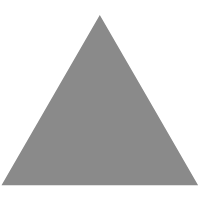
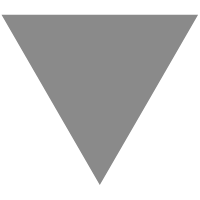
路透社文章的文本数据分析与可视化
source link: https://flashgene.com/archives/151258.html
Go to the source link to view the article. You can view the picture content, updated content and better typesetting reading experience. If the link is broken, please click the button below to view the snapshot at that time.
本站内容均来自兴趣收集,如不慎侵害的您的相关权益,请留言告知,我们将尽快删除.谢谢.
作者|Manmohan Singh 编译|VK 来源|Towards Datas Science
当我要求你解释文本数据时,你会怎幺做?你将采取什幺步骤来构建文本可视化?
本文将帮助你获得构建可视化和解释文本数据所需的信息。
从文本数据中获得的见解将有助于我们发现文章之间的联系。它将检测趋势和模式。对文本数据的分析将排除噪音,发现以前未知的信息。
这种分析过程也称为探索性文本分析(ETA)。运用K-means、Tf-IDF、词频等方法对这些文本数据进行分析。此外,ETA在数据清理过程中也很有用。
我们还使用Matplotlib、seaborn和Plotly库将结果可视化到图形、词云和绘图中。
在分析文本数据之前,请完成这些预处理任务。
从数据源检索数据
有很多非结构化文本数据可供分析。你可以从以下来源获取数据。
-
- 来自Kaggle的Twitter文本数据集。
-
- Reddit和twitter数据集使用API。
-
- 使用Beautifulsoup从网站上获取文章、。
我将使用路透社的SGML格式的文章。为了便于分析,我将使用beauthoulsoup库从数据文件中获取日期、标题和文章正文。
使用下面的代码从所有数据文件中获取数据,并将输出存储在单个CSV文件中。
from bs4 import BeautifulSoup import pandas as pd import csv article_dict = {} i = 0 list_of_data_num = [] for j in range(0,22): if j < 10: list_of_data_num.append("00" + str(j)) else: list_of_data_num.append("0" + str(j)) # 循环所有文章以提取日期、标题和文章主体 for num in list_of_data_num: try: soup = BeautifulSoup(open("data/reut2-" + num + ".sgm"), features='lxml') except: continue print(num) data_reuters = soup.find_all('reuters') for data in data_reuters: article_dict[i] = {} for date in data.find_all('date'): try: article_dict[i]["date"] = str(date.contents[0]).strip() except: article_dict[i]["date"] = None # print(date.contents[0]) for title in data.find_all('title'): article_dict[i]["title"] = str(title.contents[0]).strip() # print(title.contents) for text in data.find_all('text'): try: article_dict[i]["text"] = str(text.contents[4]).strip() except: article_dict[i]["text"] = None i += 1 dataframe_article = pd.DataFrame(article_dict).T dataframe_article.to_csv('articles_data.csv', header=True, index=False, quoting=csv.QUOTE_ALL) print(dataframe_article)
-
- 还可以使用Regex和OS库组合或循环所有数据文件。
-
- 每篇文章的正文以<Reuters>开头,因此使用find_all(‘reuters’)。
-
- 你也可以使用pickle模块来保存数据,而不是CSV。
清洗数据
在本节中,我们将从文本数据中移除诸如空值、标点符号、数字等噪声。首先,我们删除文本列中包含空值的行。然后我们处理另一列的空值。
import pandas as pd import re articles_data = pd.read_csv(‘articles_data.csv’) print(articles_data.apply(lambda x: sum(x.isnull()))) articles_nonNull = articles_data.dropna(subset=[‘text’]) articles_nonNull.reset_index(inplace=True) def clean_text(text): ‘’’Make text lowercase, remove text in square brackets,remove \n,remove punctuation and remove words containing numbers.’’’ text = str(text).lower() text = re.sub(‘<.*?>+’, ‘’, text) text = re.sub(‘[%s]’ % re.escape(string.punctuation), ‘’, text) text = re.sub(‘\n’, ‘’, text) text = re.sub(‘\w*\d\w*’, ‘’, text) return text articles_nonNull[‘text_clean’]=articles_nonNull[‘text’]\ .apply(lambda x:clean_text(x))
当我们删除文本列中的空值时,其他列中的空值也会消失。
我们使用re方法去除文本数据中的噪声。
数据清理过程中采取的步骤可能会根据文本数据增加或减少。因此,请仔细研究你的文本数据并相应地构建clean_text()方法。
随着预处理任务的完成,我们将继续分析文本数据。
让我们从分析开始。
1.路透社文章篇幅
我们知道所有文章的篇幅不一样。因此,我们将考虑长度等于或超过一段的文章。根据研究,一个句子的平均长度是15-20个单词。一个段落应该有四个句子。
