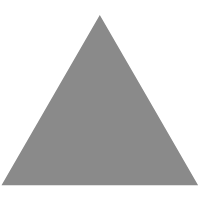
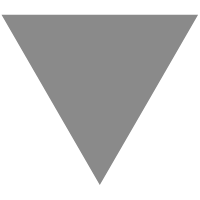
Alink漫谈(六) : TF-IDF算法的实现
source link: http://www.cnblogs.com/rossiXYZ/p/13052449.html
Go to the source link to view the article. You can view the picture content, updated content and better typesetting reading experience. If the link is broken, please click the button below to view the snapshot at that time.
Alink漫谈(六) : TF-IDF算法的实现
目录
- Alink漫谈(六) : TF-IDF算法的实现
0x00 摘要
Alink 是阿里巴巴基于实时计算引擎 Flink 研发的新一代机器学习算法平台,是业界首个同时支持批式算法、流式算法的机器学习平台。TF-IDF(term frequency–inverse document frequency)是一种用于信息检索与数据挖掘的常用加权技术。本文将为大家展现Alink如何实现TF-IDF。
0x01 TF-IDF
TF-IDF(term frequency–inverse document frequency)是一种统计方法,一种用于信息检索与数据挖掘的常用加权技术。
TF是词频(Term Frequency),IDF是逆文本频率指数(Inverse Document Frequency)。
为什么要用TF-IDF? 因为计算机只能识别数字,对于一个一个的单词,计算机是看不懂的 ,更别说是一句话,或是一篇文章。 而TF-IDF就是用来将文本转换成计算机看得懂的语言,或者说是机器学习或深度学习模型能够进行学习训练的数据集 。
1.1 原理
TF-IDF用以评估一个词对于一个文件集或一个语料库中的其中一份文件的重要程度。字词的重要性随着它在文件中出现的次数成正比增加,但同时会随着它在语料库中出现的频率成反比下降。
TF-IDF的主要思想是:如果某个词或短语在一篇文章中出现的频率TF高,并且在其他文章中很少出现,则认为此词或者短语具有很好的类别区分能力,适合用来分类。
TF-IDF实际上是:TF * IDF,TF词频(Term Frequency),IDF逆向文件频率(Inverse Document Frequency)。
词频(term frequency,TF)指的是某一个给定的词语在该文件中出现的频率。这个数字是对 词数 (term count)的归一化,以防止它偏向长的文件(同一个词语在长文件里可能会比短文件有更高的词数,而不管该词语重要与否)。
而 IDF逆向文件频率 (inverse document frequency, IDF) 反应了一个词在 所有文本(整个文档) 中出现的频率, 如果一个词在很多的文本中出现,那么它的IDF值应该低。而反过来如果一个词在比较少的文本中出现,那么它的IDF值应该高 。比如一些专业的名词如“Machine Learning”。这样的词IDF值应该高。一个极端的情况,如果一个词在所有的文本中都出现,那么它的IDF值应该为0。
如果单单以TF或者IDF来计算一个词的重要程度都是片面的,因此TF-IDF综合了TF和IDF两者的优点,用以评估 一字词 对于一个文件集或一个语料库中的其中一份文件的重要程度。字词的重要性随着它在文件中出现的次数成正比增加,但同时会随着它在语料库中出现的频率成反比下降。上述引用总结就是: 一个词语在一篇文章中出现次数越多, 同时在所有文档中出现次数越少, 越能够代表该文章,越能与其它文章区分开来。
1.2 计算方法
TF的计算公式如下:
\[TF_w = \frac {N_w}{N} \]
其中 N_w 是在某一文本中词条w出现的次数,N 是该文本总词条数。
IDF的计算公式如下:
\[IDF_w = log(\frac {Y}{Y_w + 1}) \]
其中 Y 是语料库的文档总数,Y_w 是包含词条w的文档数,分母加一是为了避免w 未出现在任何文档中从而导致分母为0 的情况。
TF-IDF 就是将TF和IDF相乘 :
\[TF-IDF_w = TF_w * IDF_w \]
从以上计算公式便可以看出,某一特定文件内的高词语频率,以及该词语在整个文件集合中的低文件频率,可以产生出高权重的TF-IDF。因此, TF-IDF倾向于过滤掉常见的词语,保留重要的词语。
0x02 Alink示例代码
2.1 示例代码
首先我们给出示例代码,下文是通过一些语料来训练出一个模型,然后用这个模型来做预测:
public class DocCountVectorizerExample { AlgoOperator getData(boolean isBatch) { Row[] rows = new Row[]{ Row.of(0, "二手旧书:医学电磁成像"), Row.of(1, "二手美国文学选读( 下册 )李宜燮南开大学出版社 9787310003969"), Row.of(2, "二手正版图解象棋入门/谢恩思主编/华龄出版社"), Row.of(3, "二手中国糖尿病文献索引"), Row.of(4, "二手郁达夫文集( 国内版 )全十二册馆藏书") }; String[] schema = new String[]{"id", "text"}; if (isBatch) { return new MemSourceBatchOp(rows, schema); } else { return new