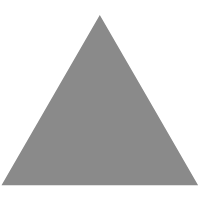
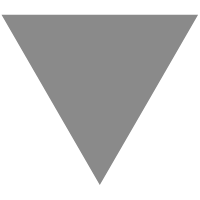
Automatic Image Captioning with CNN & RNN
source link: https://mc.ai/automatic-image-captioning-with-cnn-rnn/
Go to the source link to view the article. You can view the picture content, updated content and better typesetting reading experience. If the link is broken, please click the button below to view the snapshot at that time.
Loading Annotations/Tokenizing Captions
The RNN component of the captioning network is trained on the captions in the COCO dataset. We’re aiming to train the RNN to predict the next word of a sentence based on previous words. But, how exactly can it train on string data? Neural nets do not do well with strings. They need a well defined numerical alpha to effectively perform back-propagation and learn to produce similar output. So, we have to transform the captions associated with the image into a list of tokenized words. This tokenization turns any string into a list of words.
Working of Tokenization
First, we iterate through all of the training captions and create a dictionary that maps all unique words to a numerical index. So, every word we come across will have a corresponding integer value that can find in this dictionary. The words in this dictionary are referred to as our vocabulary. The vocabulary typically also includes a few special tokens.
Embedding Layer
Theres one more step before these words get sent as input to an RNN and thats the embedding layer, which transforms each word in a caption into a vector of a desired consistent shape.
Words to Vectors
At this point, we know that you cannot directly feed words into an LSTM and expect it to be able to train or produce the correct output. These words first must be turned into a numerical representation so that a network can use normal loss functions and optimizers to calculate how “close” a predicted word and ground truth word (from a known, training caption) are? So, we typically turn a sequence of words into a sequence of numerical values; a vector of numbers where each number maps to a specific word in our vocabulary.
Training the RNN Decoder Model with suitable parameters
The Decoder will be made of LSTM cells which is good for remembering the lengthy sequences of words. Each LSTM cell is expecting to see the same shape of the input vector at each time-step. The very first cell is connected to the output feature vector of the CNN encoder. The input to the RNN for all future time steps will be the individual words of the training caption. So, at the start of training, we have some input from our CNN, and LSTM cell with initial state. Now the RNN has two responsibilities:
- To Remember spatial information from the input feature vector.
- To Predict the next word.
We know that the very first word it produces should always be the <start> token and the next word should be those in the training caption. At every time step, we look at the current caption word as input and combine it with the hidden state of the LSTM cell to produce an output. This output is then passed to the fully connected layer that produces a distribution that represents the most likely next word. We feed the next word in the caption to the network and so on until we reach the <end>token. The hidden state of an LSTM is a function of the input token to the LSTM and the previous state also referred to as the recurrence function. The recurrence function is defined by weights and during the training process, this model uses back-propagation to update these weights until the LSTM cells learn to produce the correct next word in the caption given the current input word. As with most models, you can also take advantage of batching the training data. The model updates its weights after each training batch with the batch size is the number of image caption pairs sent through the network during a single training step. Once the model has trained, it will have learned from many image caption pairs and should be able to generate captions for new image data.
Note: Please do play around with hyperparameters if you don’t get the desired result. I’ve nailed the hyperparameters by setting them to particular value based on instinct in one go. Also, please make sure not to change the values of mean & standard deviation in transforms.Normalize() as those values are default and are considered after rigorous training of ResNet architecture on the ImageNet Dataset.
Do test the model on Test/Validation Data to check for overfitting as the above result is of the training set.
Generate Predictions
A function ( get_prediction
) used to loop over images in the test dataset and print your model’s predicted caption.
Output:
Call the ( get_prediction
) function every time you want the result.
When the model performed better:
When the model didn’t perform well:
Recommend
About Joyk
Aggregate valuable and interesting links.
Joyk means Joy of geeK