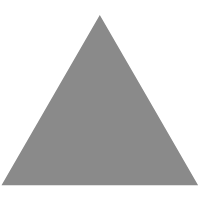
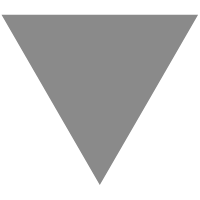
Cross-Attention is what you need! FusAtNet Fusion Network.
source link: https://mc.ai/cross-attention-is-what-you-need-fusatnet-fusion-network/
Go to the source link to view the article. You can view the picture content, updated content and better typesetting reading experience. If the link is broken, please click the button below to view the snapshot at that time.
Today, with recent advances in sensing, multimodal data is becoming easily available for various applications, especially in remote sensing (RS), where many data types like multispectral (MSI), hyperspectral (HSI), LiDAR etc. are available.
Effective fusion of these multi source datasets is becoming important, for these multi modality features have been shown to generate highly accurate land-cover maps. However, fusion in the context of RS is non-trivial considering the redundancy involved in the data and the large domain differences among multiple modalities. In addition, the feature extraction modules for different modalities hardly interact among themselves, which further limits their semantic relatedness.
Why is a single fused representation important?
Several advantages of combining multimodal images, including :
- generating a rich, fused representation, helps select task relevant features
- improved classification, increased confidence, reduced ambiguity
- complements missing or noisy data
- reducing data size
Interestingly, most common methods today often just use methods like early concatenation, CNN extracted feature level concatenation or multi-stream decision level fusion methods, totally overlooking cross-domain features. Visual a ttention , a recent addition to the deep-learning-researchers’ toolbox is largely unexplored in multi-modal domain.
A question arises: How to best fuse these modalities for a joint, rich representation which can be used in downstream tasks?
An ideal fusion method would synergistically combine the two modalities and ensure that the resultant product reflects the salient features of input modalities.
A New Concept: Cross Attention
In this work, we propose a new concept of “cross-attention and propose attention based HSI-LiDAR fusion in the context of land-cover classification.
Cross attention is a a novel and intuitive fusion method in which attention masks from one modality (hereby LiDAR) are used to highlight the extracted features in another modality (hereby HSI). Note that this is different from self-attention where attention mask from HSI is used to highlight its own spectral features.
FusAtNet: Using Cross Attention in practice
We propose a feature fusion and extraction framework, namely FusAtNet, for collective land-cover classification of HSIs and LiDAR data in this paper. The proposed framework effectively utilizses HSI modality to generate an attention map using “self-attention” mechanism that highlights its own spectral features. Similarly, a “cross-attention” approach is simultaneously used to harness the LiDAR derived attention map that accentuates the spatial features of HSI. These attentive spectral and spatial representations are then explored further along with the original data to obtain modality-specific feature embeddings. The modality oriented joint spectro-spatial information thus obtained, is subsequently utilized to carry out the land-cover classification task.
Results
Experimental evaluations on three HSI-LiDAR datasets show that the proposed method achieves the state-of-the-art classification performance, including on the largest HSI-LiDAR benchmark dataset available, Houston, opening new avenues in multimodality feature fusion classification.
Houston
Recommend
About Joyk
Aggregate valuable and interesting links.
Joyk means Joy of geeK