articles_nonNull[‘word_length’] = articles_nonNull[‘text’].apply(lambda x: len(str(x).split())) print(articles_nonNull.describe()) articles_word_limit = articles_nonNull[articles_nonNull[‘word_length’] > 60] plt.figure(figsize=(12,6)) p1=sns.kdeplot(articles_word_limit[‘word_length’], shade=True, color=”r”).set_title(‘Kernel Distribution of Number Of words’)
我删除了那些篇幅不足60字的文章。
字长分布是右偏的。
大多数文章有150字左右。
包含事实或股票信息的路透社文章用词较少。
2.路透社文章中的常用词
在这一部分中,我们统计了文章中出现的字数,并对结果进行了分析。我们基于N-gram方法对词数进行了分析。N-gram是基于N值的单词的出现。
我们将从文本数据中删除停用词。因为停用词是噪音,在分析中没有太大用处。
1最常见的单字单词(N=1)
让我们在条形图中绘制unigram单词,并为unigram单词绘制词云。
from gensim.parsing.preprocessing import remove_stopwords import genism from wordcloud import WordCloud import numpy as np import random # 从gensim方法导入stopwords到stop_list变量 # 你也可以手动添加stopwords gensim_stopwords = gensim.parsing.preprocessing.STOPWORDS stopwords_list = list(set(gensim_stopwords)) stopwords_update = ["mln", "vs","cts","said","billion","pct","dlrs","dlr"] stopwords = stopwords_list + stopwords_update articles_word_limit['temp_list'] = articles_word_limit['text_clean'].apply(lambda x:str(x).split()) # 从文章中删除停用词 def remove_stopword(x): return [word for word in x if word not in stopwords] articles_word_limit['temp_list_stopw'] = articles_word_limit['temp_list'].apply(lambda x:remove_stopword(x)) # 生成ngram的单词 def generate_ngrams(text, n_gram=1): ngrams = zip(*[text[i:] for i in range(n_gram)]) return [' '.join(ngram) for ngram in ngrams] article_unigrams = defaultdict(int) for tweet in articles_word_limit['temp_list_stopw']: for word in generate_ngrams(tweet): article_unigrams[word] += 1 article_unigrams_df = pd.DataFrame(sorted(article_unigrams.items(), key=lambda x: x[1])[::-1]) N=50 # 在路透社的文章中前50个常用的unigram fig, axes = plt.subplots(figsize=(18, 50)) plt.tight_layout() sns.barplot(y=article_unigrams_df[0].values[:N], x=article_unigrams_df[1].values[:N], color='red') axes.spines['right'].set_visible(False) axes.set_xlabel('') axes.set_ylabel('') axes.tick_params(axis='x', labelsize=13) axes.tick_params(axis='y', labelsize=13) axes.set_title(f'Top {N} most common unigrams in Reuters Articles', fontsize=15) plt.show() # 画出词云 def col_func(word, font_size, position, orientation, font_path, random_state): colors = ['#b58900', '#cb4b16', '#dc322f', '#d33682', '#6c71c4', '#268bd2', '#2aa198', '#859900'] return random.choice(colors) fd = { 'fontsize': '32', 'fontweight' : 'normal', 'verticalalignment': 'baseline', 'horizontalalignment': 'center', } wc = WordCloud(width=2000, height=1000, collocations=False, background_color="white", color_func=col_func, max_words=200, random_state=np.random.randint(1, 8)) .generate_from_frequencies(article_unigrams) fig, ax = plt.subplots(figsize=(20,10)) ax.imshow(wc, interpolation='bilinear') ax.axis("off") ax.set_title(‘Unigram Words of Reuters Articles’, pad=24, fontdict=fd) plt.show()
Share, trade, stock是一些最常见的词汇,它们是基于股票市场和金融行业的文章。
因此,我们可以说,大多数路透社文章属于金融和股票类。
2.最常见的Bigram词(N=2)
让我们为Bigram单词绘制条形图和词云。
