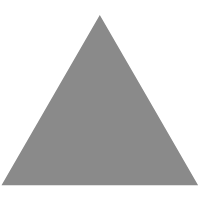
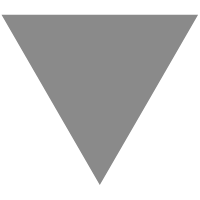
Introducing TayPO, a Unifying Framework for Reinforcement Learning
source link: https://syncedreview.com/2020/07/14/introducing-taypo-a-unifying-framework-for-reinforcement-learning/
Go to the source link to view the article. You can view the picture content, updated content and better typesetting reading experience. If the link is broken, please click the button below to view the snapshot at that time.
A team of researchers from Columbia University and DeepMind have proposed a Taylor Expansion Policy Optimization (TayPO) framework that combines two leading algorithmic improvement methods.

Policy optimization is a major framework in model-free reinforcement learning (RL), providing insights that can drive significant algorithmic performance gains. Two of the most prominent such algorithmic improvements are trust-region policy search and off-policy corrections — and these idea streams are usually evaluated separately. In the paper Taylor Expansion Policy Optimization, researchers partially unify these algorithmic ideas into a single framework, showing how Taylor expansions — a method based on the Taylor series concept used to describe and approximate math functions — share high-level similarities with both trust-region policy search and off-policy corrections. The paper was presented this week at ICML2020.
In most previous research on trust-region policy search, the main idea is to constrain the size of policy updates to limit the deviations between consecutive policies and lower-bound the performance of a new policy. Off-policy corrections meanwhile require accounting for discrepancies between target policies and behaviour policies. The researchers propose that the inherent notion of a trust-region constraint is a common feature shared by Taylor expansions and trust-region policy search, and that Taylor expansions also satisfy the requirement for off-policy evaluations.
This paper illustrates how Taylor expansions construct approximations to the full IS (Importance Sampling) corrections, which are at the core of most off-policy evaluation techniques and hence intimately relate to established off-policy evaluation techniques. Prior work has focused on applying off-policy corrections directly to policy gradient estimators instead of the surrogate objectives which generate the gradients. The researchers note that although standard policy optimization objectives involve IS weights, their link with IS is not made explicit. The use of Taylor expansions resolves the implicit link between standard policy optimization objectives and IS.
The researchers evaluated the benefits of applying the Taylor expansions across a diverse set of scenarios. The experiment results indicate that second-order correction leads to marginally better performance than first-order and retrace, and is significantly better than zero-order. In general, unbiased (or slightly biased) off-policy corrections do not yet perform as well as radically biased off-policy variants. All in all, this new formulation can bring significant gains to state-of-the-art deep RL agents.
The paper Taylor Expansion Policy Optimization is on arXiv .
Author: Grace Duan | Editor : Michael Sarazen & Fangyu Cai

Synced Report | A Survey of China’s Artificial Intelligence Solutions in Response to the COVID-19 Pandemic — 87 Case Studies from 700+ AI Vendors
This report offers a look at how the Chinese government and business owners have leveraged artificial intelligence technologies in the battle against COVID-19. It is also available on Amazon Kindle .
Click here to find more reports from us.
We know you don’t want to miss any story. Subscribe to our popular Synced Global AI Weekly to get weekly AI updates.

Advertisements
Recommend
About Joyk
Aggregate valuable and interesting links.
Joyk means Joy of geeK