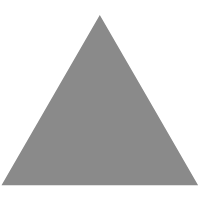
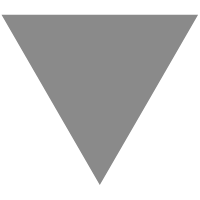
What Makes AI Intelligent?
source link: https://towardsdatascience.com/what-makes-ai-intelligent-dc4df03dfc30?gi=aa71e73bfe9
Go to the source link to view the article. You can view the picture content, updated content and better typesetting reading experience. If the link is broken, please click the button below to view the snapshot at that time.
A Guide to Artificial Networks, Deep Learning, AI and How They are Enhancing Solar Asset Management.
Dec 31 ·9min read
Artificial intelligence is at the peak of its hype curve, and its applications in the solar energy sector are amid a surge in popularity. Once upon a time confined solely to the domains of science fiction, this technology is transforming the energy landscape, altering how solar assets are managed, operated, and maintained.
Year after year, the cumulative global PV capacity is increasing by gigawatts, which are highly dependent on operating conditions that are inherently variable and hard to predict. Also, further consolidation of these solar assets is leading to these portfolios growing not only in size but also in dispersity.
These factors have made managing solar assets considerably more
challenging. Inarguably, there are substantial financial and efficiency gains to be made in using AI-driven solutions.
Moreover, as AI technology matures, it is incumbent on every solar asset owner to answer the question of how this technology will disrupt the industry on a macroscale and how it can be leveraged to benefit their portfolio at the micro-level.
This piece examines the areas, which could be aided by AI, utilizing case studies to capture the current potential of artificial intelligence to drive efficiency and Return On Investment (ROI) in solar portfolios.
There is a vast amount of noise around AI in the solar space, and, in this piece, I’ll attempt to shine a light on the facets of asset management that can be automated to help you navigate through
that noise.
Additionally, different Machine Learning (ML) techniques, which have been or can be deployed in the overall operations and management of solar assets, are highlighted to provide a working understanding of this sophisticated technology.
What Makes A.I Intelligent?
Despite the numerous and somewhat straightforward definitions available on the internet, people still often grapple with distinguishing between ordinary software and AI — and reasonably so. No clear lines seem to exist between these two realms.
However, for this piece, I’ll focus on the intelligence aspect of AI, as that is where the key differentiator of AI and the run-of-the-mill software lies. The essence of intelligence is learning. In the same manner that humans learn how to speak or identify patterns or otherwise, machines can also learn to carry out tasks, necessitating some level of intelligence.
Where AI shines is in its tremendous ability to perform under uncertain circumstances, which are the cornerstone of most prediction and optimization challenges the solar industry is facing. These challenges primarily depend on a myriad of non-human-controllable elements, for instance, environmental parameters.
This brings me to the next point of this section:
How is this Intelligence Created?
For non-specialists, this question can be daunting to tackle, which in return makes it hard to understand what AI can be used for truly. In a nutshell, AI entails programs with the ability to mimic human learning and reasoning skills.
Diving deeper, AI utilizes algorithms that can learn without explicit programming, which form what is known as Machine Learning (ML). At its crux, AI has a new type of algorithms ushering in a new wave of this technology, called Artificial Neural Networks(ANN), to replicate human brain cells on a computer.
The study of these types of algorithms has spurred another branch, called Deep Learning. The rather lengthy history of AI raises the question of ‘How come only now we can truly reap the benefits of AI?’ In brief, the three primary forces driving AI leaps are:
Big Data, Shrinking cost of computing power, and Improved algorithms.
ANN & Other AI Techniques Applied in Solar
The scope of techniques that comprise AI keeps expanding as artificial technologies continue to advance. For this piece, I’ll zoom in on some of the fundamental AI and advanced analytics techniques being applied in the solar energy industry to hopefully demystify this sophisticated technology.
Adaptive Network-based Fuzzy Inference
System and Data mining are deployed to predict solar radiation and temperature and to improve solar cell performance. Loosely speaking, AI techniques should be able to have intelligence comparable to humans or even superhumans.
Artificial Neural Networks form the foundation for Deep Learning, as mentioned earlier, and are the most common example of artificially intelligent systems.
Deep Learning is capable of carrying out astonishing tasks. It is, for example, precisely what allows your phone to recognize your voice and distinguish it from someone else’s.
Artificial Neural Networks (ANN)
Simply put, ANNs are processing devices that are designed to model the neural structure of a brain. As humans, we use our nervous system to process information gathered through our environment to adapt and make decisions.
This nervous system can be replicated, which can make it possible to recreate similar sets of behavior in artificial systems. The most striking difference between ANN and our neural structure is the number of neurons. The human brain has billions of neurons, whereas ANN may only have hundreds or thousands.
ANN are trained by learning patterns utilizing trial and error, exactly as the human brain does. These networks are generally organized in layers, constituting several interconnected ‘nodes,’ again just like a human neuron. The pattern moves through multiple layers to generate output, and the learning is achieved through the weights (W).
One of the applications of ANN in solar is forecasting, this process, just
like any AI-powered solution, necessitates a training phase. Once ANN is tuned, it can generate predictions of the solar PV system output levels, merely utilizing weather forecast as input.
The results should be continuously evaluated to ensure their accuracy. Solar radiation and other parameters measured on the plant affect how accurate the weather forecasting — and hence the method — is. It is evident that accurate and reliable data is the backbone of this entire model.