MemSourceStreamOp(rows, schema); } } public static void main(String[] args) throws Exception { DocCountVectorizerExample test = new DocCountVectorizerExample(); BatchOperator batchData = (BatchOperator) test.getData(true); // 分词 SegmentBatchOp segment = new SegmentBatchOp() .setSelectedCol("text") .linkFrom(batchData); // TF-IDF训练 DocCountVectorizerTrainBatchOp model = new DocCountVectorizerTrainBatchOp() .setSelectedCol("text") .linkFrom(segment); // TF-IDF预测 DocCountVectorizerPredictBatchOp predictBatch = new DocCountVectorizerPredictBatchOp() .setSelectedCol("text") .linkFrom(model, segment); model.print(); predictBatch.print(); } }
2.2 TF-IDF模型
TF-IDF模型打印出来如下:
model_id|model_info --------|---------- 0|{"minTF":"1.0","featureType":"\"WORD_COUNT\""} 1048576|{"f0":"二手","f1":0.0,"f2":0} 2097152|{"f0":"/","f1":1.0986122886681098,"f2":1} 3145728|{"f0":"出版社","f1":0.6931471805599453,"f2":2} 4194304|{"f0":")","f1":0.6931471805599453,"f2":3} 5242880|{"f0":"(","f1":0.6931471805599453,"f2":4} 6291456|{"f0":"入门","f1":1.0986122886681098,"f2":5} ...... 36700160|{"f0":"美国","f1":1.0986122886681098,"f2":34} 37748736|{"f0":"谢恩","f1":1.0986122886681098,"f2":35} 38797312|{"f0":"象棋","f1":1.0986122886681098,"f2":36}
2.3 TF-IDF预测
TF-IDF预测结果如下:
id|text --|---- 0|$37$0:1.0 6:1.0 10:1.0 25:1.0 26:1.0 28:1.0 1|$37$0:1.0 1:1.0 2:1.0 4:1.0 11:1.0 15:1.0 16:1.0 19:1.0 20:1.0 32:1.0 34:1.0 2|$37$0:1.0 3:2.0 4:1.0 5:1.0 8:1.0 22:1.0 23:1.0 24:1.0 29:1.0 35:1.0 36:1.0 3|$37$0:1.0 12:1.0 27:1.0 31:1.0 33:1.0 4|$37$0:1.0 1:1.0 2:1.0 7:1.0 9:1.0 13:1.0 14:1.0 17:1.0 18:1.0 21:1.0 30:1.0
0x03 分词 Segment
中文分词(Chinese Word Segmentation) 指的是将一个汉字序列切分成一个一个单独的词。分词就是将连续的字序列按照一定的规范重新组合成词序列的过程。
示例代码中,分词部分如下:
SegmentBatchOp segment = new SegmentBatchOp() .setSelectedCol("text") .linkFrom(batchData);
分词主要是如下两个类,其作用就是把中文文档分割成单词。
public final class SegmentBatchOp extends MapBatchOp <SegmentBatchOp> implements SegmentParams <SegmentBatchOp> { public SegmentBatchOp(Params params) { super(SegmentMapper::new, params); } } public class SegmentMapper extends SISOMapper { private JiebaSegmenter segmentor; }
3.1 结巴分词
有经验的同学看到这里就会露出微笑:结巴分词。
jieba分词是国内使用人数最多的中文分词工具https://github.com/fxsjy/jieba。jieba分词支持四种分词模式:
- 精确模式,试图将句子最精确地切开,适合文本分析;
- 全模式,把句子中所有的可以成词的词语都扫描出来, 速度非常快,但是不能解决歧义;
- 搜索引擎模式,在精确模式的基础上,对长词再次切分,提高召回率,适合用于搜索引擎分词。
- paddle模式,利用PaddlePaddle深度学习框架,训练序列标注(双向GRU)网络模型实现分词。
Alink使用了 com.alibaba.alink.operator.common.nlp.jiebasegment.viterbi.FinalSeg;
来 完成分词。具体是在https://github.com/huaban/jieba-analysis的基础上稍微做了调整。
