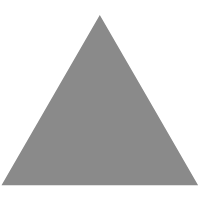
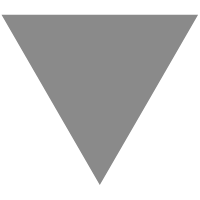
Analysing music habits with Spotify API and Python
source link: https://nvbn.github.io/2019/10/14/playlist-analysis/
Go to the source link to view the article. You can view the picture content, updated content and better typesetting reading experience. If the link is broken, please click the button below to view the snapshot at that time.
Analysing music habits with Spotify API and Python
Oct 14, 2019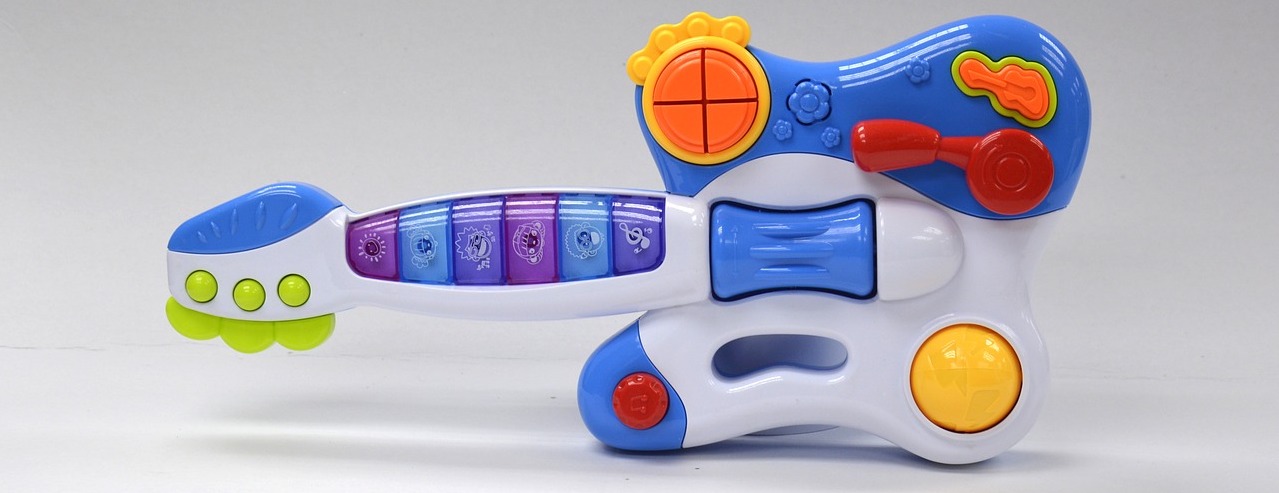
I’m using Spotify since 2013 as the main source of music, and back at that time the app automatically created a playlist for songs that I liked from artists’ radios. By innertion I’m still using the playlist to save songs that I like. As the playlist became a bit big and a bit old (6 years, huh), I’ve decided to try to analyze it.
Boring preparation
To get the data I used Spotify API and spotipy as a Python client. I’ve created an application in the Spotify Dashboard and gathered the credentials. Then I was able to initialize and authorize the client:
import spotipy
import spotipy.util as util
token = util.prompt_for_user_token(user_id,
'playlist-read-collaborative',
client_id=client_id,
client_secret=client_secret,
redirect_uri='http://localhost:8000/')
sp = spotipy.Spotify(auth=token)
Tracks metadata
As everything is inside just one playlist, it was easy to gather. The only problem was
that user_playlist
method in spotipy doesn’t support pagination and can only return the
first 100 track, but it was easily solved by just going down to private and undocumented
_get
:
playlist = sp.user_playlist(user_id, playlist_id)
tracks = playlist['tracks']['items']
next_uri = playlist['tracks']['next']
for _ in range(int(playlist['tracks']['total'] / playlist['tracks']['limit'])):
response = sp._get(next_uri)
tracks += response['items']
next_uri = response['next']
tracks_df = pd.DataFrame([(track['track']['id'],
track['track']['artists'][0]['name'],
track['track']['name'],
parse_date(track['track']['album']['release_date']) if track['track']['album']['release_date'] else None,
parse_date(track['added_at']))
for track in playlist['tracks']['items']],
columns=['id', 'artist', 'name', 'release_date', 'added_at'] )
tracks_df.head(10)
The first naive idea of data to get was the list of the most appearing artists:
tracks_df \
.groupby('artist') \
.count()['id'] \
.reset_index() \
.sort_values('id', ascending=False) \
.rename(columns={'id': 'amount'}) \
.head(10)
But as taste can change, I’ve decided to get top five artists from each year and check if I was adding them to the playlist in other years:
counted_year_df = tracks_df \
.assign(year_added=tracks_df.added_at.dt.year) \
.groupby(['artist', 'year_added']) \
.count()['id'] \
.reset_index() \
.rename(columns={'id': 'amount'}) \
.sort_values('amount', ascending=False)
in_top_5_year_artist = counted_year_df \
.groupby('year_added') \
.head(5) \
.artist \
.unique()
counted_year_df \
[counted_year_df.artist.isin(in_top_5_year_artist)] \
.pivot('artist', 'year_added', 'amount') \
.fillna(0) \
.style.background_gradient()
