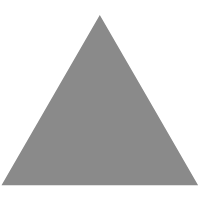
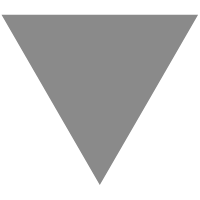
ClickHouse and MySQL – Better Together
source link: https://www.percona.com/blog/2020/02/03/clickhouse-and-mysql-better-together/
Go to the source link to view the article. You can view the picture content, updated content and better typesetting reading experience. If the link is broken, please click the button below to view the snapshot at that time.
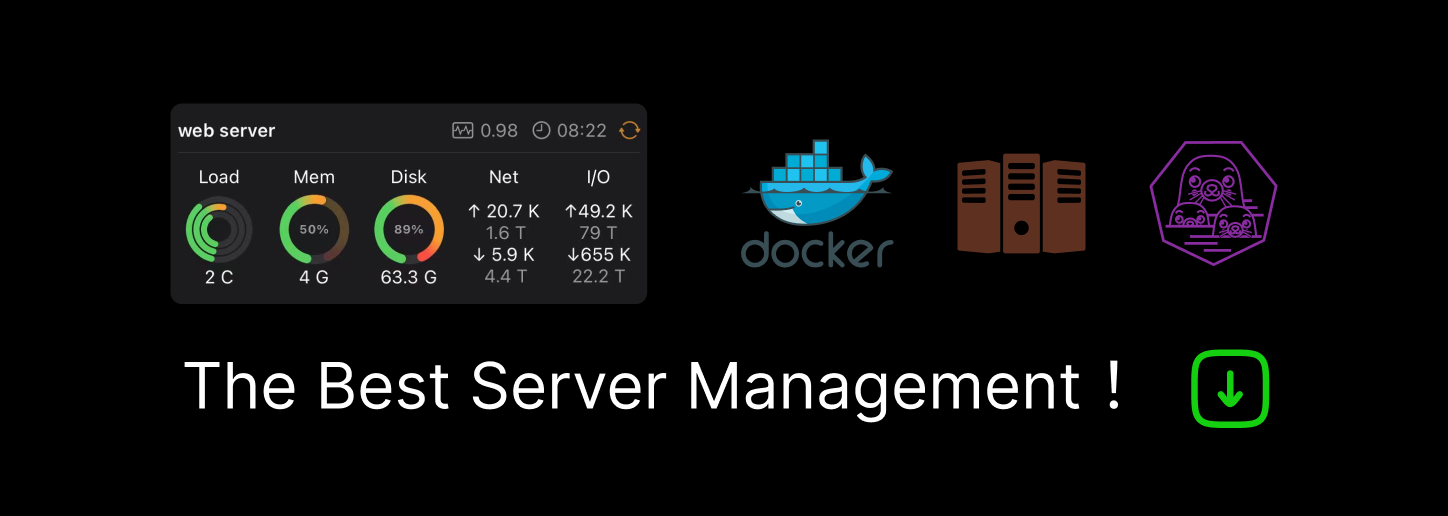
It’s been a while since I wrote about ClickHouse, there are a lot of new features that are worth mentioning, and for this, I recommend to follow the Altinity Blog but today I want to look at the improved integration of ClickHouse and MySQL.
There are two features:
- Using MySQL protocol and MySQL client to connect to ClickHouse
- Use MySQL tables to select and join with ClickHouse tables
Using MySQL Protocol
By enabling MySQL protocol in ClickHouse server, you will allow for the MySQL command line tool or applications that typically connect to MySQL to connect to ClickHouse and execute queries.
With this, keep in mind:
- It does not automatically allow you to use MySQL SQL syntax, you still need to write queries in ClickHouse-dialect.
- The same issue with datatypes. ClickHouse uses Int32/Int64 instead of INT/BIGINT and String instead of VARCHAR, so most likely management tools like MySQL Workbench won’t work.
How do you enable MySQL protocol? Actually it is easy, you add to config:
/etc/clickhouse-server/config.xml: ... <mysql_port>9001</mysql_port> ...