public class JiebaSegmenter implements Serializable { private static FinalSeg finalSeg = FinalSeg.getInstance(); private WordDictionary wordDict; ...... private Map<Integer, List<Integer>> createDAG(String sentence) }
从Alink代码中看,实现了索引分词和查询分词两种模式,应该是有分词粒度粗细之分。
createDAG函数的作用是 :在处理句子过程中,基于前缀词典实现高效的词图扫描,生成句子中汉字所有可能成词情况所构成的有向无环图 (DAG)。
结巴分词对于未登录词,采用了基于汉字成词能力的 HMM 模型,使用了 Viterbi 算法。
3.2 分词过程
分词过程主要是在SegmentMapper.mapColumn函数中完成的,当输入是 "二手旧书:医学电磁成像",结巴分词将这个句子分成了六个单词。具体参见如下:
input = "二手旧书:医学电磁成像" tokens = {ArrayList@9619} size = 6 0 = {SegToken@9630} "[二手, 0, 2]" 1 = {SegToken@9631} "[旧书, 2, 4]" 2 = {SegToken@9632} "[:, 4, 5]" 3 = {SegToken@9633} "[医学, 5, 7]" 4 = {SegToken@9634} "[电磁, 7, 9]" 5 = {SegToken@9635} "[成像, 9, 11]" mapColumn:44, SegmentMapper (com.alibaba.alink.operator.common.nlp) apply:-1, 35206803 (com.alibaba.alink.common.mapper.SISOMapper$$Lambda$646) handleMap:75, SISOColsHelper (com.alibaba.alink.common.mapper) map:52, SISOMapper (com.alibaba.alink.common.mapper) map:21, MapperAdapter (com.alibaba.alink.common.mapper) map:11, MapperAdapter (com.alibaba.alink.common.mapper) collect:79, ChainedMapDriver (org.apache.flink.runtime.operators.chaining) collect:35, CountingCollector (org.apache.flink.runtime.operators.util.metrics) invoke:196, DataSourceTask (org.apache.flink.runtime.operators)
0x04 训练
训练是在DocCountVectorizerTrainBatchOp类完成的,其通过linkFrom完成了模型的构建。其实计算TF IDF相对 简单,复杂之处在于之后的大规模排序。
public DocCountVectorizerTrainBatchOp linkFrom(BatchOperator<?>... inputs) { BatchOperator<?> in = checkAndGetFirst(inputs); DataSet<DocCountVectorizerModelData> resDocCountModel = generateDocCountModel(getParams(), in); DataSet<Row> res = resDocCountModel.mapPartition(new MapPartitionFunction<DocCountVectorizerModelData, Row>() { @Override public void mapPartition(Iterable<DocCountVectorizerModelData> modelDataList, Collector<Row> collector) { new DocCountVectorizerModelDataConverter().save(modelDataList.iterator().next(), collector); } }); this.setOutput(res, new DocCountVectorizerModelDataConverter().getModelSchema()); return this; }
4.1 计算IDF
计算 IDF 的工作是在generateDocCountModel完成的,具体步骤如下:
第一步 通过DocWordSplitCount和UDTF的混合使用得到了 文档中的单词数目docWordCnt 。
BatchOperator<?> docWordCnt = in.udtf( params.get(SELECTED_COL), new String[] {WORD_COL_NAME, DOC_WORD_COUNT_COL_NAME}, new DocWordSplitCount(NLPConstant.WORD_DELIMITER), new String[] {});
DocWordSplitCount.eval
的输入是已经分词的句子,然后按照空格分词,按照单词计数。其结果是:
map = {HashMap@9816} size = 6 "医学" -> {Long@9833} 1 "电磁" -> {Long@9833} 1 ":" -> {Long@9833} 1 "成像" -> {Long@9833} 1 "旧书" -> {Long@9833} 1 "二手" -> {Long@9833} 1
