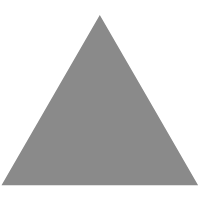
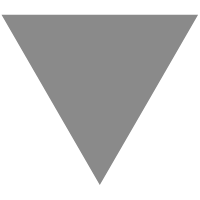
Tensorflow基础入门十大操作总结
source link: http://mp.weixin.qq.com/s?__biz=MzIyNjM2MzQyNg%3D%3D&%3Bmid=2247514734&%3Bidx=1&%3Bsn=7288a98b29063c75aa89020ea324ebee
Go to the source link to view the article. You can view the picture content, updated content and better typesetting reading experience. If the link is broken, please click the button below to view the snapshot at that time.
↑↑↑关注后" 星标 "Datawhale
每日干货 & 每月组队学习 ,不错过
Datawhale干货
作者:李祖贤, Datawhale高校群成员,深圳大学
TensorFlow 是一个开源的、基于 Python 的机器学习框架,它由 Google 开发,提供了 Python,C/C++、Java、Go、R 等多种编程语言的接口, 并 在图形分类、音频处理、推荐系统和自然语言处理等场景下有着丰富的应用,是目前最热门的机器学习框架。
但不 少小伙伴跟我吐苦水说 T ensorflow的应用太乱了,感觉学的云里雾里, 能不能搞个Tensorflow的教程呀。 今天,就和大家一起梳理下TensorFlow的十大基础操作。详情如下:
一、Tensorflow的排序与张量
Tensorflow允许用户把张量操作和功能定义为计算图。张量是通用的数学符号,代表保存数据值的多维列阵,张量的维数称为阶。
引用相关的库
import tensorflow as tf
import numpy as np
获取张量的阶(从下面例子看到tf的计算过程)
# 获取张量的阶(从下面例子看到tf的计算过程)
g = tf.Graph()
# 定义一个计算图
with g.as_default():
## 定义张量t1,t2,t3
t1 = tf.constant(np.pi)
t2 = tf.constant([1,2,3,4])
t3 = tf.constant([[1,2],[3,4]])
## 获取张量的阶
r1 = tf.rank(t1)
r2 = tf.rank(t2)
r3 = tf.rank(t3)
## 获取他们的shapes
s1 = t1.get_shape()
s2 = t2.get_shape()
s3 = t3.get_shape()
print("shapes:",s1,s2,s3)
# 启动前面定义的图来进行下一步操作
with tf.Session(graph=g) as sess:
print("Ranks:",r1.eval(),r2.eval(),r3.eval())
二、Tensorflow 计算图
Tensorflow 的核心在于构建计算图,并用计算图推导从输入到输出的所有张量之间的关系。
假设有0阶张量a,b,c,要评估 ,可以表示为下图所示的计算图:
可以看到,计算图就是一个节点网络,每个节点就像是一个操作,将函数应用到输入张量,然后返回0个或者更多个张量作为张量作为输出。
在Tensorflow编制计算图步骤如下:
1. 初始化一个空的计算图
2. 为该计算图加入节点(张量和操作)
3. 执行计算图:
a.开始一个新的会话
b.初始化图中的变量
c.运行会话中的计算图
# 初始化一个空的计算图
g = tf.Graph()
# 为该计算图加入节点(张量和操作)
with g.as_default():
a = tf.constant(1,name="a")
b = tf.constant(2,name="b")
c = tf.constant(3,name="c")
z = 2*(a-b)+c
# 执行计算图
## 通过调用tf.Session产生会话对象,该调用可以接受一个图为参数(这里是g),否则将启动默认的空图
## 执行张量操作的用sess.run(),他将返回大小均匀的列表
with tf.Session(graph=g) as sess:
print('2*(a-b)+c =>',sess.run(z))
2*(a-b)+c => 1
三、Tensorflow中的占位符
Tensorflow有提供数据的特别机制。其中一种机制就是使用占位符,他们是一些预先定义好类型和形状的张量。
通过调用tf.placeholder函数把这些张量加入计算图中,而且他们不包括任何数据。然而一旦执行图中的特定节点就需要提供数据阵列。
3.1 定义占位符
g = tf.Graph()
with g.as_default():
tf_a = tf.placeholder(tf.int32,shape=(),name="tf_a") # shape=[]就是定义0阶张量,更高阶张量可以用【n1,n2,n3】表示,如shape=(3,4,5)
tf_b = tf.placeholder(tf.int32,shape=(),name="tf_b")
tf_c = tf.placeholder(tf.int32,shape=(),name="tf_c")
r1 = tf_a - tf_b
r2 = 2*r1
z = r2 + tf_c
3.2 为占位符提供数据
当在图中处理节点的时候,需要产生python字典来为占位符来提供数据阵列。
with tf.Session(graph=g) as sess:
feed = {
tf_a:1,
tf_b:2,
tf_c:3
}
print('z:',sess.run(z,feed_dict=feed))
z: 1
3.3 用batch_sizes为数据阵列定义占位符
在研发神经网络模型的时候,有时会碰到大小规模不一致的小批量数据。占位符的一个功能是把大小无法确定的维度定义为None。
g = tf.Graph()
with g.as_default():
tf_x = tf.placeholder(tf.float32,shape=(None,2),name="tf_x")