article_bigrams = defaultdict(int) for tweet in articles_word_limit[‘temp_list_stopw’]: for word in generate_ngrams(tweet, n_gram=2): article_bigrams[word] += 1 df_article_bigrams=pd.DataFrame(sorted(article_bigrams.items(), key=lambda x: x[1])[::-1]) N=50 # 前50个单词的柱状图 fig, axes = plt.subplots(figsize=(18, 50), dpi=100) plt.tight_layout() sns.barplot(y=df_article_bigrams[0].values[:N], x=df_article_bigrams[1].values[:N], color=’red’) axes.spines[‘right’].set_visible(False) axes.set_xlabel(‘’) axes.set_ylabel(‘’) axes.tick_params(axis=’x’, labelsize=13) axes.tick_params(axis=’y’, labelsize=13) axes.set_title(f’Top {N} most common Bigrams in Reuters Articles’, fontsize=15) plt.show() #词云 wc = WordCloud(width=2000, height=1000, collocations=False, background_color=”white”, color_func=col_func, max_words=200, random_state=np.random.randint(1,8))\ .generate_from_frequencies(article_bigrams) fig, ax = plt.subplots(figsize=(20,10)) ax.imshow(wc, interpolation=’bilinear’) ax.axis(“off”) ax.set_title(‘Trigram Words of Reuters Articles’, pad=24, fontdict=fd) plt.show()
Bigram比unigram提供更多的文本信息和上下文。比如,share loss显示:大多数人在股票上亏损。
3.最常用的Trigram词
让我们为trigma单词绘制条形图和词云。
article_trigrams = defaultdict(int) for tweet in articles_word_limit[‘temp_list_stopw’]: for word in generate_ngrams(tweet, n_gram=3): article_trigrams[word] += 1 df_article_trigrams = pd.DataFrame(sorted(article_trigrams.items(), key=lambda x: x[1])[::-1]) N=50 # 柱状图的前50个trigram fig, axes = plt.subplots(figsize=(18, 50), dpi=100) plt.tight_layout() sns.barplot(y=df_article_trigrams[0].values[:N], x=df_article_trigrams[1].values[:N], color=’red’) axes.spines[‘right’].set_visible(False) axes.set_xlabel(‘’) axes.set_ylabel(‘’) axes.tick_params(axis=’x’, labelsize=13) axes.tick_params(axis=’y’, labelsize=13) axes.set_title(f’Top {N} most common Trigrams in Reuters articles’, fontsize=15) plt.show() # 词云 wc = WordCloud(width=2000, height=1000, collocations=False, background_color=”white”, color_func=col_func, max_words=200, random_state=np.random.randint(1,8)).generate_from_frequencies(article_trigrams) fig, ax = plt.subplots(figsize=(20,10)) ax.imshow(wc, interpolation=’bilinear’) ax.axis(“off”) ax.set_title(‘Trigrams Words of Reuters Articles’, pad=24, fontdict=fd) plt.show()
大多数的三元组都与双元组相似,但无法提供更多信息。所以我们在这里结束这一部分。
3.文本数据的命名实体识别(NER)标记
NER是从文本数据中提取特定信息的过程。在NER的帮助下,我们从文本中提取位置、人名、日期、数量和组织实体。在这里了解NER的更多信息。我们使用Spacy python库来完成这项工作。
import spacy from matplotlib import cm from matplotlib.pyplot import plt nlp = spacy.load('en_core_web_sm') ner_collection = {"Location":[],"Person":[],"Date":[],"Quantity":[],"Organisation":[]} location = [] person = [] date = [] quantity = [] organisation = [] def ner_text(text): doc = nlp(text) ner_collection = {"Location":[],"Person":[],"Date":[],"Quantity":[],"Organisation":[]} for ent in doc.ents: if str(ent.label_) == "GPE": ner_collection['Location'].append(ent.text) location.append(ent.text) elif str(ent.label_) == "DATE": ner_collection['Date'].append(ent.text) person.append(ent.text) elif str(ent.label_) == "PERSON": ner_collection['Person'].append(ent.text) date.append(ent.text) elif str(ent.label_) == "ORG": ner_collection['Organisation'].append(ent.text) quantity.append(ent.text) elif str(ent.label_) == "QUANTITY": ner_collection['Quantity'].append(ent.text) organisation.append(ent.text) else: continue return ner_collection articles_word_limit['ner_data'] = articles_word_limit['text'].map(lambda x: ner_text(x)) location_name = [] location_count = [] for i