (Pennell et al. 2008), and the 5′ coding region of the R2 retrotransposon (Hart et al. 2008). The anti-HIV and VMV pseudoknots contain 3-nt and 6-nt interhelix loop L3, respectively.
STRUCTURAL MODEL
A three-vector virtual bond model
Because the torsional angles of the C–O bonds (Fig. 1B, ε, β) in the nucleotide backbone tend to adopt the trans isomeric state, Olson (Olson and Flory 1972; Olson 1980) proposed to use a two-vector virtual bond to represent nucleic structures (see Fig. 1A). We recently developed a virtual-bond-based RNA folding model (the Vfold model) for H-type RNA pseudoknots (Cao and Chen 2006b). In Vfold, we model the helix as an A-form RNA helix using the experimentally measured atomic coordinates. For loops, which can be flexible, we use the usual gauche + (g +), trans (t), and gauche −1 (g −1) rotational isomeric states for polymers (Flory 1969) to sample backbone conformations. The fact that the three isomeric states can be exactly configured in a diamond lattice (Cao and Chen 2005, 2006b) suggests that we can effectively configure the loop conformations as random walks of the virtual bonds on a diamond lattice. We note that the rotameric nature of RNA backbone conformations also has been observed for the known RNA structures (Duarte and Pyle 1998; Murthy et al. 1999; Murray et al. 2003; Wadley et al. 2007; Richardson et al. 2008).
The traditional two-vector virtual bond model cannot describe the base orientations. Motivated by the need to explicitly include base orientations in the structural description, we here propose a three-vector virtual bond model by introducing a third virtual bond to describe the base orientation (see Fig. 1B). Specifically, we add the N1 (for pyrimidine) or N9 (for purine) atom to the original P–C4 and C4–P virtual bonds (Fig. 1B). From the PDB database (Michiels et al. 2001; Theimer et al. 2005) for RNA pseudoknots, we find that the distance (D CN) between N1 (N9) and C4 atoms is close to 3.9 Å. In addition, we find that the torsion angle (χ) between plane Pi–C4–Pi +1 and plane Pi–C4–N1 (N9) is close to the g −1 isomeric state; see the distributions for D CN and the torsion angle in Figure 2. The localized distributions for the virtual bond C4–N1 (N9) in Figure 2 suggest that C4–N1 (N9) is quite rigid and can be configured in a diamond lattice. A previous study on RNA molecules also suggested a rigid base orientation (Olson and Flory 1972).
Survey of the (DCN, χ) distributions for two pseudoknot structures: (A) the 47-nt ΔU177 pseudoknot (Theimer et al. 2005) (PDB code: 1YMO) and (B) the 36-nt SRV-1 pseudoknot (Michiels et al. 2001) (PDB code: 1E95). DCN is the length of the C4–N1,9 virtual bond, and χ is the torsion angle between the Pi–C4–Pi +1 plane and the Pi–C4–N1,9 plane.
Figure 1A shows a pseudoknot with an interhelix loop. We use the atomic coordinates of the A-form RNA helix to configure the helices (Arnott and Hukins 1972). The (r, θ, z) coordinates (in a cylindrical coordinate system) for the P, C4, and N1 (or N9) atoms are (8.71 Å, 70.5 + 32.7i, −3.75 + 2.81i), (9.68 Å, 46.9 + 32.7i, −3.10 + 2.81i), and (7.12 Å, 37.2 + 32.7i, −1.39 + 2.81i) (i = 0, 1, 2, …) (Arnott and Hukins 1972), respectively. For the other strand, we need to negate θ and z.
We generate loop (L1, L2, or L3) conformations through self-avoiding walks in a diamond lattice (Cao and Chen 2005), where a virtual bond is represented by a lattice bond. In the Vfold model, helices are configured off-lattice, while loop conformations are on-lattice. Loops and helices are connected via the six loop–helix interfacial nucleotides (Fig. 1A,C, a1, a2, b1, b2, c1, c2). These six interfacial nucleotides can be configured either as on-lattice loop terminals or as off-lattice helix terminals. We connect the loop and the helix through the minimum RMSD between the on-lattice and off-lattice coordinates for the six terminal nucleotides. Our calculation shows that the minimum RMSD is as small as 0.56 Å for the (Pi, C4, N1 or N9, Pi +1) virtual bond atoms. The small RMSD indicates a smooth connection/transition between the on-lattice loop and the off-lattice helix.
CONFORMATIONAL ENTROPY FOR PSEUDOKNOT WITH AN INTERHELIX LOOP
For a given pseudoknot defined by the stem lengths (S1, S2) and the loop lengths (L1, L2, L3), we enumerate all the possible (virtual bond) conformations in the 3D space. From the total number of the viable conformations Ω, we calculate the conformational entropy of the given pseudoknot as ΔS(S1, S2, L1, L2, L3) = k B ln Ω, where k B = 1.99 cal/K is the Boltzmann constant. We choose different (S1, S2, L1, L2, L3) values (i.e., different pseudoknots), compute the conformational entropy for each pseudoknot, and compile the results as a large table for pseudoknot conformational entropy parameters.
Compared to simple canonical H-pseudoknots with no interhelix junction (junction-free pseudoknots), the pseudoknots here are much more complicated because the interhelix loop (Fig. 1, L3) between the two stems may be flexible, causing variable relative orientations between the helices. The previous model for the junction-free pseudoknots is a special case for the model developed here. To compute the total conformational count Ω for a pseudoknot with an interhelix loop, we enumerate the possible orientations between the two helices and then enumerate the loop conformations for each helix orientation:

where ΩPK is the number of conformations for a given helix orientation.