x_mean = tf.reduce_mean(tf_x,axis=0,name="mean")
np.random.seed(123)
with tf.Session(graph=g) as sess:
x1 = np.random.uniform(low=0,high=1,size=(5,2))
print("Feeding data with shape",x1.shape)
print("Result:",sess.run(x_mean,feed_dict={tf_x:x1}))
x2 = np.random.uniform(low=0,high=1,size=(10,2))
print("Feeding data with shape",x2.shape)
print("Result:",sess.run(x_mean,feed_dict={tf_x:x2}))
四、Tensorflow 的变量
就Tensorflow而言,变量是一种特殊类型的张量对象,他允许我们在训练模型阶段,在tensorflow会话中储存和更新模型的参数。
4.1 定义变量
-
方式1:tf.Variable() 是为新变量创建对象并将其添加到计算图的类。
-
方式2:tf.get_variable()是假设某个变量名在计算图中,可以复用给定变量名的现有值或者不存在则创建新的变量,因此变量名的name非常重要!
无论采用哪种变量定义方式,直到调用tf.Session启动计算图并且在会话中具体运行了初始化操作后才设置初始值。事实上,只有初始化Tensorflow的变量之后才会为计算图分配内存。
g1 = tf.Graph()
with g1.as_default():
w = tf.Variable(np.array([[1,2,3,4],[5,6,7,8]]),name="w")
print(w)
4.2 初始化变量
由于变量是直到调用tf.Session启动计算图并且在会话中具体运行了初始化操作后才设置初始值,只有初始化Tensorflow的变量之后才会为计算图分配内存。因此这个初始化的过程十分重要,这个初始化过程包括:为 相关张量分配内存空间并为其赋予初始值。
初始化方式:
-
方式1.tf.global_variables_initializer函数,返回初始化所有计算图中现存的变量,要注意的是:定义变量一定要造初始化之前,不然会报错!!!
-
方式2:将tf.global_variables_initializer函数储存在init_op(名字不唯一,自己定)对象内,然后用sess.run出来
with tf.Session(graph=g1) as sess:
sess.run(tf.global_variables_initializer())
print(sess.run(w))
# 我们来比较定义变量与初始化顺序的关系
g2 = tf.Graph()
with g2.as_default():
w1 = tf.Variable(1,name="w1")
init_op = tf.global_variables_initializer()
w2 = tf.Variable(2,name="w2")
with tf.Session(graph=g2) as sess:
sess.run(init_op)
print("w1:",sess.run(w1))
w1: 1
with tf.Session(graph=g2) as sess: sess.run(init_op)
print("w2:",sess.run(w2))
4.3 变量范围
变量范围是一个重要的概念,对建设大型神经网络计算图特别有用。
可以把变量的域划分为独立的子部分。在创建变量时,该域内创建的操作与张量的名字都以域名为前缀,而且这些域可以嵌套。
g = tf.Graph()
with g.as_default():
with tf.variable_scope("net_A"): #定义一个域net_A
with tf.variable_scope("layer-1"): # 在域net_A下再定义一个域layer-1
w1 = tf.Variable(tf.random_normal(shape=(10,4)),name="weights") # 该变量定义在net_A/layer-1域下
with tf.variable_scope("layer-2"):
w2 = tf.Variable(tf.random_normal(shape=(20,10)),name="weights")
with tf.variable_scope("net_B"): # 定义一个域net_B
with tf.variable_scope("layer-2"):
w3 = tf.Variable(tf.random_normal(shape=(10,4)),name="weights")
print(w1)
print(w2)
print(w3)
五、建立回归模型
我们需要定义的变量:
-
1.输入x:占位符tf_x
-
2.输入y:占位符tf_y
-
3.模型参数w:定义为变量weight
-
4.模型参数b:定义为变量bias
-
5.模型输出 ̂ y^:有操作计算得到
import tensorflow as tf
import numpy as np
import matplotlib.pyplot as plt
%matplotlib inline
g = tf.Graph()
# 定义计算图
with g.as_default():
tf.set_random_seed(123)
## placeholder
tf_x = tf.placeholder(shape=(None),dtype=tf.float32,name="tf_x")
tf_y = tf.placeholder(shape=(None),dtype=tf.float32,name="tf_y")
## define the variable (model parameters)
weight = tf.Variable(tf.random_normal(shape=(1,1),stddev=0.25),name="weight")
bias = tf.Variable(0.0,name="bias")
## build the model
y_hat = tf.add(weight*tf_x,bias,name="y_hat")
