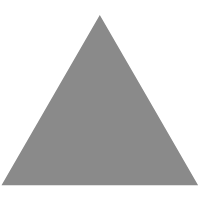
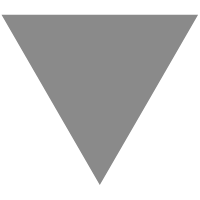
使用Python预测缺失值
source link: https://segmentfault.com/a/1190000024484295
Go to the source link to view the article. You can view the picture content, updated content and better typesetting reading experience. If the link is broken, please click the button below to view the snapshot at that time.
作者|Sadrach Pierre, Ph.D.
编译|VK
来源|Towards Data Science
对于数据科学家来说,处理丢失的数据是数据清理和模型开发过程中的一个重要部分。通常情况下,真实数据包含多个稀疏字段或包含错误值的字段。在这篇文章中,我们将讨论如何建立可以用来填补数据中缺失或错误值的模型。
出于我们的目的,我们将使用可以在这里找到的葡萄酒数据集: https://www.kaggle.com/zynici...
import pandas as pd df = pd.read_csv("winemag-data-130k-v2.csv")
接下来,让我们输出前五行数据:
print(df.head())
让我们从这些数据中随机抽取500条记录。这将有助于加快模型训练和测试,尽管读者可以很容易地对其进行修改:
import pandas as pd df = pd.read_csv("winemag-data-130k-v2.csv").sample(n=500, random_state = 42)
现在,让我们打印与数据对应的信息,这将使我们了解哪些列缺少值:
print(df.info())
有几个列的非空值小于500,这与缺少的值相对应。首先,让我们考虑建立一个模型,用“points”来估算缺失的“price”值。首先,让我们打印“price”和“points”之间的相关性:
print("Correlation: ", df['points'].corr(df['price']))
我们看到了一个微弱的正相关。让我们建立一个线性回归模型,用“points”来预测“price”。首先,让我们从“scikit learn”导入“LinearRegresssion”模块:
from sklearn.linear_model import LinearRegression
现在,让我们为训练和测试拆分数据。我们希望能够预测缺失值,但我们应该使用真实值“price”来验证我们的预测。让我们通过只选择正价格值来筛选缺少的值:
import numpy as np df_filter = df[df['price'] > 0].copy()
我们还可以初始化用于存储预测和实际值的列表:
y_pred = [] y_true = []
我们将使用K-fold交叉验证来验证我们的模型。让我们从“scikit learn”导入“KFolds”模块。我们将使用10折来验证我们的模型:
from sklearn.model_selection import KFold kf = KFold(n_splits=10, random_state = 42) for train_index, test_index in kf.split(df_filter): df_test = df_filter.iloc[test_index] df_train = df_filter.iloc[train_index]
我们现在可以定义我们的输入和输出:
for train_index, test_index in kf.split(df_filter): ... X_train = np.array(df_train['points']).reshape(-1, 1) y_train = np.array(df_train['price']).reshape(-1, 1) X_test = np.array(df_test['points']).reshape(-1, 1) y_test = np.array(df_test['price']).reshape(-1, 1)
并拟合我们的线性回归模型:
for train_index, test_index in kf.split(df_filter): ... model = LinearRegression() model.fit(X_train, y_train)
现在让我们生成并存储我们的预测:
for train_index, test_index in kf.split(df_filter): ... y_pred.append(model.predict(X_test)[0]) y_true.append(y_test[0])
现在让我们评估一下模型的性能。让我们用均方误差来评估模型的性能:
print("Mean Square Error: ", mean_squared_error(y_true, y_pred))
并不太好。我们可以通过训练平均价格加上一个标准差来改善这一点:
df_filter = df[df['price'] <= df['price'].mean() + df['price'].std() ].copy() ... print("Mean Square Error: ", mean_squared_error(y_true, y_pred))
虽然这大大提高了性能,但其代价是无法准确估算葡萄酒的price。与使用单一特征的回归模型预测价格不同,我们可以使用树基模型,例如随机森林模型,它可以处理类别和数值变量。
让我们建立一个随机森林回归模型,使用“country”、“province”、“variety”、“winery”和“points”来预测葡萄酒的“price”。首先,让我们将分类变量转换为可由随机森林模型处理的分类代码:
df['country_cat'] = df['country'].astype('category') df['country_cat'] = df['country_cat'].cat.codes df['province_cat'] = df['province'].astype('category') df['province_cat'] = df['province_cat'].cat.codes df['winery_cat'] = df['winery'].astype('category') df['winery_cat'] = df['winery_cat'].cat.codes df['variety_cat'] = df['variety'].astype('category') df['variety_cat'] = df['variety_cat'].cat.codes
让我们将随机样本大小增加到5000:
df = pd.read_csv("winemag-data-130k-v2.csv").sample(n=5000, random_state = 42)
接下来,让我们从scikit learn导入随机森林回归器模块。我们还可以定义用于训练模型的特征列表:
from sklearn.ensemble import RandomForestRegressor features = ['points', 'country_cat', 'province_cat', 'winery_cat', 'variety_cat']
让我们用一个随机森林来训练我们的模型,它有1000个估计量,最大深度为1000。然后,让我们生成预测并将其附加到新列表中:
for train_index, test_index in kf.split(df_filter): df_test = df_filter.iloc[test_index] df_train = df_filter.iloc[train_index] X_train = np.array(df_train[features]) y_train = np.array(df_train['price']) X_test = np.array(df_test[features]) y_test = np.array(df_test['price']) model = RandomForestRegressor(n_estimators = 1000, max_depth = 1000, random_state = 42) model.fit(X_train, y_train) y_pred_rf.append(model.predict(X_test)[0]) y_true_rf.append(y_test[0])
最后,让我们评估随机森林和线性回归模型的均方误差:
print("Mean Square Error (Linear Regression): ", mean_squared_error(y_true, y_pred)) print("Mean Square Error (Random Forest): ", mean_squared_error(y_pred_rf, y_true_rf))
我们看到随机森林模型具有优越的性能。现在,让我们使用我们的模型预测缺失的价格值,并显示price预测:
df_missing = df[df['price'].isnull()].copy() X_test_lr = np.array(df_missing['points']).reshape(-1, 1) X_test_rf = np.array(df_missing[features]) X_train_lr = np.array(df_filter['points']).reshape(-1, 1) y_train_lr = np.array(df_filter['price']).reshape(-1, 1) X_train_rf = np.array(df_filter[features]) y_train_rf = np.array(df_filter['price']) model_lr = LinearRegression() model_lr.fit(X_train_lr, y_train_lr) print("Linear regression predictions: ", model_lr.predict(X_test_lr)[0][0]) model_rf = RandomForestRegressor(n_estimators = 1000, max_depth = 1000, random_state = 42) model_rf.fit(X_train_rf, y_train_rf) print("Random forests regression predictions: ", model_rf.predict(X_test_rf)[0])
我就到此为止,但我鼓励你尝试一下特征选择和超参数调整,看看是否可以提高性能。此外,我鼓励你扩展此数据进行插补模型,以填补“region_1”和“designation”等分类字段中的缺失值。在这里,你可以构建一个基于树的分类模型,根据分类和数值特征来预测所列类别的缺失值。
结论
总而言之,在这篇文章中,我们讨论了如何建立机器学习模型,我们可以用来填补数据中的缺失值。首先,我们建立了一个线性回归模型,用以预测葡萄酒的价格。然后,我们建立了一个随机森林模型,用“points”和其他分类变量来预测葡萄酒价格。我们发现,随机森林模型显著优于基于线性回归的数据插补模型。本文中的代码可以在GitHub上找到。谢谢你的阅读!
Github链接: https://github.com/spierre91/...
原文链接: https://towardsdatascience.co...
欢迎关注磐创AI博客站:
http://panchuang.net/sklearn机器学习中文官方文档:
http://sklearn123.com/欢迎关注磐创博客资源汇总站:
http://docs.panchuang.net/Recommend
About Joyk
Aggregate valuable and interesting links.
Joyk means Joy of geeK