第二步 得到了 文档数目docCnt
BatchOperator docCnt = in.select("COUNT(1) AS " + DOC_COUNT_COL_NAME);
这个数目会广播出去 .withBroadcastSet(docCnt.getDataSet(), "docCnt");,
后面的CalcIdf会继续使用,进行 行数统计。
第三步 会通过CalcIdf 计算出每一个单词的DF和IDF 。
open时候会获取docCnt。然后reduce会计算IDF,具体计算如下:
double idf = Math.log((1.0 + docCnt) / (1.0 + df)); collector.collect(Row.of(featureName, -wordCount, idf));
具体得到如下
df = 1.0 wordCount = 1.0 featureName = "中国" idf = 1.0986122886681098 docCnt = 5
这里一个重点是: 返回值中,是 -wordCount,因为单词越多权重越小,为了比较所以取负 。
4.2 排序
得到所有单词的IDF之后,就得到了一个IDF字典, 这时候需要对字典按照权重进行排序 。排序具体分为两步。
4.2.1 SortUtils.pSort
第一步是SortUtils.pSort,大规模并行抽样排序。
Tuple2<DataSet<Tuple2<Integer, Row>>, DataSet<Tuple2<Integer, Long>>> partitioned = SortUtils.pSort(sortInput, 1);
这步非常复杂,Alink参考了论文,如果有兴趣的兄弟可以深入了解下。
* reference: Yang, X. (2014). Chong gou da shu ju tong ji (1st ed., pp. 25-29). * Note: This algorithm is improved on the base of the parallel sorting by regular sampling(PSRS).
pSort返回值是:
* @return f0: dataset which is indexed by partition id, f1: dataset which has partition id and count.
pSort中又分如下几步
采样SampleSplitPoint
SortUtils.SampleSplitPoint.mapPartition这里完成了采样。
DataSet <Tuple2 <Object, Integer>> splitPoints = input .mapPartition(new SampleSplitPoint(index)) .reduceGroup(new SplitPointReducer());
这里的输入row就是上文IDF的返回数值。
用allValues记录了本task目前处理的句子有多少个单词。
用splitPoints做了采样。如何选择呢,通过genSampleIndex函数。
public static Long genSampleIndex(Long splitPointIdx, Long count, Long splitPointSize) { splitPointIdx++; splitPointSize++; Long div = count / splitPointSize; Long mod = count % splitPointSize; return div * splitPointIdx + ((mod > splitPointIdx) ? splitPointIdx : mod) - 1; }
后续操作也使用同样的genSampleIndex函数来做选择,这样保证在操作所有序列上可以选取同样的采样点。
allValues = {ArrayList@10264} size = 8 //本task有多少单词 0 = {Double@10266} -2.0 1 = {Double@10271} -1.0 2 = {Double@10272} -1.0 3 = {Double@10273} -1.0 4 = {Double@10274} -1.0 5 = {Double@10275} -1.0 6 = {Double@10276} -1.0 7 = {Double@10277} -1.0 splitPoints = {ArrayList@10265} size = 7 //采样了7个 0 = {Double@10266} -2.0 1 = {Double@10271} -1.0 2 = {Double@10272} -1.0 3 = {Double@10273} -1.0 4 = {Double@10274} -1.0 5 = {Double@10275} -1.0 6 = {Double@10276} -1.0
最后返回采样数据,返回时候附带当前taskID new Tuple2 <Object, Integer>(obj,taskId)
。
这里有一个trick点
for (Object obj : splitPoints) { Tuple2 <Object, Integer> cur = new Tuple2 <Object, Integer>( obj, taskId); //这里返回的是类似 (-5.0,2) :其中2就是task id,-5.0是-wordcount。 out.collect(cur); } out.collect(new Tuple2( getRuntimeContext().getNumberOfParallelSubtasks(), -taskId - 1));//这里返回的是一个特殊元素,类似(4,-2) :其中4是本应用中并行task数目,-2是当前-taskId - 1。这个task数目后续就会用到。