Enumeration of helix orientations
The orientations of helices S1 and S2 are determined by the coordinates of the terminal nucleotides (Fig. 1A, c1, c2) of the loop L3. To enumerate the relative orientations of the helices, we fix the (Pi, C4, N1 or N9, Pi +1) coordinates for c1, then enumerate the viable (Pj, C4, N1 or N9, Pj +1) coordinates for c2. Specifically, we enumerate the loop L3 conformations as self-avoiding random walks of the virtual bonds in a diamond lattice. The number of possible coordinates of the terminal nucleotide c2 (specifically, the coordinates of Pj, C4, N1, or N9, and Pj +1 atoms of nucleotide c2) is much smaller than the number of loop L3 conformations (see Fig. 4A, below). Therefore, the number of helix orientations, as determined by the c1 and c2 positions/coordinates, increases with L3 much more slowly than the number of loop (L3) conformations. For instance, the number of helix orientations grows as 73 → 390 → 1358 → 3208 → 6096 → 10,272 → 15,984 for an increasing interhelix loop length 1 → 2 → 3 → 4 → 5 → 6 → 7 nt. The slow growth of the number of helix orientations makes the exact enumeration of all the possible helix orientations computationally viable.
(A, filled triangle) The number of single-stranded RNA chain conformations grows much faster than (unfilled triangle) the number of the end–end configurations (relative coordinates) of the chain. (B) We study the excluded volume effects on the loop entropy for a given pseudoknot with S1 = 4 bp, S2 = 4 bp, L1 = 4 nt, and L3 = 2 nt. We vary the loop length for L2 from 1 nt to 7 nt and find that we can neglect the loop–loop excluded volume interactions. (Open square) Results without excluded volume interactions; (filled triangle) results without considering the loop–loop excluded volume interactions; (open triangle) results with all the excluded volume interactions fully considered. (C) Comparison between the conformational entropy from the exact computer enumeration and the entropy from our theory. The deviation is small (<1 k BT in free energy).
Enumeration of loop conformations for a given helix orientation
For each relative orientation of the helices S1 and S2, we compute ΩPK in Equation 1 by enumerating the conformations for loops L1 and L2 and loop L3. The key is how to treat the excluded volume effect, i.e., the effect that different atoms cannot bump into each other.
We have two choices to treat the excluded volume effect here. We may fit the off-lattice helix onto the diamond lattice, then both helix and loop are configured in the same lattice, thus the volume exclusion effect can be conveniently treated in the lattice framework. Such an approach is computationally time-consuming because it requires off-lattice → on-lattice fitting for all the helices for each and every helix orientation. Given the large number of helix orientations that we enumerate (Equation 1), the excluded volume treatment based on the above lattice-fitting is highly inefficient.
Alternatively, we can take a different approach that avoids the off-lattice → on-lattice fitting procedure. The strategy of the alternative approach is to keep the off-lattice helix structure. To treat the mixed system with the on-lattice loop conformations and off-lattice helix structure, we introduce a cutoff distance Dc such that atoms separated by a distance below the cutoff are considered to bump into each other, and the corresponding conformation is eliminated. Such a cutoff would allow us to treat the excluded volume effect in a unified framework, irrespective of the on-lattice or off-lattice representation of the conformations. We determine the value of the cutoff distance Dc from the criterion that it gives the same entropy as the one calculated from the off-lattice → on-lattice fitting. We found that the optimal Dc value is 2.8 Å (see Fig. 3C,D). Therefore, in our entropy computation, we use Dc = 2.8 Å as the criterion for volume exclusion.
Test of the excluded volume effects with different distance cutoffs (Dc). (A) Using a pseudoknot motif as the test system, we find that the cutoff distance of 2.8 Å can best reproduce the conformational count from exact enumeration of on-lattice conformations. (B) The triangles are the calculated entropies with cutoff distance varying from 2.2 Å to 3.4 Å. The line denotes the entropies from the on-lattice exact computer enumeration. (C) We also test the cutoff distances for different stem lengths while keeping the loop lengths fixed and (D) for different loop lengths while keeping the stem length fixed. (Dashed lines) The results with off-lattice helix and the cutoff distance; (solid lines) the results with on-lattice exact conformational enumerations. (B–D) The y-axes are the numbers of loop (L2) conformations (in log scale).
For a fixed helix–helix orientation, we enumerate the loop conformations through self-avoiding random walks in the diamond lattice. The excluded volume between helix and helix, helix and loop, and loop and loop are explicitly considered. The treatment here for the excluded volume effect is more rigorous than previous Gaussian chain-based models (Gultyaev et al. 1999; Isambert and Siggia 2000; Bon et al. 2008), which ignore the excluded volume effect.
Using the three-vector virtual bond conformational model developed here, we can test the strengths of the different excluded volume effects (helix–helix, helix–loop, and loop–loop) (see Fig. 4B). We find that the loop–helix excluded volume interaction is strong. In contrast, the loop–loop excluded volume effect is rather weak (Fig. 4B), suggesting that we can neglect loop–loop correlations when enumerating the conformations. This leads to the “independent loop approximation”: we can ignore the presence of the other loops when we count the conformations for a loop.
Tests for the above method against exact computer enumeration suggest that our method can give reliable conformational entropies (see Fig. 4C). We have computed and tabulated the loop entropies ΔS(S1, S2, L1, L2, L3) for stems S1 and S2 as ≤10 bp; loops L1, L2 as ≤7 nt; and loop L3 as between 2 nt and 6 nt. For L3 ≤ 1 nt, we use the entropy table in Cao and Chen (2006b). The results for ΔS(S1, S2, L1, L2, L3) are compiled as an entropy table (see Supplemental Table S1). On a Dell EM64T cluster system with 48 Intel(R) Xeon(R) 5150 (2.66 GHz) processors, the computation of the full entropy table took less than a week.
