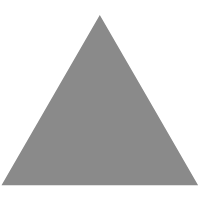
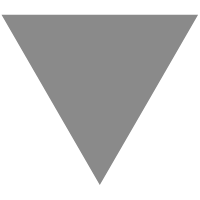
Benchmarking deep learning activation functions on MNIST
source link: https://mc.ai/benchmarking-deep-learning-activation-functions-on-mnist/
Go to the source link to view the article. You can view the picture content, updated content and better typesetting reading experience. If the link is broken, please click the button below to view the snapshot at that time.
Some popular activation functions
ReLU (and softmax)
A rectified linear unit, or ReLU, is a very simple activation function. It returns 0 when the input is smaller than 0, or the value if it’s greater than or equal to 0. In a formula:
Almost every neural networks outputs values between 0 and 1 — the probability of an input belonging to some class. Since ReLUs allow values far greater than 0, the values need to be scaled.
The way this is usually done is by applying the softmax activation function. Mathematically, it’s defined as
with k
as the number of inputs.
This function might seem complex, but the idea is quite simple. Basically, softmax scales the output to range 0 to 1, with all the numbers adding up to 1. This is reasonable because it means the neural network is 100% certain that the input image one of the output categories (note that if there are images in a dataset without a label, they should be labeled as ‘unclassified’ or ‘other’).
Another thing softmax does is scale the numbers based on the input value. The scaled value of the output node with the highest value will be much higher than the node with the second highest output.
ReLU activation functions are a very popular choice among deep learning practitioners because they are very cheap to compute.
Sigmoid
Sigmoid is another one of the classic activation functions. The sigmoid function is 0.5 at the y-axis and has two asymptotes at 0 and 1.
The mathematical formula is
Tanh
Tanh is very similar to sigmoid. The key difference is that tanh has asymptotes at -1 and 1 instead of 0 and 1.
Since the value of tanh can be smaller than 0, it is recommended to use a softmax function in the output layer.
elu
elu stands for exponential linear unit. This unit is similar to both ReLU and tanh. For x<0, it looks like tanh, and for x>1 it’s more similar to ReLUs.
Like tanh, an elu can output values lower than 0, so for tanh, it’s recommended to use softmax in the output layer as well.
softplus
Softplus is similar to elu, but it’s value is always greater than 0.
Recommend
About Joyk
Aggregate valuable and interesting links.
Joyk means Joy of geeK