具体数据参见如下:
row = {Row@10211} "中国,-1.0,1.0986122886681098" fields = {Object[3]@10214} cur = {Tuple2@10286} "(-5.0,2)" // 返回采样数据,返回时候附带当前taskID f0 = {Double@10285} -5.0 // -wordcount。 f1 = {Integer@10300} 2 // 当前taskID
归并 SplitPointReducer
归并所有task生成的sample。然后再次sample,把sample数据组成一个数据块,这个数据块选择的原则是:每个task都尽量选择若干sample 。
这里其实是有一个转换,就是从正常单词的抽样 转换到 某一类单词的抽样,这某一类的意思举例是:出现次数为一,或者出现次数为五 这种单词。
这里all是所有采样数据,其中一个元素内容举例 (-5.0,2) :其中2就是task id,-5.0是-wordcount。
这里用 Collections.sort(all, new PairComparator());
来对所有采样数据做排序。排序基准是首先对 -wordcount,然后对task ID。
SplitPointReducer的返回采样数值就作为广播变量存储起来: .withBroadcastSet(splitPoints, "splitPoints");
这里的trick点是:
for (Tuple2 <Object, Integer> value : values) { if (value.f1 < 0) { instanceCount = (int) value.f0; // 特殊数据,类似(4,-2) :其中4是本应用中task数目,这个就是后续选择哪些taskid的基准 continue; } all.add(new Tuple2 <>(value.f0, value.f1)); // (-5.0,2) 正常数据 }
选择sample index splitPoints.add(allValues.get(index));
也使用了同样的genSampleIndex。
计算中具体数据如下:
for (int i = 0; i < splitPointSize; ++i) { int index = genSampleIndex( Long.valueOf(i), Long.valueOf(count), Long.valueOf(splitPointSize)) .intValue(); spliters.add(all.get(index)); } for (Tuple2 <Object, Integer> spliter : spliters) { out.collect(spliter); } count = 33 all = {ArrayList@10245} size = 33 // 所有采样数据, 0 = {Tuple2@10256} "(-5.0,2)"// 2就是task id,-5.0是-wordcount。 1 = {Tuple2@10285} "(-2.0,0)" ...... 6 = {Tuple2@10239} "(-1.0,0)" 7 = {Tuple2@10240} "(-1.0,0)" 8 = {Tuple2@10241} "(-1.0,0)" 9 = {Tuple2@10242} "(-1.0,0)" 10 = {Tuple2@10243} "(-1.0,0)" 11 = {Tuple2@10244} "(-1.0,1)" ...... 16 = {Tuple2@10278} "(-1.0,1)" ...... 24 = {Tuple2@10279} "(-1.0,2)" ...... 32 = {Tuple2@10313} "(-1.0,3)" // spliters是返回结果,这里分别选取了all中index为8,16,24这个三个record。每个task都选择了一个元素。 spliters = {HashSet@10246} size = 3 0 = {Tuple2@10249} "(-1.0,0)" // task 0 被选择。就是说,这里从task 0中选择了一个count是1的元素,具体选择哪个单词其实不重要,就是为了选择count是1的这种即可。 1 = {Tuple2@10250} "(-1.0,1)" // task 1 被选择。具体同上。 2 = {Tuple2@10251} "(-1.0,2)" // task 2 被选择。具体同上。
SplitData把真实数据IDF插入
use binary search to partition data into sorted subsets。前面函数给出的是词的count,但是没有IDF。这里将用二分法查找 找到IDF,然后把IDF插入到partition data中。
首先要注意一点: splitData的输入就是原始输入input, 和splitPoints的输入是一样 的 。
DataSet <Tuple2 <Integer, Row>> splitData = input .mapPartition(new SplitData(index)) .withBroadcastSet(splitPoints, "splitPoints");
open函数中会取出广播变量 splitPoints。