For large loops L1 > 7 nt or L2 > 7 nt, we use the following fitted formula (Serra and Turner 1995) for the entropy ΔS:

where l is the loop length and a and b are fitted parameters; see Supplemental Tables S2 and S3 for the a and b parameters for different loop sizes.
PARTITION FUNCTION
In this section, we show how to use the recursive algorithm (Cao and Chen 2005, 2006b) to compute the partition function, from which all the thermodynamic properties of the system can be determined. Our partition function calculation is a sum over all the possible secondary and pseudoknotted structures, with and without an interhelix loop. A typical “prototype” structure contains internal/bulge loops in the helix stems (see Fig. 5). Our conformational ensemble also includes other structures that stem from the prototype structure. For example, for the pseudoknot in Figure 5, we enumerate different L1 and L2 loop structures by allowing the formation of possible secondary structures in loops L1 and L2. We also allow multidomained structures, where each domain is an independently folded pseudoknotted or secondary structure.
A general pseudoknotted structural element considered in the partition function calculation.
We denote the partition function for the ensemble of all the possible pseudoknotted structures by C3(a, b), where a and b denote the 5′ and 3′ terminal nucleotides, respectively, and the subscript 3 denotes a pseudoknot (tertiary) structure. A general pseudoknotted structure shown in Figure 5 is described by 11 parameters: the lengths of loops (L1, L2, L3), the lengths of upper and lower segments of the helices (n11, n12, n21, n22), and the lengths of the internal loops (l 11, l 12, l 21, l 22). Exhaustive enumeration of all the possibilities for these 11 parameters for an n-nt chain requires a computational time scale on the order of n11, which would be computationally unfeasible for long pseudoknot sequences.
If the internal/bulge loops in the helix stems S1 and S2 are flexible, S1 and S2 would be floppy, causing complications in the calculations of the loop (L1 and L2) entropies. In order to make use of our entropy parameter table, which assumes rigid helix stems (Fig. 1A), we employ an approximation for the stems, as described below. The (internal/bulge) loop tends to leave or enter a helix in a right-handed helical way, resulting in rigid conformations for a short internal/bulge loop. Therefore, for the purpose of the loop entropy calculation, we treat an internal/bulge loop as an effective helix of length S eff1 (S eff2) as determined by the following equations (see Fig. 5):

In this way, we can read the entropy directly from the entropy table ΔS(S1, S2, L1, L2, L3) with S1 and S2 substituted by the effective helix lengths S eff1 and S eff2 for stems S1 and S2, respectively. For pseudoknots without internal/bulge loops in the stems, S eff1 and S eff2 are equal to the lengths of the original helices. An internal/bulge loop often causes bending of the stem (S1 or S2). Through the above approximation, we replace a bent stem with a continuous helix for the purpose of loop entropy calculation. Our control tests show that the approximation causes minor errors: ≤5% and 15% in the entropy results with helix stems containing bulge loops of length ≤2 nt and 3 nt, respectively (see details in the Supplemental Material).
For a loop (L1 or L2) with nested helices (see Fig. 8A, below), we neglect the excluded volume effect from the nested helices and calculate the effective loop length as the number of helices plus the unpaired nucleotides outside the helices.
The density plots and the predicted structures for the 74-nt 5′ header of an R2 retrotransposon at different temperatures. Stem S3 is the most stable stem, which is the last one to be unzipped. At room temperature, the predicted structure shows the highest SE = 1.0 and SP = 1.0 as tested against the experimental NMR structure (Hart et al. 2008).
We separate out pseudoknot-containing structures from pseudoknot-free structures (secondary structures) in the partition function calculation. We compute the partition function C3(a, b) for pseudoknot-containing structures from nucleotide a at the 5′ end to nucleotide b at the 3′-end by enumerating all the possible values of helix stem lengths S eff1 and S eff2 and loop lengths L1, L2, and L3:

where ΔG(S eff1, S eff2, L1, L2, L3) is the free energy for a given structure:

We read out ΔS(S eff1, S eff2, L1, L2, L3) from the entropy table. ΔGstem(S eff1) and ΔGstem(S eff2) are the folding free energy of the respective stems. ΔGstem(S eff) for a stem (S1 or S2) is computed from the local partition function for the stem:

Here in the sum for stems with a given S eff, we consider the presence and absence of an internal or bulge loop and the different sizes and positions of the loop. The free energy of the stem ΔGstem in the above equation is the sum of the free energies for the base stacks and the loop in the stem, as determined by the nearest-neighbor model (Serra and Turner 1995; Cao and Chen 2005).
With the internal loops replaced by the effective helices in the loop entropy calculation, the conformational entropy for a general structure shown in Figure 5 is only dependent on five (instead of 11) parameters: S eff1, S eff2, L1, L2, and L3. As shown in Equation 3, the computation for the partition function is now much more efficient, and the computational time scales as n5 instead of n11 for an n-nt chain.
Using the recursive algorithm in Cao and Chen (2006b), we compute the total partition function Q(a, b) for a chain from a to b. From the conditional partition function Qij for all the conformations that contain base pair (i, j) between nucleotides i and j, we compute the base-pairing probability Pij:

Here Q tot is the total partition function for all the possible structures. From Pij for all the possible (i, j)’s, we can predict the stable structures and the equilibrium folding pathways.