The input data is supplied through meteorological service, the position of the sun using time and date, as well as the site geographical coordinates. This kind of predictive analytics is especially valuable in cases where contractual obligations require asset owners to meet production targets or risk steep penalties.
Using AI & ML to Optimize Solar Assets
Moving on to the primary purpose of this piece:
which facets of the asset management equation can be dependably automated?
The answer: Data analytics related to ongoing plant performance and optimization.
As any asset manager overseeing a sizeable solar portfolio will attest, one of the most time consuming and nerve-wracking aspects of the job is ensuring that adequate performance data for each plant gets properly acquired, vetted and analyzed in such a way as to yield timely and actionable intelligence. This
sounds straightforward enough, but, in reality, that is not the case. Especially when some or all aspects of the process remain manual, untrustworthy, or time-consuming (or all of the above).
That is where automated analytics come into the picture — rapidly and efficiently transforming large volumes of standard solar plant data into quantified and prioritized performance improvement actions both at the individual plant level and at the whole portfolio level.
To achieve such an extensive level of analytics sophistication, automation, and usability, service providers are developing advanced toolsets.
Availability and Downtime Analysis
There is a reason that Availability is one of the solar industry’s most important Key Performance Indicators (KPIs): Down inverters can quickly turn into hours or even days of missed production. The asset owner needs to understand the production impact, cause, and responsible party for every outage affecting the plant.
Even without a CMMS tool, smart data engines using monitoring data can provide this understanding efficiently, objectively, and automatically. Solar plants offer a variety of monitoring data: Alarms and status from the inverter, irradiance, and power, plant controller status, and energy meter status, to name a few.
Advanced Analytics leverages these sources of information, learns how to recognize the correct indicator of the root cause of an event, and correctly identifies data outages as either real power outages or data quality issues.
Let’s imagine inverter downtime events at the utility-scale plant in the summer month by initial cause, analyzed by algorithms relying strictly on monitoring data. The most significant amount of energy lost is based on issues related to the inverter’s control system. Still, a sizeable amount of loss can also result from ground faults on the buried DC cables, which had suffered damage during construction.
In previous months, other causes of loss could include snow cover and a curtailment request from the grid. With reliable and automated root cause analysis at their fingertips, asset managers can correctly assign responsibility, and more adequately adjust response plans at the fleet level.
Early Detection of Equipment Reliability Issues
With a robust data processing tool, certain inverter issues that would otherwise go undetected or be discarded as isolated glitches can now be detected as an early indicator of future reliability issues.
ML tools can be trained to detect specific patterns that are known to be indicative of larger reliability issues or catastrophic failures. Advanced Analytics makes possible the treatment of vast amounts of data such that these anomaly signals can be separated from what would otherwise be deemed as noise.
Through the lens of Advanced Analytics, inverters can reveal signals that indicate overheating behavior, which can rapidly affect power electronics components; frequent self-restart behavior, which increases thermal cycling stress; late startup issues, which can hide an irregularity in the inverter manufacturer’s settings.
Aerial Inspection
Having a regular inspection in place is a crucial part of proper (preventive) maintenance to ensure the profitability of solar assets in the long-term.
Aerial inspections help clients to understand better what is happening with their plants as they provide an overview of any existing anomalies and their causes. Naturally, the bigger the plant, the harder it is to spot the problems hindering any performance. In these cases, an inspection can reap the benefits of ML algorithms, which first validate every panel to discover their overall health.
Aerial inspection offers increased accuracy and the ability to inspect any PV plant entirely regularly. This part of the piece focuses on the core pillars allowing for effective drone inspection in particular, and ultimately new ways of asset optimization. According to Sitemark , a global AI-powered aerial data platform, drone inspection constitutes four main pillars:
Data Acquisition:
Overall, four primary factors affect the quality of data
including:
• The size of the PV plant.
• Inconsistency in the standard used by different pilots, flying the mission over multiple days.
• Pilots’ dependability on weather and
irradiance, leading to non-compliant
image acquisition against IEC standards
• Inconsistencies in image resolution due to manual adaptation of drone’s height to hilly terrains.
Data Processing
Once any data is collected, it needs to be processed. Aerial inspection entails
Photogrammetry, which essentially stitches together composite imagery of a site in successive frames. Sitemark has developed a custom-built photogrammetry system that allows for fully automated processing of radiometric thermal data. This methodology aids the generation of large radiometric maps, which not only show where the anomalies are located, but also offer the possibility of comparing and measuring individual cell temperatures.
AI
AI technology makes the complex task of automated locating and categorizing any anomalies possible. However, there are powerful AI-powered technologies available based on the combination of all of the data they have processed up to now, which only improves over time as it is provided with more and more anomalies are detected in sites all across the globe
Platform
After data is gathered and processed, it must be translated into actionable insights to address the faults detected. A consideration at this step is that an actionable report should include different elements for different teams performing the maintenance on the site to better direct them.
Another challenge in visualizing the results and actionable remediation is the lack of organization, caused by the high
number of anomalies. These challenges can be resolved through the Sitemark platform, which employs a region functionality.
This helps to categorize the site into smaller parts on three levels, those of Zones, Inverter, and String, leveraging the power of drones and AI to manage and compare all of the relevant sites from within one web-browser. Comprehensive reports can also be created on the platform to create overviews of types of anomalies, hotspot distribution, as well as severity distribution.
All lessons learned, it is important to remember that the true promise of AI will be fulfilled only when treated as a strategic capability that can mature over time, which requires well-thought planning.
Recommend
About Joyk
Aggregate valuable and interesting links.
Joyk means Joy of geeK