splitPoints = {ArrayList@10248} size = 3 0 = {Tuple2@10257} "(-1.0,0)" 1 = {Tuple2@10258} "(-1.0,1)" 2 = {Tuple2@10259} "(-1.0,2)"
本函数的输入举例
row = {Row@10232} "入门,-1.0,1.0986122886681098"
会在splitPoints中二分法查找,得到splits中每一个 sample 对应的真实IDF。然后发送出去。
这里需要特殊说明下,这个二分法查找查找的是IDF数值,比如count为1的这种单词对应的IDF数值,可能很多单词都是count为1,所以找到一个这样单词的IDF即可 。
splitPoints = {ArrayList@10223} size = 3 0 = {Tuple2@10229} "(-1.0,0)" 1 = {Tuple2@10230} "(-1.0,1)" 2 = {Tuple2@10231} "(-1.0,2)" curTuple.f0 = {Double@10224} -1.0 int bsIndex = Collections.binarySearch(splitPoints, curTuple, new PairComparator()); int curIndex; if (bsIndex >= 0) { curIndex = bsIndex; } else { curIndex = -bsIndex - 1; } // 假设单词是 "入门",则发送的是 "入门" 这类单词在本partition的index,和 "入门" 的单词本身 // 其实,从调试过程看,是否发送单词信息本身并不重要,因为接下来的那一步操作中,并没有用到单词本身信息 out.collect(new Tuple2 <>(curIndex, row));
reduceGroup计算同类型单词数目
这里是计算在某一partition中,某一种类单词的数目。比如count为1的单词,这种单词总共有多少个 。
后续会把 new Tuple2 <>(id, count)
作为partitionCnt广播变量存起来。
id就是这类单词在这partition中间的index,我们暂时称之为partition index。count就是这类单词在本partition的数目。
// 输入举例 value = {Tuple2@10312} "(0,入门,-1.0,1.0986122886681098)" f0 = {Integer@10313} 0 // 计算数目 for (Tuple2 <Integer, Row> value : values) { id = value.f0; count++; } out.collect(new Tuple2 <>(id, count)); // 输出举例,假如是序号为0的这类单词,其总体数目是12。这个序号0就是这类单词在某一partition中的序号。就是上面的 curIndex。 id = {Integer@10313} 0 count = {Long@10338} 12
4.2.2 localSort
第二步是localSort。Sort a partitioned dataset. 最终排序并且会返回最终数值,比如 (29, "主编,-1.0,1.0986122886681098"), 29就是"主编" 这个单词在 IDF字典中的序号。
DataSet<Tuple2<Long, Row>> ordered = localSort(partitioned.f0, partitioned.f1, 1);
open函数中会获取partitionCnt。然后计算出某一种类单词,其在本partition之前所有partition中,这类单词数目。
public void open(Configuration parameters) throws Exception { List <Tuple2 <Integer, Long>> bc = getRuntimeContext().getBroadcastVariable("partitionCnt"); startIdx = 0L; int taskId = getRuntimeContext().getIndexOfThisSubtask(); for (Tuple2 <Integer, Long> pcnt : bc) { if (pcnt.f0 < taskId) { startIdx += pcnt.f1; } } } bc = {ArrayList@10303} size = 4 0 = {Tuple2@10309} "(0,12)" // 就是task0里面,这种单词有12个 1 = {Tuple2@10310} "(2,9)"// 就是task1里面,这种单词有2个 2 = {Tuple2@10311} "(1,7)"// 就是task2里面,这种单词有1个 3 = {Tuple2@10312} "(3,9)"// 就是task3里面,这种单词有3个 // 如果本task id是4,则其startIdx为30。就是所有partition之中,它前面index所有单词的和。
然后进行排序。 Collections.sort(valuesList, new RowComparator(field));