And after a ClickHouse restart you can connect with mysql command line:
mysql -h127.0.0.1 -P9001 -udefault -padmin
And after that, we can work as we usually work from MySQL command line. Some examples with ontime databases :
mysql> use default; Reading table information for completion of table and column names You can turn off this feature to get a quicker startup with -A Database changed mysql> show tables; +--------+ | name | +--------+ | ontime | +--------+ 1 row in set (0.00 sec) Read 1 rows, 31.00 B in 0.000 sec., 2038 rows/sec., 61.71 KiB/sec.
Select count from ontime tables (I loaded data only for the Jan-2017 to Nov-2019 timeframe)
select count(*) from ontime; +----------+ | count() | +----------+ | 19684341 | +----------+ 1 row in set (0.00 sec) Read 1 rows, 4.01 KiB in 0.000 sec., 2150 rows/sec., 8.42 MiB/sec.
And now we can run some analytical queries, e.g., what ten airports had the most departures for the given time frame:
mysql> SELECT Origin,count(*) cnt FROM ontime GROUP BY Origin ORDER BY cnt DESC LIMIT 10; +--------+---------+ | Origin | cnt | +--------+---------+ | ATL | 1117414 | | ORD | 911572 | | DFW | 739334 | | DEN | 689847 | | LAX | 637070 | | CLT | 562160 | | SFO | 507377 | | PHX | 488398 | | IAH | 467543 | | LAS | 463627 | +--------+---------+ 10 rows in set (0.03 sec) Read 19684341 rows, 93.86 MiB in 0.027 sec., 726992466 rows/sec., 3.39 GiB/sec.
And what the most popular routes are:
mysql> SELECT Origin,Dest,count(*) cnt FROM ontime GROUP BY Origin,Dest ORDER BY cnt DESC LIMIT 10; +--------+-------+-------+ | Origin | Dest | cnt | +--------+-------+-------+ | SFO | LAX | 44419 | | LAX | SFO | 44204 | | LGA | ORD | 39276 | | ORD | LGA | 39141 | | LAX | JFK | 37309 | | JFK | LAX | 37256 | | LAX | LAS | 33780 | | LAS | LAX | 33757 | | OGG | HNL | 29619 | | HNL | OGG | 29616 | +--------+-------+-------+ 10 rows in set (0.05 sec) Read 19684341 rows, 187.73 MiB in 0.053 sec., 372125496 rows/sec., 3.47 GiB/sec.
Well, I can do this all day long, but let me just show the last query which highlights the difference in syntax.
Assume we want to get the data from the previous query but per year (ten most popular routes per year). The most elegant solution to get this is to use WINDOW functions, which are supported in MySQL 8 but not yet supported in ClickHouse. However, ClickHouse has a workaround for this one special case. We can get the result we need using the LIMIT BY extension (not to be confused with LIMIT ).
For ClickHouse we write:
mysql> SELECT Year,Origin,Dest,count(*) cnt FROM ontime GROUP BY Year,Origin,Dest ORDER BY Year asc,cnt DESC LIMIT 5 BY Year; +------+--------+-------+-------+ | Year | Origin | Dest | cnt | +------+--------+-------+-------+ | 2017 | SFO | LAX | 15786 | | 2017 | LAX | SFO | 15564 | | 2017 | JFK | LAX | 12758 | | 2017 | LAX | JFK | 12752 | | 2017 | LAX | LAS | 11032 | | 2018 | ORD | LGA | 15281 | | 2018 | LGA | ORD | 15279 | | 2018 | SFO | LAX | 15158 | | 2018 | LAX | SFO | 15143 | | 2018 | LAX | JFK | 12791 | | 2019 | ORD | LGA | 13696 | | 2019 | LGA | ORD | 13691 | | 2019 | LAX | SFO | 13497 | | 2019 | SFO | LAX | 13475 | | 2019 | LAX | JFK | 11766 | +------+--------+-------+-------+ 15 rows in set (0.07 sec) Read 19684341 rows, 225.27 MiB in 0.073 sec., 270137514 rows/sec., 3.02 GiB/sec.
JOIN with MySQL Tables.
The feature to get data from MySQL using dictionaries in ClickHouse was implemented long ago, but it was not convenient, leading to using non-standard SQL extensions. Since then, two new features were implemented in ClickHouse:
- Support of JOIN syntax
- Support of external tables
This allows us to run more familiar queries with the mix of MySQL and ClickHouse tables. Before we jump to an example, let’s review why this is needed.
The typical data analytics design assumes there are big fact tables with references to dimension tables (aka dictionaries if using ClickHouse lexicon). For a detailed example, see Star Schema .