year_added 2013 2014 2015 2016 2017 2018 2019 artist Arcade Fire 2 0 0 1 3 0 0 Clinic 1 0 0 2 0 0 1 Crystal Castles 0 0 2 2 0 0 0 Depeche Mode 1 0 3 1 0 2 0 Die Antwoord 1 4 0 0 0 1 0 FM Belfast 3 3 0 0 0 0 0 Factory Floor 3 0 0 0 0 0 0 Fever Ray 3 1 1 0 1 0 0 Grimes 1 0 3 1 0 0 0 Holy Ghost! 1 0 0 0 3 1 1 Joe Goddard 0 0 0 0 3 1 0 John Maus 0 0 4 0 0 0 1 KOMPROMAT 0 0 0 0 0 0 2 LCD Soundsystem 0 0 1 0 3 0 0 Ladytron 5 1 0 0 0 1 0 Lindstrøm 0 0 0 0 0 0 2 Marie Davidson 0 0 0 0 0 0 2 Metronomy 0 1 0 6 0 1 1 Midnight Magic 0 4 0 0 1 0 0 Mr. Oizo 0 0 0 1 0 3 0 New Order 1 5 0 0 0 0 0 Pet Shop Boys 0 12 0 0 0 0 0 Röyksopp 0 4 0 3 0 0 0 Schwefelgelb 0 0 0 0 1 0 4 Soulwax 0 0 0 0 5 3 0 Talking Heads 0 0 3 0 0 0 0 The Chemical Brothers 0 0 2 0 1 0 3 The Fall 0 0 0 0 0 2 0 The Knife 5 1 3 1 0 0 1 The Normal 0 0 0 2 0 0 0 The Prodigy 0 0 0 0 0 2 0 Vitalic 0 0 0 0 2 2 0
As a bunch of artists was reappearing in different years, I decided to check if that correlates with new releases, so I’ve checked the last ten years:
counted_release_year_df = tracks_df \
.assign(year_added=tracks_df.added_at.dt.year,
year_released=tracks_df.release_date.dt.year) \
.groupby(['year_released', 'year_added']) \
.count()['id'] \
.reset_index() \
.rename(columns={'id': 'amount'}) \
.sort_values('amount', ascending=False)
counted_release_year_df \
[counted_release_year_df.year_released.isin(
sorted(tracks_df.release_date.dt.year.unique())[-11:]
)] \
.pivot('year_released', 'year_added', 'amount') \
.fillna(0) \
.style.background_gradient()
year_added 2013 2014 2015 2016 2017 2018 2019 year_released 2010.0 19 8 2 10 6 5 10 2011.0 14 10 4 6 5 5 5 2012.0 11 15 6 5 8 2 0 2013.0 28 17 3 6 5 4 2 2014.0 0 30 2 1 0 10 1 2015.0 0 0 15 5 8 7 9 2016.0 0 0 0 8 7 4 5 2017.0 0 0 0 0 23 5 5 2018.0 0 0 0 0 0 4 8 2019.0 0 0 0 0 0 0 14
Audio features
Spotify API has an endpoint that provides features like danceability, energy, loudness and etc for tracks. So I gathered features for all tracks from the playlist:
features = []
for n, chunk_series in tracks_df.groupby(np.arange(len(tracks_df)) // 50).id:
features += sp.audio_features([*map(str, chunk_series)])
features_df = pd.DataFrame.from_dict(filter(None, features))
tracks_with_features_df = tracks_df.merge(features_df, on=['id'], how='inner')
tracks_with_features_df.head()
After that I’ve checked changes in features over time, only instrumentalness had some visible difference:
sns.boxplot(x=tracks_with_features_df.added_at.dt.year,
y=tracks_with_features_df.instrumentalness)
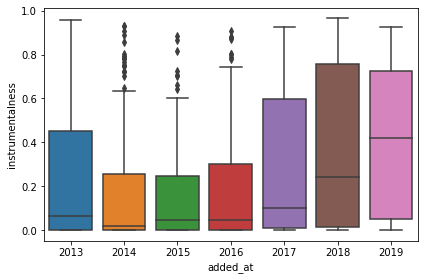
Then I had an idea to check seasonality and valence, and it kind of showed that in depressing months valence is a bit lower:
sns.boxplot(x=tracks_with_features_df.added_at.dt.month,
y=tracks_with_features_df.valence)
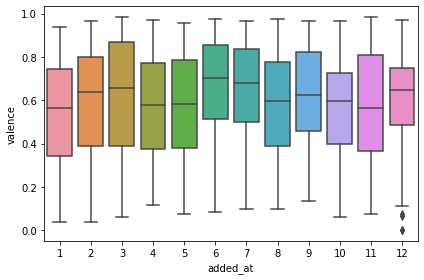
To play a bit more with data, I decided to check that danceability and valence might correlate:
tracks_with_features_df.plot(kind='scatter', x='danceability', y='valence')
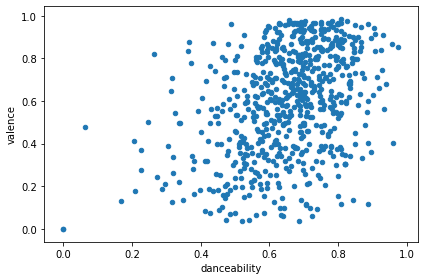
And to check that the data is meaningful, I checked instrumentalness vs speechiness, and those featues looked mutually exclusive as expected:
tracks_with_features_df.plot(kind='scatter', x='instrumentalness', y='speechiness')
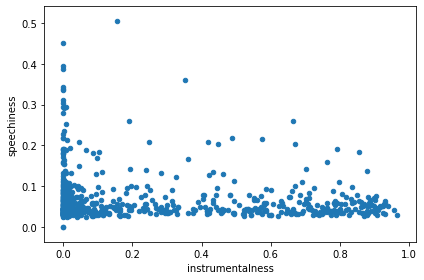
Tracks difference and similarity
As I already had a bunch of features classifying tracks, it was hard not to make vectors out of them:
encode_fields = [
'danceability',
'energy',
'key',
'loudness',
'mode',
'speechiness',
'acousticness',
'instrumentalness',
'liveness',
'valence',
'tempo',
'duration_ms',
'time_signature',
]
def encode(row):
return np.array([
(row[k] - tracks_with_features_df[k].min())
/ (tracks_with_features_df[k].max() - tracks_with_features_df[k].min())
for k in encode_fields])
tracks_with_features_encoded_df = tracks_with_features_df.assign(
encoded=tracks_with_features_df.apply(encode, axis=1))
Then I just calculated distance between every two tracks:
tracks_with_features_encoded_product_df = tracks_with_features_encoded_df \
.assign(temp=0) \
.merge(tracks_with_features_encoded_df.assign(temp=0), on='temp', how='left') \
.drop(columns='temp')
tracks_with_features_encoded_product_df = tracks_with_features_encoded_product_df[
tracks_with_features_encoded_product_df.id_x != tracks_with_features_encoded_product_df.id_y
]
tracks_with_features_encoded_product_df['merge_id'] = tracks_with_features_encoded_product_df \
.apply(lambda row: ''.join(sorted([row['id_x'], row['id_y']])), axis=1)
tracks_with_features_encoded_product_df['distance'] = tracks_with_features_encoded_product_df \
.apply(lambda row: np.linalg.norm(row['encoded_x'] - row['encoded_y']), axis=1)
After that I was able to get most similar songs/songs with the minimal distance, and it selected kind of similar songs:
tracks_with_features_encoded_product_df \
.sort_values('distance') \
.drop_duplicates('merge_id') \
[['artist_x', 'name_x', 'release_date_x', 'artist_y', 'name_y', 'release_date_y', 'distance']] \
.head(10)
The most different songs weren’t that fun, as two songs were too different from the rest:
tracks_with_features_encoded_product_df \
.sort_values('distance', ascending=False) \
.drop_duplicates('merge_id') \
[['artist_x', 'name_x', 'release_date_x', 'artist_y', 'name_y', 'release_date_y', 'distance']] \
.head(10)
Then I calculated the most avarage songs, eg the songs with the least distance from every other song:
tracks_with_features_encoded_product_df \
.groupby(['artist_x', 'name_x', 'release_date_x']) \
.sum()['distance'] \
.reset_index() \
.sort_values('distance') \
.head(10)
And totally opposite thing – the most outstanding songs:
tracks_with_features_encoded_product_df \
.groupby(['artist_x', 'name_x', 'release_date_x']) \
.sum()['distance'] \
.reset_index() \
.sort_values('distance', ascending=False) \
.head(10)
artist_x name_x release_date_x distance 665 YACHT Le Goudron - Long Version 2012-05-25 2823.572387 300 Labyrinth Ear Navy Light 2010-11-21 1329.234390 301 Labyrinth Ear Wild Flowers 2010-11-21 1329.234390 57 Blonde Redhead For the Damaged Coda 2000-06-06 1095.393120 616 The Velvet Underground After Hours 1969-03-02 1080.491779 593 The Knife Forest Families 2006-02-17 1040.114214 615 The Space Lady Major Tom 2013-11-18 1016.881467 107 CocoRosie By Your Side 2004-03-09 1015.970860 170 El Perro Del Mar Party 2015-02-13 1012.163212 403 Mr.Kitty XIII 2014-10-06 1010.115117
Conclusion
Although the dataset is a bit small, it was still fun to have a look at the data.
Gist with a jupyter notebook with even more boring stuff, can be reused by modifying credentials.
Recommend
About Joyk
Aggregate valuable and interesting links.
Joyk means Joy of geeK