valuesList = {ArrayList@10405} size = 9 0 = {Row@10421} ":,-1.0,1.0986122886681098" 1 = {Row@10422} "主编,-1.0,1.0986122886681098" 2 = {Row@10423} "国内,-1.0,1.0986122886681098" 3 = {Row@10424} "文献,-1.0,1.0986122886681098" 4 = {Row@10425} "李宜燮,-1.0,1.0986122886681098" 5 = {Row@10426} "糖尿病,-1.0,1.0986122886681098" 6 = {Row@10427} "美国,-1.0,1.0986122886681098" 7 = {Row@10428} "谢恩,-1.0,1.0986122886681098" 8 = {Row@10429} "象棋,-1.0,1.0986122886681098" // 最后返回时候,就是 (29, "主编,-1.0,1.0986122886681098"),29就是“主编”这个单词在最终字典中的序号。 // 这个序号是startIdx + cnt,startIdx是某一种类单词,其在本partition之前所有partition中,这类单词数目。比如在本partition之前,这类单词有28个,则本partition中,从29开始计数。就是最终序列号 for (Row row : valuesList) { out.collect(Tuple2.of(startIdx + cnt, row)); cnt++; // 这里就是在某一类单词中,单调递增,然后赋值一个字典序列而已 } cnt = 1 row = {Row@10336} "主编,-1.0,1.0986122886681098" fields = {Object[3]@10339} startIdx = 28
4.3 过滤
最后还要进行过滤,如果文字个数超出了字典大小,就抛弃多余文字。
ordered.filter(new FilterFunction<Tuple2<Long, Row>>() { @Override public boolean filter(Tuple2<Long, Row> value) { return value.f0 < vocabSize; } })
0x05 生成模型
具体生成模型代码如下。
DataSet<DocCountVectorizerModelData> resDocCountModel = ordered.filter(new FilterFunction<Tuple2<Long, Row>>() { @Override public boolean filter(Tuple2<Long, Row> value) { return value.f0 < vocabSize; } }).mapPartition(new BuildDocCountModel(params)).setParallelism(1); return resDocCountModel;
其中关键类是 DocCountVectorizerModelData 和 BuildDocCountModel。
5.1 DocCountVectorizerModelData
这是向量信息。
/** * Save the data for DocHashIDFVectorizer. * * Save a HashMap: index(MurMurHash3 value of the word), value(Inverse document frequency of the word). */ public class DocCountVectorizerModelData { public List<String> list; public String featureType; public double minTF; }
5.2 BuildDocCountModel
最终生成的模型信息如下,这个也就是之前样例代码给出的输出。
modelData = {DocCountVectorizerModelData@10411} list = {ArrayList@10409} size = 37 0 = "{"f0":"9787310003969","f1":1.0986122886681098,"f2":19}" 1 = "{"f0":"下册","f1":1.0986122886681098,"f2":20}" 2 = "{"f0":"全","f1":1.0986122886681098,"f2":21}" 3 = "{"f0":"华龄","f1":1.0986122886681098,"f2":22}" 4 = "{"f0":"图解","f1":1.0986122886681098,"f2":23}" 5 = "{"f0":"思","f1":1.0986122886681098,"f2":24}" 6 = "{"f0":"成像","f1":1.0986122886681098,"f2":25}" 7 = "{"f0":"旧书","f1":1.0986122886681098,"f2":26}" 8 = "{"f0":"索引","f1":1.0986122886681098,"f2":27}" 9 = "{"f0":":","f1":1.0986122886681098,"f2":28}" 10 = "{"f0":"主编","f1":1.0986122886681098,"f2":29}" 11 = "{"f0":"国内","f1":1.0986122886681098,"f2":30}" 12 = "{"f0":"文献","f1":1.0986122886681098,"f2":31}" 13 = "{"f0":"李宜燮","f1":1.0986122886681098,"f2":32}" 14 = "{"f0":"糖尿病","f1":1.0986122886681098,"f2":33}" 15 = "{"f0":"美国","f1":1.0986122886681098,"f2":34}" 16 = "{"f0":"谢恩","f1":1.0986122886681098,"f2":35}" 