## compute the cost
cost = tf.reduce_mean(tf.square(tf_y-y_hat),name="cost")
## train the model
optim = tf.train.GradientDescentOptimizer(learning_rate=0.001)
train_op = optim.minimize(cost,name="train_op")
# 创建会话启动计算图并训练模型
## create a random toy dataset for regression
np.random.seed(0)
def make_random_data():
x = np.random.uniform(low=-2,high=4,size=100)
y = []
for t in x:
r = np.random.normal(loc=0.0,scale=(0.5 + t*t/3),size=None)
y.append(r)
return x,1.726*x-0.84+np.array(y)
x,y = make_random_data()
plt.plot(x,y,'o')
plt.show()
## train/test splits
x_train,y_train = x[:100],y[:100]
x_test,y_test = x[100:],y[100:]
n_epochs = 500
train_costs = []
with tf.Session(graph=g) as sess:
sess.run(tf.global_variables_initializer())
## train the model for n_epochs
for e in range(n_epochs):
c,_ = sess.run([cost,train_op],feed_dict={tf_x:x_train,tf_y:y_train})
train_costs.append(c)
if not e % 50:
print("Epoch %4d: %.4f"%(e,c))
plt.plot(train_costs)
plt.show()
只需要把
sess.run([cost,train_op],feed_dict={tf_x:x_train,tf_y:y_train})
改为
sess.run(['cost:0','train_op:0'],feed_dict={'tf_x:0':x_train,'tf_y:0':y_train})
注意: 只有张量名才有:0后缀,操作是没有:0后缀的,例如train_op并没有train_op:0
## train/test splits
x_train,y_train = x[:100],y[:100]
x_test,y_test = x[100:],y[100:]
n_epochs = 500
train_costs = []
with tf.Session(graph=g) as sess:
sess.run(tf.global_variables_initializer())
## train the model for n_epochs
for e in range(n_epochs):
c,_ = sess.run(['cost:0','train_op'],feed_dict={'tf_x:0':x_train,'tf_y:0':y_train})
train_costs.append(c)
if not e % 50:
print("Epoch %4d: %.4f"%(e,c))
七、在Tensorflow中储存和恢复模型
神经网络训练可能需要几天几周的时间,因此我们需要把训练出来的模型储存下来供下次使用。
储存的方法是在定义计算图的时候加入:saver = tf.train.Saver(),并且在训练后输入saver.save(sess,'./trained-model')
g = tf.Graph()
# 定义计算图
with g.as_default():
tf.set_random_seed(123)
## placeholder
tf_x = tf.placeholder(shape=(None),dtype=tf.float32,name="tf_x")
tf_y = tf.placeholder(shape=(None),dtype=tf.float32,name="tf_y")
## define the variable (model parameters)
weight = tf.Variable(tf.random_normal(shape=(1,1),stddev=0.25),name="weight")
bias = tf.Variable(0.0,name="bias")
## build the model
y_hat = tf.add(weight*tf_x,bias,name="y_hat")
## compute the cost
cost = tf.reduce_mean(tf.square(tf_y-y_hat),name="cost")
## train the model
optim = tf.train.GradientDescentOptimizer(learning_rate=0.001)
train_op = optim.minimize(cost,name="train_op")
saver = tf.train.Saver()
# 创建会话启动计算图并训练模型
## create a random toy dataset for regression
np.random.seed(0)
def make_random_data():
x = np.random.uniform(low=-2,high=4,size=100)
y = []
for t in x:
r = np.random.normal(loc=0.0,scale=(0.5 + t*t/3),size=None)
y.append(r)
return x,1.726*x-0.84+np.array(y)
x,y = make_random_data()
plt.plot(x,y,'o')
plt.show()