STRUCTURAL PREDICTIONS
Comparison with other models
We measure the accuracy of structure predictions by two parameters: (1) the sensitivity parameter SE, defined as the ratio between the number of correctly predicted base pairs and number of the base pairs in the experimentally determined structure; and (2) the specificity parameter SP, defined as the ratio between the number of correctly predicted base pairs and the total number of predicted base pairs. Our tests for structural predictions indicate that the model developed here gives better results than other models that we have tested (see Tables 1, ,2).2). Specifically, our model gives the highest SE value for 15 sequences among the total 18 sequences, and the highest SP value for 13 sequences. In addition, our model gives higher overall average SE (0.91) and SP (0.91) than other models.
H-type pseudoknot
LP-PK1 and SRV-1 are two H-type pseudoknots. LP-PK1 is a PK1 domain of Legionella pneumophila tmRNA (Zwieb et al. 1999), and Simian retrovirus type-1 (SRV-1) (ten Dam et al. 1995) forms a pseudoknot that promotes the ribosomal frameshifting. For the two H-type pseudoknots, our Vfold model gives the highest SE value (see Fig. 6A,B; Table 1). We note that the ILM model gives a false prediction for the SRV-1 pseudoknot. The failure of the ILM model may be due to the fact that the model does not account for the loop entropy for pseudoknots.
The predicted structures for three pseudoknots. (A) For LP-PK1, Hotknots (Ren et al. 2005), ILM (Ruan et al. 2004), pknotsRE (Rivas and Eddy 1999), STAR (Gultyaev et al. 1995), and pknots-RG (Reeder and Giegerich 2004) all give poor predictions for the structure (SE = 0.5). NUPACK (Dirks and Pierce 2003) gives a relatively high SE value (SE = 0.8). Our Vfold model gives the highest accuracy with SE = 0.9. (B) For the SRV-1 pseudoknot, the ILM model fails to predict the native structure of the SRV-1 pseudoknot. (C) For the 70.8 anti-HIV aptamer, we predict a pseudoknot with a 3-nt interhelix loop. In the calculations, the temperature is 37°C for A and B and 20°C for C according to the experimental condition (Held et al. 2006a,b). Also shown in the figures are the density plot for the base-pairing probability Pij (Equation 4). In the density plots, the horizontal and vertical axes denote the indices of the nucleotides i and j.
VMV pseudoknot
From a recent biochemical study, Pennell and Brierley and colleagues found that the stimulatory RNA for VMV frameshifting forms a pseudoknot structure (Pennell et al. 2008) instead of a stem–loop structure. Moreover, the pseudoknot is quite unique because it contains a long interhelix loop. We perform the structural prediction for this 67-nt RNA. Figure 7A shows that the predicted structure agrees exactly with the experimental structure, with SE and SP values both equal to 1.
The density plots and the predicted structures for the 67-nt 5′ VMV pseudoknot at different temperatures. Stem S1 is the most stable stem, which is the last one to be unzipped. At T = 37°C, our predicted structure shows the highest SE = 1.0 and SP = 1.0 as tested against the experimental structure (Pennell et al. 2008).
R2 retrotransposon pseudoknot
The 5′ header of the R2 retrotransposon controls the R2 protein binding and cleavage of the DNA target (Christensen et al. 2006). Based on the NMR spectra and computational models (Hart et al. 2008), Hart and Turner and colleagues found a knotted structure in the 74-nt header of the R2 retrotransposon. In this study, we use the Vfold model developed here to predict the secondary structure for the 74-nt header. Figure 8A shows our predicted structure. The predicted structure is a pseudoknot with four stems. All four stems have been found in the experiments (Hart et al. 2008). The predicted structure shows a high accuracy with SE = 1.0 and SP = 1.0. In the calculation, we have added the base-stacking energy for the Watson-Crick base pairs between nucleotides 48CG49 and 62CG63 (see the dashed lines in Fig. 8A). This tertiary interaction has been confirmed in previous NMR measurement (Ferré-D'Amaré et al. 1998).
Anti-HIV RNA aptamer
Recently experiments suggested that the interhelix loop may be essential for efficient ribosomal frameshifting (Brierley et al. 2008; Giedroc and Cornish 2008). Moreover, previous experimental studies on the anti-HIV RNA aptamer (Held et al. 2006a,b) suggested that the interhelix loop, which causes flexible helix orientations in the pseudoknot aptamers, may play an important functional role in accommodating aptamer binding to the HIV reverse transcriptase. For example, for an aptamer (labeled as 70.8 according to the notations used in the literature) (Held et al. 2006a,b), the proposed native structure contains a 3-nt interhelix loop. The predicted structure from our model (Fig. 6C), indeed, shows a 3-nt interhelix loop. The structure has a high accuracy of SE = 0.9 and SP = 1.0 if we treat the experimentally proposed structure (Held et al. 2006a,b) as the “experimental” structure.
FOLDING THERMODYNAMICS
The 70.8 aptamer
As the temperature increases, stems S1 and S2 of the pseudoknot (see Fig. 6A) is disrupted at nearly the same temperature. At T = 80°C, both stems are unfolded. Thus, stems S1 and S2 have the comparable thermal stability.
VMV pseudoknot
A recent combined biochemical and NMR experiment (Pennell et al. 2008) showed that the VMV pseudoknot contains a 6-nt interhelix loop. Our predicted unfolding pathway suggests that at T = 80°C, stem S2 is the first stem to be unzipped, and stem S1 is the last one to be unzipped (see Fig. 7B,C). Our prediction agrees with the experimental finding (Pennell et al. 2008), which suggested that S1 is the most stable and S2 is disrupted at a temperature around 76.8°C.
