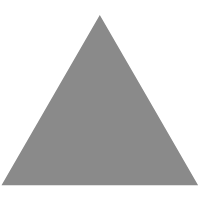
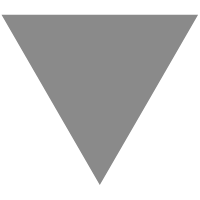
BiLSTM的PyTorch应用
source link: https://www.wmathor.com/index.php/archives/1447/
Go to the source link to view the article. You can view the picture content, updated content and better typesetting reading experience. If the link is broken, please click the button below to view the snapshot at that time.
本文介绍一下如何使用BiLSTM(基于PyTorch)解决一个实际问题,实现 给定一个长句子预测下一个单词
如果不了解LSTM的同学请先看我的这两篇文章LSTM、PyTorch中的LSTM。下面直接开始代码讲解
导库
''' code by Tae Hwan Jung(Jeff Jung) @graykode, modify by wmathor ''' import torch import numpy as np import torch.nn as nn import torch.optim as optim import torch.utils.data as Data dtype = torch.FloatTensor
准备数据
sentence = ( 'GitHub Actions makes it easy to automate all your software workflows ' 'from continuous integration and delivery to issue triage and more' ) word2idx = {w: i for i, w in enumerate(list(set(sentence.split())))} idx2word = {i: w for i, w in enumerate(list(set(sentence.split())))} n_class = len(word2idx) # classification problem max_len = len(sentence.split()) n_hidden = 5
我水平不佳,一开始看到这个 sentence
不懂这种写法是什么意思,如果你调用 type(sentence)
以及打印 sentence
就会知道,这其实就是个字符串,就是将上下两行字符串连接在一起的一个大字符串
数据预处理,构建dataset,定义dataloader
def make_data(sentence): input_batch = [] target_batch = [] words = sentence.split() for i in range(max_len - 1): input = [word2idx[n] for n in words[:(i + 1)]] input = input + [0] * (max_len - len(input)) target = word2idx[words[i + 1]] input_batch.append(np.eye(n_class)[input]) target_batch.append(target) return torch.Tensor(input_batch), torch.LongTensor(target_batch) # input_batch: [max_len - 1, max_len, n_class] input_batch, target_batch = make_data(sentence) dataset = Data.TensorDataset(input_batch, target_batch) loader = Data.DataLoader(dataset, 16, True)
这里面的循环还是有点复杂的,尤其是 input
和 input_batch
里面存的东西,很难理解。所以下面我会详细解释
首先开始循环, input
的第一个赋值语句会将第一个词 Github
对应的索引存起来。 input
的第二个赋值语句会将剩下的 max_len - len(input)
都用0去填充
第二次循环, input
的第一个赋值语句会将前两个词 Github
和 Actions
对应的索引存起来。 input
的第二个赋值语句会将剩下的 max_len - len(input)
都用0去填充
每次循环, input
和 target
中所存的 索引转换成word
如下图所示,因为我懒得去查看每个词对应的索引是什么,所以干脆直接写出存在其中的词
从上图可以看出, input
的长度永远保持 max_len(=21)
,并且循环了 max_len-1
次,所以最终 input_batch
的维度是 [max_len - 1, max_len, n_class]
定义网络架构
class BiLSTM(nn.Module): def __init__(self): super(BiLSTM, self).__init__() self.lstm = nn.LSTM(input_size=n_class, hidden_size=n_hidden, bidirectional=True) # fc self.fc = nn.Linear(n_hidden * 2, n_class) def forward(self, X): # X: [batch_size, max_len, n_class] batch_size = X.shape[0] input = X.transpose(0, 1) # input : [max_len, batch_size, n_class] hidden_state = torch.randn(1*2, batch_size, n_hidden) # [num_layers(=1) * num_directions(=2), batch_size, n_hidden] cell_state = torch.randn(1*2, batch_size, n_hidden) # [num_layers(=1) * num_directions(=2), batch_size, n_hidden] outputs, (_, _) = self.lstm(input, (hidden_state, cell_state)) outputs = outputs[-1] # [batch_size, n_hidden * 2] model = self.fc(outputs) # model : [batch_size, n_class] return model model = BiLSTM() criterion = nn.CrossEntropyLoss() optimizer = optim.Adam(model.parameters(), lr=0.001)
Bi-LSTM的网络结构图如下所示,其中Backward Layer意思不是"反向传播",而是将"句子反向输入"。具体流程就是,现有有由四个词构成的一句话"i like your friends"。常规单向LSTM的做法就是直接输入"i like your",然后预测出"friends",而双向LSTM会同时输入"i like your"和"your like i",然后将Forward Layer和Backward Layer的output进行concat(这样做可以理解为同时"汲取"正向和反向的信息),最后预测出"friends"
而正因为多了一个反向的输入,所以整个网络结构中很多隐藏层的输入和输出的某些维度会变为原来的两倍,具体如下图所示。对于双向LSTM来说, num_directions = 2
训练&测试
# Training for epoch in range(10000): for x, y in loader: pred = model(x) loss = criterion(pred, y) if (epoch + 1) % 1000 == 0: print('Epoch:', '%04d' % (epoch + 1), 'cost =', '{:.6f}'.format(loss)) optimizer.zero_grad() loss.backward() optimizer.step() # Pred predict = model(input_batch).data.max(1, keepdim=True)[1] print(sentence) print([idx2word[n.item()] for n in predict.squeeze()])
Recommend
About Joyk
Aggregate valuable and interesting links.
Joyk means Joy of geeK