## train/test splits
x_train,y_train = x[:100],y[:100]
x_test,y_test = x[100:],y[100:]
n_epochs = 500
train_costs = []
with tf.Session(graph=g) as sess:
sess.run(tf.global_variables_initializer())
## train the model for n_epochs
for e in range(n_epochs):
c,_ = sess.run(['cost:0','train_op'],feed_dict={'tf_x:0':x_train,'tf_y:0':y_train})
train_costs.append(c)
if not e % 50:
print("Epoch %4d: %.4f"%(e,c))
saver.save(sess,'C:/Users/Leo/Desktop/trained-model/')
# 加载保存的模型g2 = tf.Graph()
with tf.Session(graph=g2) as sess:
new_saver = tf.train.import_meta_graph("C:/Users/Leo/Desktop/trained-model/.meta")
new_saver.restore(sess,'C:/Users/Leo/Desktop/trained-model/')
y_pred = sess.run('y_hat:0',feed_dict={'tf_x:0':x_test})
## 可视化模型
x_arr = np.arange(-2,4,0.1)
g2 = tf.Graph()
with tf.Session(graph=g2) as sess:
new_saver = tf.train.import_meta_graph("C:/Users/Leo/Desktop/trained-model/.meta")
new_saver.restore(sess,'C:/Users/Leo/Desktop/trained-model/')
y_arr = sess.run('y_hat:0',feed_dict={'tf_x:0':x_arr})
plt.figure()
plt.plot(x_train,y_train,'bo')
plt.plot(x_test,y_test,'bo',alpha=0.3)
plt.plot(x_arr,y_arr.T[:,0],'-r',lw=3)
plt.show()
八、把张量转换成多维数据阵列
8.1 获得张量的形状
在numpy中我们可以用arr.shape来获得Numpy阵列的形状,而在Tensorflow中则用tf.get_shape函数完成:
注意:在tf.get_shape函数的结果是不可以索引的,需要用as.list()换成列表才能索引。
g = tf.Graph()
with g.as_default():
arr = np.array([[1.,2.,3.,3.5],[4.,5.,6.,6.5],[7.,8.,9.,9.5]])
T1 = tf.constant(arr,name="T1")
print(T1)
s = T1.get_shape()
print("Shape of T1 is ",s)
T2 = tf.Variable(tf.random_normal(shape=s))
print(T2)
T3 = tf.Variable(tf.random_normal(shape=(s.as_list()[0],)))
print(T3)
8.2 改变张量的形状
现在来看看Tensorflow如何改变张量的形状,在Numpy可以用np.reshape或arr.reshape,在一维的时候可以用-1来自动计算最后的维度。在Tensorflow内调用tf.reshape
with g.as_default():
T4 = tf.reshape(T1,shape=[1,1,-1],name="T4")
print(T4)
T5 = tf.reshape(T1,shape=[1,3,-1],name="T5")
print(T5)
with tf.Session(graph=g) as sess:
print(sess.run(T4))
print()
print(sess.run(T5))
with g.as_default():
tf_splt = tf.split(T5,num_or_size_splits=2,axis=2,name="T8")
print(tf_splt)
8.4 张量的拼接
g = tf.Graph()
with g.as_default():
t1 = tf.ones(shape=(5,1),dtype=tf.float32,name="t1")
t2 = tf.zeros(shape=(5,1),dtype=tf.float32,name="t2")
print(t1)
print(t2)
with g.as_default():
t3 = tf.concat([t1,t2],axis=0,name="t3")
print(t3)
t4 = tf.concat([t1,t2],axis=1,name="t4")
print(t4)
with tf.Session(graph=g) as sess:
print(t3.eval())
print()
print(t4.eval())
with tf.Session(graph=g) as sess: print(sess.run(t3))
print()
print(sess.run(t4))
这里主要讨论在Tensorflow执行像python一样的if语句,循环while语句,if...else..语句等。
9.1 条件语句
tf.cond()语句 我们来试试:
x,y = 1.0,2.0
g = tf.Graph()
with g.as_default():
tf_x = tf.placeholder(dtype=tf.float32,shape=None,name="tf_x")
tf_y = tf.placeholder(dtype=tf.float32,shape=None,name="tf_y")