R2 retrotransposon pseudoknot
The native structure of the R2 retrotransposon pseudoknot contains four stems (Fig. 8A). The structure shows high stability against temperature increase. At T = 80°C, stem S1 is the first stem to be unfolded, resulting in an intermediate state that contains stem S3 and a partially unfolded stem S2. As the temperature is further increased to 90°C, stem S2 becomes completely unzipped since the hairpin with S2 is destabilized by the large loop. Stem S3 is the most robust stem and is the last stem to be unzipped. The melting temperature for S3 is ∼100°C.
SUMMARY
In summary, we have developed a new virtual-bond-based model (Vfold) for general RNA pseudoknots with interhelix loops. The model allows an accurate treatment for the loop–helix excluded volume interactions and rigorous calculations for the conformational entropy for general pseudoknotted folds. Tests against other existing models suggest that this new model gives improved predictions for the native structures, with average sensitivity and specificity measures of the accuracy equal to 0.91 and 0.91, respectively. We attribute the improved accuracy to the rigorous conformational entropy parameters. For any given RNA sequence, the model enables predictions for not only the native structures, but also the folding stabilities and equilibrium folding pathways. Despite the success of this new model, it has several limitations that should be removed in future model development. First, the model does not treat possible noncanonical interactions such as base triple interactions between loops and stems and noncanonical base-pairing between loop nucleotides. These interactions can be biologically important for more complex pseudoknotted structures (Cornish et al. 2005; Theimer et al. 2005). Moreover, ions, especially Mg2+ ions, can play an important role in loop entropy and the global folding stability of pseudoknots (Chen 2008; Tan and Chen 2008).
SUPPLEMENTAL MATERIAL
Supplemental material can be found at http://www.rnajournal.org.
ACKNOWLEDGMENTS
We thank Professor Donald H. Burke for useful discussions. The research was supported by NIH through grant GM063732 (to S.-J.C.). Most of the computations involved in this research were performed on the HPC resources at the University of Missouri Bioinformatics Consortium (UMBC).
Footnotes
Article published online ahead of print. Article and publication date are at http://www.rnajournal.org/cgi/doi/10.1261/rna.1429009.
REFERENCES
- Arnott S., Hukins D.W.L. Optimized parameters for RNA double-helices. Biochem. Biophys. Res. Commun. 1972;48:1392–1399. [PubMed] [Google Scholar]
- Bon M., Vernizzi G., Orland H., Zee A. Topological classification of RNA structures. J. Mol. Biol. 2008;379:900–911. [PubMed] [Google Scholar]
- Brierley I., Digard P., Inglis S.C. Characterization of an efficient coronavirus ribosomal frameshifting signal: requirement for an RNA pseudoknot. Cell. 1989;57:537–547. [PMC free article] [PubMed] [Google Scholar]
- Brierley I., Pennell S., Gilbert R.J.C. Viral RNA pseudoknots: Versatile motifs in gene expression and replication. Nat. Rev. Microbiol. 2007;5:598–610. [PMC free article] [PubMed] [Google Scholar]
- Brierley I., Gilbert R.J.C., Pennell S. RNA pseudoknots and the regulation of protein synthesis. Biochem. Soc. Trans. 2008;36:684–689. [PubMed] [Google Scholar]
- Burke D.H., Scates L., Andrews K., Gold L. Bent pseudoknots and novel RNA inhibitors of type 1 human immunodeficiency virus (HIV-1) reverse transcriptase. J. Mol. Biol. 1996;264:650–666. [PubMed] [Google Scholar]
- Cao S., Chen S.-J. Predicting RNA folding thermodynamics with a reduced chain representation model. RNA. 2005;11:1884–1897. [PMC free article] [PubMed] [Google Scholar]
- Cao S., Chen S.-J. Free-energy landscapes of RNA/RNA complexes: With applications to snRNA complexes in spliceosomes. J. Mol. Biol. 2006a;357:292–312. [PMC free article] [PubMed] [Google Scholar]
- Cao S., Chen S.-J. Predicting RNA pseudoknot folding thermodynamics. Nucleic Acids Res. 2006b;34:2634–2652. [PMC free article] [PubMed] [Google Scholar]
- Cao S., Chen S.-J. Predicting ribosomal frameshifting efficiency. Phys. Biol. 2008;5:016002. doi: 10.1088/1478-3975/5/1/016002. [PMC free article] [PubMed] [CrossRef] [Google Scholar]
- Chen S.-J. RNA folding: Conformational statistics, folding kinetics, and ion electrostatics. Annu. Rev. Biophys. 2008;37:197–214. [PMC free article] [PubMed] [Google Scholar]
- Chen S.-J., Dill K.A. RNA folding energy landscapes. Proc. Natl. Acad. Sci. 2000;97:646–651. [PMC free article] [PubMed] [Google Scholar]