in location_counts.most_common()[:10]: location_name.append(i[0].upper()) location_count.append(i[1]) fig, ax = plt.subplots(figsize=(15, 8), dpi=100) ax.barh(location_name, location_count, alpha=0.7, # width = 0.5, color=cm.Blues([i / 0.00525 for i in [ 0.00208, 0.00235, 0.00281, 0.00317, 0.00362, 0.00371, 0.00525, 0.00679, 0.00761, 0.00833]]) ) plt.rcParams.update({'font.size': 10}) rects = ax.patches for i, label in enumerate(location_count): ax.text(label+100 , i, str(label), size=10, ha='center', va='center') ax.text(0, 1.02, 'Count of Location name Extracted from Reuters Articles', transform=ax.transAxes, size=12, weight=600, color='#777777') ax.xaxis.set_ticks_position('bottom') ax.tick_params(axis='y', colors='black', labelsize=12) ax.set_axisbelow(True) ax.text(0, 1.08, 'TOP 10 Location Mention in Reuters Articles', transform=ax.transAxes, size=22, weight=600, ha='left') ax.text(0, -0.1, 'Source: http://kdd.ics.uci.edu/databases/reuters21578/reuters21578.html', transform=ax.transAxes, size=12, weight=600, color='#777777') ax.spines['right'].set_visible(False) ax.spines['top'].set_visible(False) ax.spines['left'].set_visible(False) ax.spines['bottom'].set_visible(False) plt.tick_params(axis='y',which='both', left=False, top=False, labelbottom=False) ax.set_xticks([]) plt.show()
从这个图表中,你可以说大多数文章都包含来自美国、日本、加拿大、伦敦和中国的新闻。
对美国的高度评价代表了路透在美业务的重点。
person变量表示1987年谁是名人。这些信息有助于我们了解这些人。
organization变量包含世界上提到最多的组织。
4.文本数据中的唯一词
我们将在使用TF-IDF的文章中找到唯一的词汇。词频(TF)是每篇文章的字数。反向文档频率(IDF)同时考虑所有提到的文章并衡量词的重要性,。
TF-IDF得分较高的词在一篇文章中的数量较高,而在其他文章中很少出现或不存在。
让我们计算TF-IDF分数并找出唯一的单词。
from sklearn.feature_extraction.text import TfidfVectorizer tfidf_vectorizer = TfidfVectorizer(use_idf=True) tfidf_vectorizer_vectors=tfidf_vectorizer.fit_transform(articles_word_limit[‘text_clean’]) tfidf = tfidf_vectorizer_vectors.todense() tfidf[tfidf == 0] = np.nan # 使用numpy的nanmean,在计算均值时忽略nan means = np.nanmean(tfidf, axis=0) # 将其转换为一个字典,以便以后查找 Means_words = dict(zip(tfidf_vectorizer.get_feature_names(), means.tolist()[0])) unique_words=sorted(means_words.items(), key=lambda x: x[1], reverse=True) print(unique_words)
5.用K-均值聚类文章
K-Means是一种无监督的机器学习算法。它有助于我们在一组中收集同一类型的文章。我们可以通过初始化k值来确定组或簇的数目。了解更多关于K-Means以及如何在这里选择K值。作为参考,我选择k=4。
from sklearn.feature_extraction.text import TfidfVectorizer from sklearn.cluster import KMeans from sklearn.metrics import adjusted_rand_score vectorizer = TfidfVectorizer(stop_words=’english’,use_idf=True) X = vectorizer.fit_transform(articles_word_limit[‘text_clean’]) k = 4 model = KMeans(n_clusters=k, init=’k-means++’, max_iter=100, n_init=1) model.fit(X) order_centroids = model.cluster_centers_.argsort()[:, ::-1] terms = vectorizer.get_feature_names() clusters = model.labels_.tolist() articles_word_limit.index = clusters for i in range(k): print(“Cluster %d words:” % i, end=’’) for title in articles_word_limit.ix[i [[‘text_clean’,’index’]].values.tolist(): print(‘ %s,’ % title, end=’’)
它有助于我们将文章按不同的组进行分类,如体育、货币、金融等。K-Means的准确性普遍较低。
结论
NER和K-Means是我最喜欢的分析方法。其他人可能喜欢N-gram和Unique words方法。在本文中,我介绍了最着名和闻所未闻的文本可视化和分析方法。本文中的所有这些方法都是独一无二的,可以帮助你进行可视化和分析。
我希望这篇文章能帮助你发现文本数据中的未知数。
Recommend
About Joyk
Aggregate valuable and interesting links.
Joyk means Joy of geeK