Dimension tables may be changed/updated more frequently, and ClickHouse does not quite like it, as it operates in more append-only like mode. So we may want to store Dimension tables in OLTP databases, like MySQL. This, by the way, also helps with GDPR policies for personalized data removal. In case you store all personal data in a denormalized fact table, it becomes very complicated to remove it. In contrast, if the personal data is stored in a dimension table, it is quite easy to anonymize it.
So let’s go back to our example.
Ontime table (fact tables) has a reference field AirlineID, which is not very helpful, as we want to see the airline name in our report.
The data for the AirlineID – AirlineName lookup (dimension) table, can be found on the BTS website .
It looks like this:
We load this data into a MySQL table:
CREATE TABLE airlines id INT NOT NULL PRIMARY KEY, name VARCHAR(255) ) select count(*) from airlines; +----------+ | count(*) | +----------+ | 1671 | +----------+
Now, how do we connect this table to ClickHouse? For this, in ClickHouse we create a table with “MySQL table engine”:
Clickhouse -> (and we can connect to it with mysql client tool, see part one).
CREATE TABLE airlinesclick ( `id` Int32, `name` String ) ENGINE = MySQL('127.0.0.1:3306', 'click', airlines, 'sbtest', 'sbtest');
Now we can execute a query which joins ClickHouse fact table, ontime, and MySQL dimension table airlines, to report the top ten airlines with the most departures:
SELECT name,count(*) cnt FROM ontime JOIN airlinesclick ON ontime.AirlineID=airlinesclick.id GROUP BY name ORDER BY cnt DESC LIMIT 10; +-----------------------------+---------+ | name | cnt | +-----------------------------+---------+ | Southwest Airlines Co.: WN | 3931500 | | Delta Air Lines Inc.: DL | 2783305 | | American Airlines Inc.: AA | 2680537 | | SkyWest Airlines Inc.: OO | 2245105 | | United Air Lines Inc.: UA | 1780665 | | JetBlue Airways: B6 | 875858 | | Alaska Airlines Inc.: AS | 673652 | | ExpressJet Airlines LLC: EV | 665911 | | Republic Airline: YX | 616362 | | Envoy Air: MQ | 595908 | +-----------------------------+---------+ 10 rows in set (0.05 sec) Read 19686012 rows, 75.15 MiB in 0.050 sec., 394346280 rows/sec., 1.47 GiB/sec.
To summarize what just happened: We executed a query in ClickHouse using normal human-readable JOIN syntax (compare to queries in ClickHouse in a General Analytical Workload (Based on a Star Schema Benchmark) and join tables stored in different servers: MySQL and ClickHouse.
If I am to illustrate:
Recommend
-
12
ClickHouse和他的朋友们(9)MySQL实时复制与实现 - 老叶茶馆 - OSCHINA - 中文开源技术交流社区原文出处:https://bohutang.me/2020/07/26/clickhouse-and-friends-mysql-replication/
-
7
Percona and PostgreSQL: Better Together Back to the Blog The future of technology adoption belon...
-
7
Load Balancing ...
-
6
SAST and SCA: Better together with Snyk Daniel Berman
-
9
目前来说,网上有很多相关的资料证明ClickHouse数据库查询响应速度比MySQL快上一百到几百倍。实际上,ClickHouse和MySQL具有不同的应用场景和局限性,最近在研究这个ClickHouse打算应用于大...
-
11
clickhouse,tidb,mysql 读取速度对比«海底苍鹰(tank)博客 clickhouse,tidb,mysql,这三个数据库都是现在在用的。准备了一些测试数据,测试一下读取数据的速度。 一,硬件说明 1,mysql环境,普通的台机,没...
-
16
2 March 2021 Clickhouse as an alternative to ElasticSearch and MySQL, for log storage and analysis, in 2021 In 2018, I've written an
-
8
Using ClickHouse as an Analytic Extension for MySQL Back to the Blog
-
5
使用 ClickHouse 作为 MySQL 的数据分析扩展原文链接:https://www.percona.com/blog/using-clickhouse-as-a...
-
3
继续书接上文 Docker Compose V2 安装常用数据库MySQL+Mongo,部署安装好之后我本来是找了一个web端的在线连接数据库的工具,但是使...
About Joyk
Aggregate valuable and interesting links.
Joyk means Joy of geeK