17 = "{"f0":"象棋","f1":1.0986122886681098,"f2":36}" 18 = "{"f0":"二手","f1":0.0,"f2":0}" 19 = "{"f0":")","f1":0.6931471805599453,"f2":1}" 20 = "{"f0":"/","f1":1.0986122886681098,"f2":2}" 21 = "{"f0":"出版社","f1":0.6931471805599453,"f2":3}" 22 = "{"f0":"(","f1":0.6931471805599453,"f2":4}" 23 = "{"f0":"入门","f1":1.0986122886681098,"f2":5}" 24 = "{"f0":"医学","f1":1.0986122886681098,"f2":6}" 25 = "{"f0":"文集","f1":1.0986122886681098,"f2":7}" 26 = "{"f0":"正版","f1":1.0986122886681098,"f2":8}" 27 = "{"f0":"版","f1":1.0986122886681098,"f2":9}" 28 = "{"f0":"电磁","f1":1.0986122886681098,"f2":10}" 29 = "{"f0":"选读","f1":1.0986122886681098,"f2":11}" 30 = "{"f0":"中国","f1":1.0986122886681098,"f2":12}" 31 = "{"f0":"书","f1":1.0986122886681098,"f2":13}" 32 = "{"f0":"十二册","f1":1.0986122886681098,"f2":14}" 33 = "{"f0":"南开大学","f1":1.0986122886681098,"f2":15}" 34 = "{"f0":"文学","f1":1.0986122886681098,"f2":16}" 35 = "{"f0":"郁达夫","f1":1.0986122886681098,"f2":17}" 36 = "{"f0":"馆藏","f1":1.0986122886681098,"f2":18}" featureType = "WORD_COUNT" minTF = 1.0
0x06 预测
预测业务逻辑是DocCountVectorizerModelMapper
首先我们可以看到 FeatureType,这个可以用来配置输出哪种信息。比如可以输出以下若干种:
public enum FeatureType implements Serializable { /** * IDF type, the output value is inverse document frequency. */ IDF( (idf, termFrequency, tokenRatio) -> idf ), /** * WORD_COUNT type, the output value is the word count. */ WORD_COUNT( (idf, termFrequency, tokenRatio) -> termFrequency ), /** * TF_IDF type, the output value is term frequency * inverse document frequency. */ TF_IDF( (idf, termFrequency, tokenRatio) -> idf * termFrequency * tokenRatio ), /** * BINARY type, the output value is 1.0. */ BINARY( (idf, termFrequency, tokenRatio) -> 1.0 ), /** * TF type, the output value is term frequency. */ TF( (idf, termFrequency, tokenRatio) -> termFrequency * tokenRatio ); }
其次,在open函数中,会加载模型,比如:
wordIdWeight = {HashMap@10838} size = 37 "医学" -> {Tuple2@10954} "(6,1.0986122886681098)" "选读" -> {Tuple2@10956} "(11,1.0986122886681098)" "十二册" -> {Tuple2@10958} "(14,1.0986122886681098)" ... "华龄" -> {Tuple2@11022} "(22,1.0986122886681098)" "索引" -> {Tuple2@11024} "(27,1.0986122886681098)" featureType = {DocCountVectorizerModelMapper$FeatureType@10834} "WORD_COUNT"
最后,预测时候调用predictSparseVector函数,会针对输入 二手 旧书 : 医学 电磁 成像
来进行匹配。生成稀疏向量SparseVector。
0|$37$0:1.0 6:1.0 10:1.0 25:1.0 26:1.0 28:1.0
以上表示那几个单词 分别对应0 6 10 25 26 28 这几个字典中对应序号的单词,其在本句对应的出现数目都是一个。
0x07 参考
Recommend
About Joyk
Aggregate valuable and interesting links.
Joyk means Joy of geeK