- Chen X.Y., Kang H.S., Shen L.X., Chamorro M., Varmus H.E., Tinoco I., Jr A characteristic bent conformation of RNA pseudoknots promotes −1 frameshifting during translation of retroviral RNA. J. Mol. Biol. 1996;260:479–483. [PubMed] [Google Scholar]
- Chen J.L., Blasco M.A., Greider C.W. Secondary structure of vertebrate telomerase RNA. Cell. 2000;100:503–514. [PubMed] [Google Scholar]
- Chen X., He S., Zhang F., Wang Z., Chen R., Gao W. FlexStem: Improving predictions of RNA secondary structures with pseudoknots by reducing the search space. Bioinformatics. 2008;24:1994–2001. [PubMed] [Google Scholar]
- Chu V.B., Herschlag D. Unwinding RNA's secrets: Advances in the biology, physics, and modeling of complex RNAs. Curr. Opin. Struct. Biol. 2008;18:305–314. [PMC free article] [PubMed] [Google Scholar]
- Comolli L.R., Smirnov I., Xu L., Blackburn E.H., James T.L. A molecular switch underlies a human telomerase disease. Proc. Natl. Acad. Sci. 2002;99:16998–17003. [PMC free article] [PubMed] [Google Scholar]
- Cornish P.V., Hennig M., Giedroc D.P. A loop 2 cytidine-stem 1 minor groove interaction as a positive determinant for pseudoknot-stimulated −1 ribosomal frameshifting. Proc. Natl. Acad. Sci. 2005;102:12694–12699. [PMC free article] [PubMed] [Google Scholar]
- Christensen S.M., Ye J.Q., Eickbush T.H. RNA from the 5′ end of the R2 retrotransposon controls R2 protein binding to and cleavage of its DNA target site. Proc. Natl. Acad. Sci. 2006;103:17602–17607. [PMC free article] [PubMed] [Google Scholar]
- Deiman B.A., Kortlever R.M., Pleij C.W. The role of the pseudoknot at the 3′end of turnip yellow mosaic virus RNA in minus-strand synthesis by the viral RNA-dependent RNA polymerase. J. Virol. 1997;71:5990–5996. [PMC free article] [PubMed] [Google Scholar]
- Ding Y. Statistical and Bayesian approaches to RNA secondary structure prediction. RNA. 2006;12:323–331. [PMC free article] [PubMed] [Google Scholar]
- Dirks R.M., Pierce N.A. A partition function algorithm for nucleic acid secondary structure including pseudoknots. J. Comput. Chem. 2003;24:1664–1677. [PubMed] [Google Scholar]
- Duarte C.M., Pyle A.M. Stepping through an RNA structure: A novel approach to conformational analysis. J. Mol. Biol. 1998;284:1465–1478. [PubMed] [Google Scholar]
- Ferré-D'Amaré A.R., Zhou K.H., Doudna J.A. Crystal structure of a hepatitis delta virus ribozyme. Nature. 1998;395:567–574. [PubMed] [Google Scholar]
- Flory P.J. Statistical mechanics of chain molecules. Wiley, New York: 1969. [Google Scholar]
- Giedroc D.P., Cornish P.V. Frameshifting RNA pseudoknots: Structure and mechanism. Virus Res. 2008 doi: 10.1016/j.virusres.2008.06.008. (in press) [PMC free article] [PubMed] [CrossRef] [Google Scholar]
- Giedroc D.P., Theimer C.A., Nixon P.L. Structure, stability and function of RNA pseudoknots involved in stimulating ribosomal frameshifting. J. Mol. Biol. 2000;298:167–185. [PMC free article] [PubMed] [Google Scholar]
- Gultyaev A.P., van Batenburg F.H.D., Pleij C.W.A. The computer simulation of RNA folding pathways using a genetic algorithm. J. Mol. Biol. 1995;250:37–51. [PubMed] [Google Scholar]
- Gultyaev A.P., Van Batenburg F.H.D., Pleij C.W.A. An approximation of loop free-energy values of RNA H-pseudoknots. RNA. 1999;5:609–617. [PMC free article] [PubMed] [Google Scholar]
- Hansen T.M., Reihani S.N.S., Oddershede L.B., Sørensen M.A. Correlation between mechanical strength of messenger RNA pseudoknots and ribosomal frameshifting. Proc. Natl. Acad. Sci. 2007;104:5830–5835. [PMC free article] [PubMed] [Google Scholar]
- Hart J.M., Kennedy S.D., Mathews D.H., Turner D.H. NMR-assisted prediction of RNA secondary structure: Identification of a probable pseudoknot in the coding region of an R2 retrotransposon. J. Am. Chem. Soc. 2008;130:10233–10239. [PMC free article] [PubMed] [Google Scholar]
- Held D.M., Kissel J.D., Saran D., Michalowski D., Burke D.H. Differential susceptibility of HIV-1 reverse transcriptase to inhibition by RNA aptamers in enzymatic reactions monitoring specific steps during genome replication. J. Biol. Chem. 2006a;281:25712–25722. [PubMed] [Google Scholar]
- Held D.M., Kissel J.D., Patterson J.T., Nickens D.G., Burke D.H. HIV-1 inactivation by nucleic acid aptamers. Front. Biosci. 2006b;11:89–112. [PubMed] [Google Scholar]
- Hofacker I.L. Vienna RNA secondary structure server. Nucleic Acids Res. 2003;31:3429–3431. [PMC free article] [PubMed] [Google Scholar]
- Huang X., Ali H. High sensitivity RNA pseudoknot prediction. Nucleic Acids Res. 2007;35:656–663. [PMC free article] [PubMed] [Google Scholar]
- Isambert H., Siggia E.D. Modeling RNA folding paths with pseudoknots: Application to hepatitis delta virus ribozyme. Proc. Natl. Acad. Sci. 2000;97:6515–6520. [PMC free article] [PubMed] [Google Scholar]