res = tf.cond(tf_x<tf_y,lambda: tf.add(tf_x,tf_y,name="result_add"),lambda: tf.subtract(tf_x,tf_y,name="result_sub"))
print("Object:",res) #对象被命名为"cond/Merge:0"
with tf.Session(graph=g) as sess:
print("x<y: %s -> Result:"%(x<y),res.eval(feed_dict={"tf_x:0":x,"tf_y:0":y}))
x,y = 2.0,1.0
print("x<y: %s -> Result:"%(x<y),res.eval(feed_dict={"tf_x:0":x,"tf_y:0":y}))
9.2 执行python的if...else语句
tf.case()
f1 = lambda: tf.constant(1)
f2 = lambda: tf.constant(0)
result = tf.case([(tf.less(x,y),f1)],default=f2)
print(result)
9.3 执行python的while语句
tf.while_loop()
i = tf.constant(0)
threshold = 100
c = lambda i: tf.less(i,100)
b = lambda i: tf.add(i,1)
r = tf.while_loop(cond=c,body=b,loop_vars=[i])
print(r)
十、用TensorBoard可视化图
TensorBoard是Tensorflow一个非常好的工具,它负责可视化和模型学习。可视化允许我们看到节点之间的连接,探索它们之间的依赖关系,并且在需要的时候进行模型调试。
def build_classifier(data, labels, n_classes=2):
data_shape = data.get_shape().as_list()
weights = tf.get_variable(name='weights',
shape=(data_shape[1], n_classes),
dtype=tf.float32)
bias = tf.get_variable(name='bias',
initializer=tf.zeros(shape=n_classes))
print(weights)
print(bias)
logits = tf.add(tf.matmul(data, weights),
bias,
name='logits')
print(logits)
return logits, tf.nn.softmax(logits)
def build_generator(data, n_hidden):
data_shape = data.get_shape().as_list()
w1 = tf.Variable(
tf.random_normal(shape=(data_shape[1],
n_hidden)),
name='w1')
b1 = tf.Variable(tf.zeros(shape=n_hidden),
name='b1')
hidden = tf.add(tf.matmul(data, w1), b1,
name='hidden_pre-activation')
hidden = tf.nn.relu(hidden, 'hidden_activation')
w2 = tf.Variable(
tf.random_normal(shape=(n_hidden,
data_shape[1])),
name='w2')
b2 = tf.Variable(tf.zeros(shape=data_shape[1]),
name='b2')
output = tf.add(tf.matmul(hidden, w2), b2,
name = 'output')
return output, tf.nn.sigmoid(output)
batch_size=64
g = tf.Graph()
with g.as_default():
tf_X = tf.placeholder(shape=(batch_size, 100),
dtype=tf.float32,
name='tf_X')
## build the generator
with tf.variable_scope('generator'):
gen_out1 = build_generator(data=tf_X,
n_hidden=50)
## build the classifier
with tf.variable_scope('classifier') as scope:
## classifier for the original data:
cls_out1 = build_classifier(data=tf_X,
labels=tf.ones(
shape=batch_size))
## reuse the classifier for generated data
scope.reuse_variables()
cls_out2 = build_classifier(data=gen_out1[1],
labels=tf.zeros(
shape=batch_size))
init_op = tf.global_variables_initializer()
with tf.Session(graph=g) as sess:
sess.run(tf.global_variables_initializer())
file_writer = tf.summary.FileWriter(logdir="C:/Users/Leo/Desktop/trained-model/logs/",graph=g)
在win+R输入cmd后 输入命令:
tensorboard --logdir="C:/Users/Leo/Desktop/trained-model/logs"
接着复制这个链接到浏览器打开:
本文电子版 后台回复 TF 获取
“给Datawhale 点个 赞 吧 ↓
Recommend
About Joyk
Aggregate valuable and interesting links.
Joyk means Joy of geeK