- Jabbari H., Condon A., Zhao S. Novel and efficient RNA secondary structure prediction using hierarchical folding. J. Comput. Biol. 2008;15:139–163. [PubMed] [Google Scholar]
- Jossinet F., Ludwig T.E., Westhof E. RNA structure: Bioinformatic analysis. Curr. Opin. Microbiol. 2007;10:279–285. [PubMed] [Google Scholar]
- Kopeikin Z., Chen S.J. Folding thermodynamics of pseudoknotted chain conformations. J. Chem. Phys. 2006;124:154903. doi: 10.1063/1.2188940. [PMC free article] [PubMed] [CrossRef] [Google Scholar]
- Li P.T.X., Vieregg J., Tinoco I., Jr How RNA unfolds and refolds. Annu. Rev. Biochem. 2008;77:77–100. [PubMed] [Google Scholar]
- Mathews D.H., Turner D.H. Prediction of RNA secondary structure by free-energy minimization. Curr. Opin. Struct. Biol. 2006;16:270–278. [PubMed] [Google Scholar]
- Mathews D.H., Sabina J., Zuker M., Turner D.H. Expanded sequence dependence of thermodynamic parameters improves prediction of RNA secondary structure. J. Mol. Biol. 1999;288:911–940. [PubMed] [Google Scholar]
- McCaskill J.S. The equilibrium partition function and base-pair binding probabilities for RNA secondary structure. Biopolymers. 1990;29:1105–1119. [PubMed] [Google Scholar]
- Metzler D., Nebel M.E. Predicting RNA secondary structures with pseudoknots by MCMC sampling. J. Math. Biol. 2008;56:161–181. [PubMed] [Google Scholar]
- Michiels P.J.A., Versleijen A.A.M., Verlaan P.W., Pleij C.W.A., Hilbers C.W., Heus H.A. Solution structure of the pseudoknot of SRV-1 RNA, involved in ribosomal frameshifting. J. Mol. Biol. 2001;310:1109–1123. [PMC free article] [PubMed] [Google Scholar]
- Murray L.J., Arendall W.B., III, Richardson D.C., Richardson J.S. RNA backbone is rotameric. Proc. Natl. Acad. Sci. 2003;100:13904–13909. [PMC free article] [PubMed] [Google Scholar]
- Murthy V.L., Srinivasan R., Draper D.E., Rose G.D. A complete conformational map for RNA. J. Mol. Biol. 1999;291:313–327. [PubMed] [Google Scholar]
- Namy O., Moran S.J., Stuart D.I., Gilbert R.J., Brierley I. A mechanical explanation of RNA pseudoknot function in programmed ribosomal frameshifting. Nature. 2006;441:244–247. [PMC free article] [PubMed] [Google Scholar]
- Nussinov R., Jacobson A.B. Fast algorithm for predicting the secondary structure of single-stranded RNA. Proc. Natl. Acad. Sci. 1980;77:6909–6913. [PMC free article] [PubMed] [Google Scholar]
- Nussinov R., Pieczenik G., Griggs J., Kleitman D. Algorithms for loop matchings. SIAM Rev. Soc. Ind. Appl. Math. 1978;35:68–82. [Google Scholar]
- Olson W.K. Configurational statistics of polynucleotide chains: An updated virtual bond model to treat effects of base stacking. Macromolecules. 1980;13:721–728. [Google Scholar]
- Olson W.K., Flory P.J. Spatial configurations of polynucleotide chains. I, Steric interactions in polyribonucleotides: A virtual bond model. Biopolymers. 1972;11:1–23. [PubMed] [Google Scholar]
- Pennell S., Manktelow E., Flatt A., Kelly G., Smerdon S.J., Brierley I. The stimulatory RNA of the Visna-Maedi retrovirus ribosomal frameshifting signal is an unusual pseudoknot with an interstem element. RNA. 2008;14:1366–1377. [PMC free article] [PubMed] [Google Scholar]
- Perrotta A.T., Been M.D. A pseudoknot-like structure required for efficient self-cleavage of hepatitis delta-virus RNA. Nature. 1991;350:434–436. [PubMed] [Google Scholar]
- Plant E.P., Jacobs K.L., Harger J.W., Meskauskas A., Jacobs J.L., Baxter J.L., Petrov A.N., Dinman J.D. The 9 Å solution: How mRNA pseudoknots promote efficient programmed −1 ribosomal frameshifting. RNA. 2003;9:168–174. [PMC free article] [PubMed] [Google Scholar]
- Reeder J., Giegerich R. Design, implementation, and evaluation of a practical pseudoknot folding algorithm based on thermodynamics. BMC Bioinformatics. 2004;5:104. doi: 10.1186/1471-2105-5-104. [PMC free article] [PubMed] [CrossRef] [Google Scholar]
- Reeder J., Hochsmann M., Rehmsmeier M., Voss B., Giegerich R. Beyond Mfold: Recent advances in RNA bioinformatics. J. Biotechnol. 2006;124:41–55. [PMC free article] [PubMed] [Google Scholar]
- Ren J., Rastegari B., Condon A., Hoos H.H. HotKnots: Heuristic prediction of RNA secondary structures including pseudoknots. RNA. 2005;11:1494–1504. [PMC free article] [PubMed] [Google Scholar]
- Richardson J.S., Schneider B., Murray L.W., Kapral G.J., Immormino R.M., Headd J.J., Richardson D.C., Ham D., Hershkovits E., Williams L.D., et al. RNA backbone: Consensus all-angle conformers and modular string nomenclature (an RNA Ontology Consortium contribution) RNA. 2008;14:465–481. [PMC free article] [PubMed] [Google Scholar]
- Rivas E., Eddy S.R. A dynamic programming algorithm for RNA structure prediction including pseudoknots. J. Mol. Biol. 1999;285:2053–2068. [PubMed] [Google Scholar]
- Ruan J., Stormo G.D., Zhang W. An iterated loop matching approach to the prediction of RNA secondary structures with pseudoknots. Bioinformatics. 2004;20:58–66. [PubMed] [Google Scholar]
- Schultes E.A., Bartel D.P. One sequence, two ribozymes: Implications for the emergence of new ribozyme folds. Science. 2000;289:448–452. [PubMed] [Google Scholar]
- Schuster P. Prediction of RNA secondary structures: From theory to models and real molecules. Rep. Prog. Phys. 2006;69:1419–1477. [Google Scholar]
- Serra M.J., Turner D.H. Predicting thermodynamic properties of RNA. Methods Enzymol. 1995;259:242–261. [PubMed] [Google Scholar]
- Shapiro B.A., Yingling Y.G., Kasprzak W., Bindewald E. Bridging the gap in RNA structure prediction. Curr. Opin. Struct. Biol. 2007;17:157–165. [PubMed] [Google Scholar]
- Somogyi P., Jenner A.J., Brierley I., Inglis S.C. Ribosomal pausing during translation of an RNA pseudoknot. Mol. Cell. Biol. 1993;13:6931–6940. [PMC free article] [PubMed] [Google Scholar]
- Sperschneider J., Datta A. KnotSeeker: Heuristic pseudoknot detection in long RNA sequences. RNA. 2008;14:630–640. [PMC free article] [PubMed] [Google Scholar]
- Staple D.W., Butcher S.E. Pseudoknots: RNA structures with diverse functions. PLoS Biol. 2005;3:e213. doi: 10.1371/journal.pbio.0030213. [PMC free article] [PubMed] [CrossRef] [Google Scholar]
- Su L., Chen L., Egli M., Berger J.M., Rich A. Minor groove RNA triplex in the crystal structure of a ribosomal frameshifting viral pseudoknot. Nat. Struct. Biol. 1999;6:285–292. [PMC free article] [PubMed] [Google Scholar]
- Tan Z.J., Chen S.-J. Salt dependence of nucleic acid hairpin stability. Biophys. J. 2008;95:738–752. [PMC free article] [PubMed] [Google Scholar]
- Tanner N.K., Schaff S., Thill G., Petitkoskas E., Craindenoyelle A.M., Westhof E. A three-dimensional model of hepatitis delta virus ribozyme based on biochemical and mutational analyses. Curr. Biol. 1994;4:488–498. [PubMed] [Google Scholar]
- ten Dam E., Verlaan P.W.G., Pleij C.W.A. Analysis of the role of the pseudoknot component in the SRV-1 gag-pro ribosomal frameshift signal: Loop lengths and stability of the stem regions. RNA. 1995;1:146–154. [PMC free article] [PubMed] [Google Scholar]
- Theimer C.A., Blois C.A., Feigon J. Structure of the human telomerase RNA pseudoknot reveals conserved tertiary interactions essential for function. Mol. Cell. 2005;17:671–682. [PubMed] [Google Scholar]
- Tuerk C., MacDougal S., Gold L. RNA pseudoknots that inhibit human immunodeficiency virus type 1 reverse transcriptase. Proc. Natl. Acad. Sci. 1992;89:6988–6992. [PMC free article] [PubMed] [Google Scholar]
- van Batenburg F.H.D., Gultyaev A.P., Pleij C.W.A., Ng J., Oliehoek J. Pseudobase: A database with RNA pseudoknots. Nucleic Acids Res. 2000;28:201–204. [PMC free article] [PubMed] [Google Scholar]
- van Belkum A., Abrahams J., Pleij C., Bosch L. Five pseudoknots are present at the 204 nucleotides long 3′ noncoding region of tobacco mosaic virus RNA. Nucleic Acids Res. 1985;13:7673–7686. [PMC free article] [PubMed] [Google Scholar]
- Wadley L.M., Keating K.S., Duarte C.M., Plye A.M. Evaluating and learning from RNA pseudotorsional space: Quantitative validation of a reduced representation for RNA structure. J. Mol. Biol. 2007;372:942–957. [PMC free article] [PubMed] [Google Scholar]
- Walter A.E., Turner D.H. Sequence dependence of stability for coaxial stacking of RNA helixes with Watson-Crick base paired interfaces. Biochemistry. 1994;33:12715–12719. [PubMed] [Google Scholar]
- Williams A.L., Jr, Tinoco I., Jr A dynamic programming algorithm for finding alternative RNA secondary structures. Nucleic Acids Res. 1986;14:299–315. [PMC free article] [PubMed] [Google Scholar]
- Zhang W.B., Chen S.J. A three-dimensional statistical mechanical model of folding double-stranded chain molecules. J. Chem. Phys. 2001;114:7669–7681. [Google Scholar]
- Zhang J., Lin M., Chen R., Wang W., Liang J. Discrete state model and accurate estimation of loop entropy of RNA secondary structures. J. Chem. Phys. 2008;128:125107. doi: 10.1063/1.2895050. [PMC free article] [PubMed] [CrossRef] [Google Scholar]
- Zuker M. On finding all suboptimal foldings of an RNA molecule. Science. 1989;244:48–52. [PubMed] [Google Scholar]
- Zwieb C., Wower I., Wower J. Comparative sequence analysis of tmRNA. Nucleic Acids Res. 1999;27:2063–2071. [PMC free article] [PubMed] [Google Scholar]
Articles from RNA are provided here courtesy of The RNA Society
Recommend
About Joyk
Aggregate valuable and interesting links.
Joyk means Joy of geeK